Abstract
OBJECTIVE. This study investigated whether unexpected length of stay (LOS) could be used as an indicator to identify hospital patients who experienced complications or whose care exhibited low adherence to normative practices. DATA SOURCES AND STUDY SETTING. We analyzed 1,477 cases admitted for one of three medical conditions. All cases were discharged from one of nine participating Department of Veterans Affairs (VA) hospitals from October 1987 through September 1989. Analyses used administrative data and information abstracted through chart reviews that included severity of illness indicators, complications, and explicit process of care criteria reflecting adherence to normative practices. STUDY DESIGN. We developed separate multiple linear regression models for each disease using LOS as the dependent measure and variables that could be assumed present at the time of admission as explanatory variables. Unexpectedly long LOS (i.e., discharges with high residuals) was used to target complications and unexpectedly short LOS was used to target cases whose care might have exhibited low adherence to normative practices. Information gleaned from chart reviews served as the gold standard for determining actual complications and low adherence. PRINCIPAL FINDINGS. Analyses of administrative data showed that unexpectedly long LOS identified complications with sensitivities ranging from 40 through 62 percent across the three conditions. Positive predictive values all were at greater than chance levels (p < .05). This represented substantial improvement over identification of complications using ICD-9-CM codes contained in the administrative database where sensitivities were from 26 through 39 percent. Unexpectedly short LOS identified low provider adherence with sensitivities ranging from 33 through 45 percent with positive predictive values all above chance levels (p < .05). The addition to the LOS models of chart-based severity of illness information helped explain LOS, but failed to facilitate identification of complications or low adherence beyond what was accomplished using administrative data. CONCLUSIONS. Administrative data can be used to target cases when seeking to identify complications or low provider adherence to normative practices. Targeting can be accomplished through the creation of indirect measures based on unexpected LOS. Future efforts should be devoted to validating unexpected LOS as a hospital-level quality indicator. RELEVANCE/IMPACT. Scrutiny of unexpected LOS holds promise for enhancing the usefulness of administrative data as a resource for quality initiatives.
Full text
PDF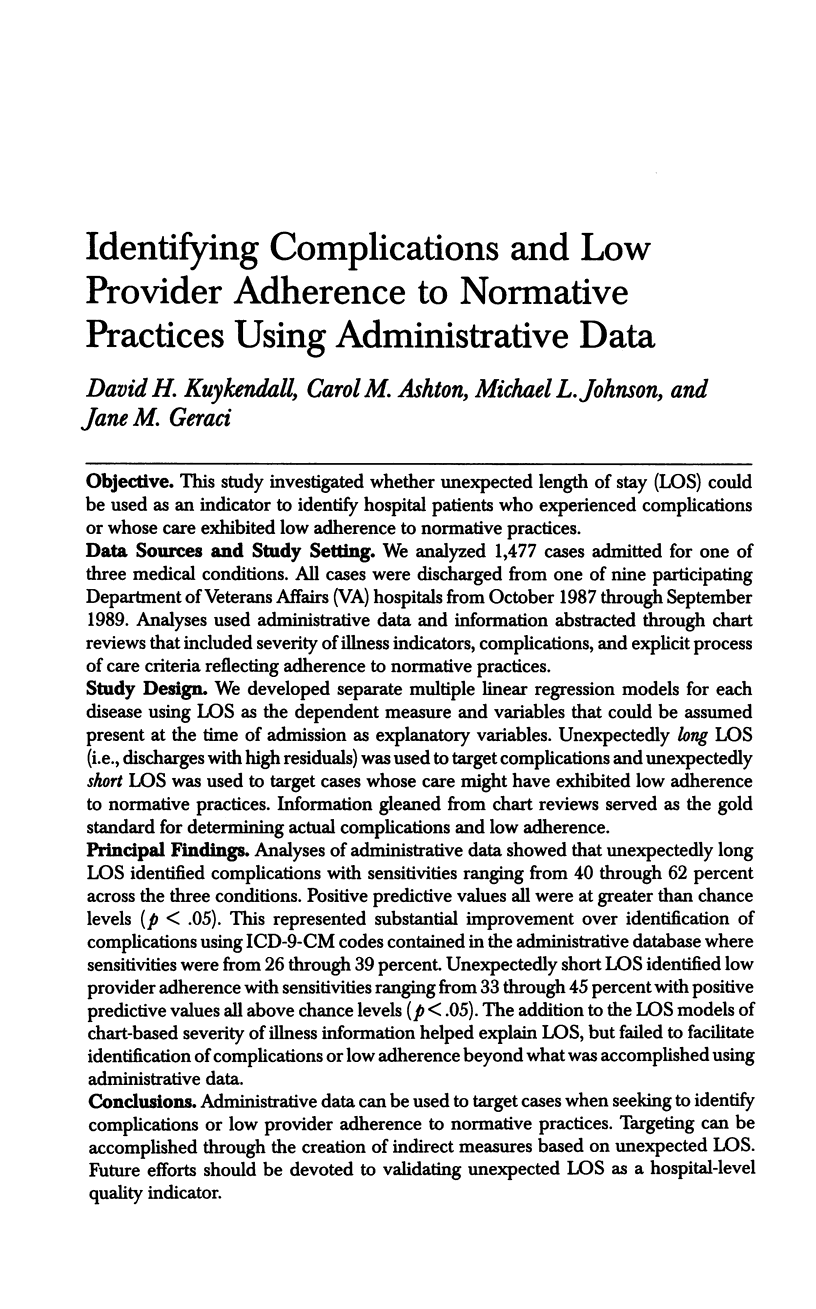
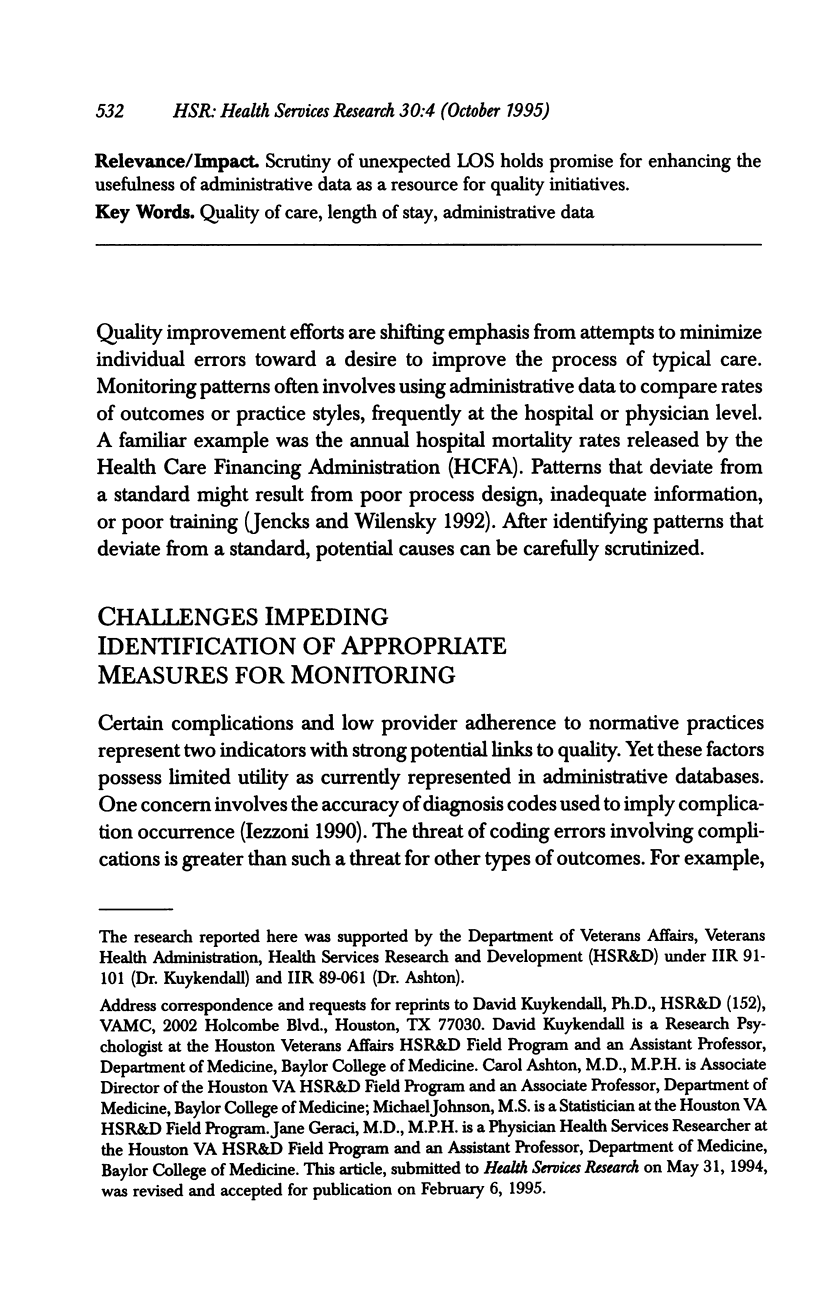
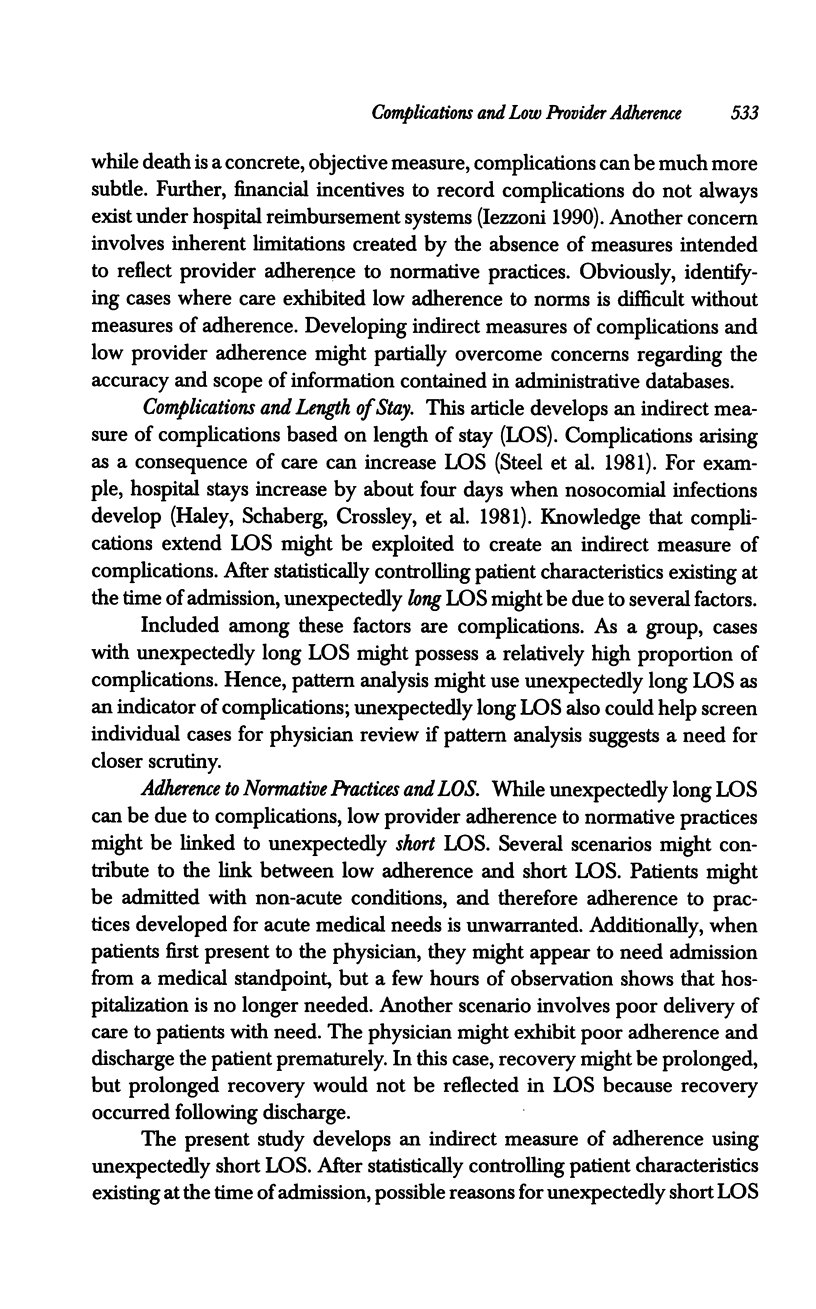
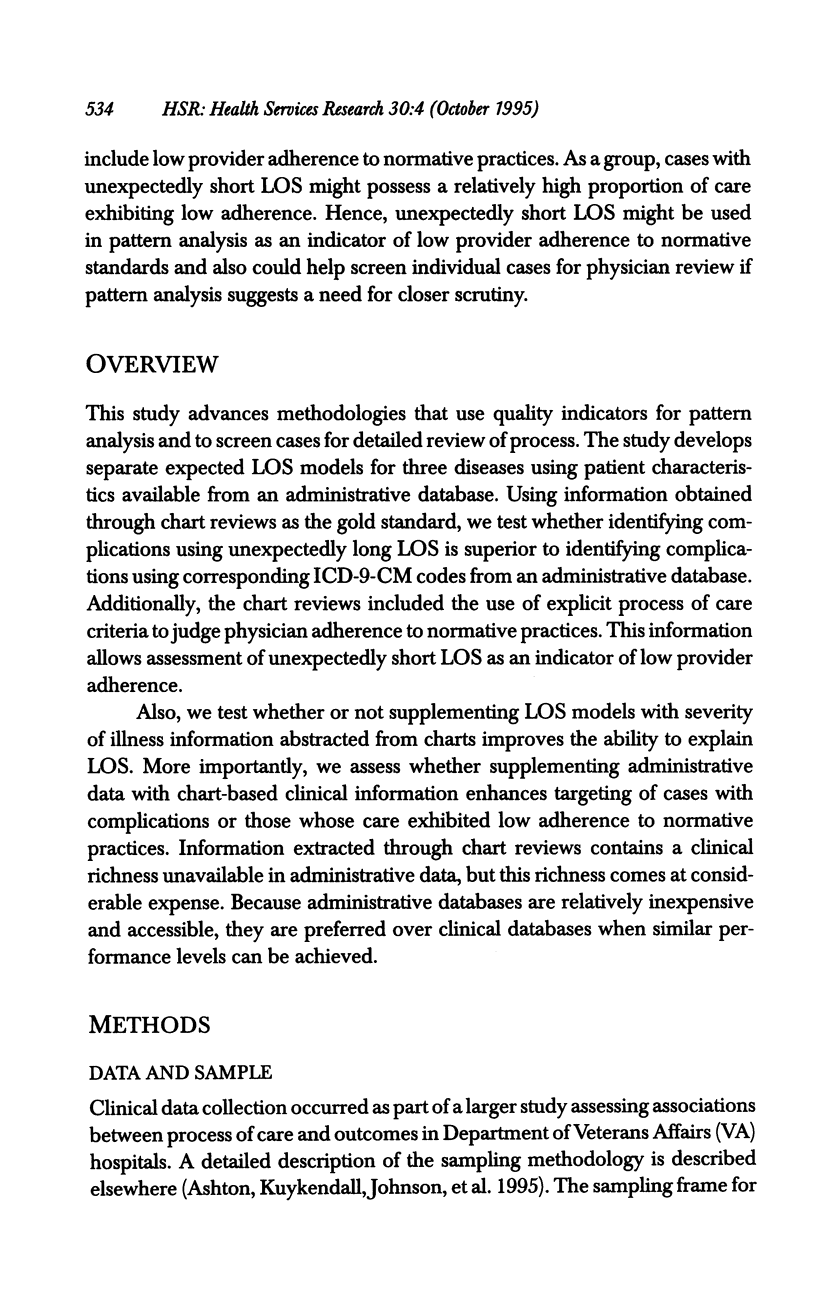
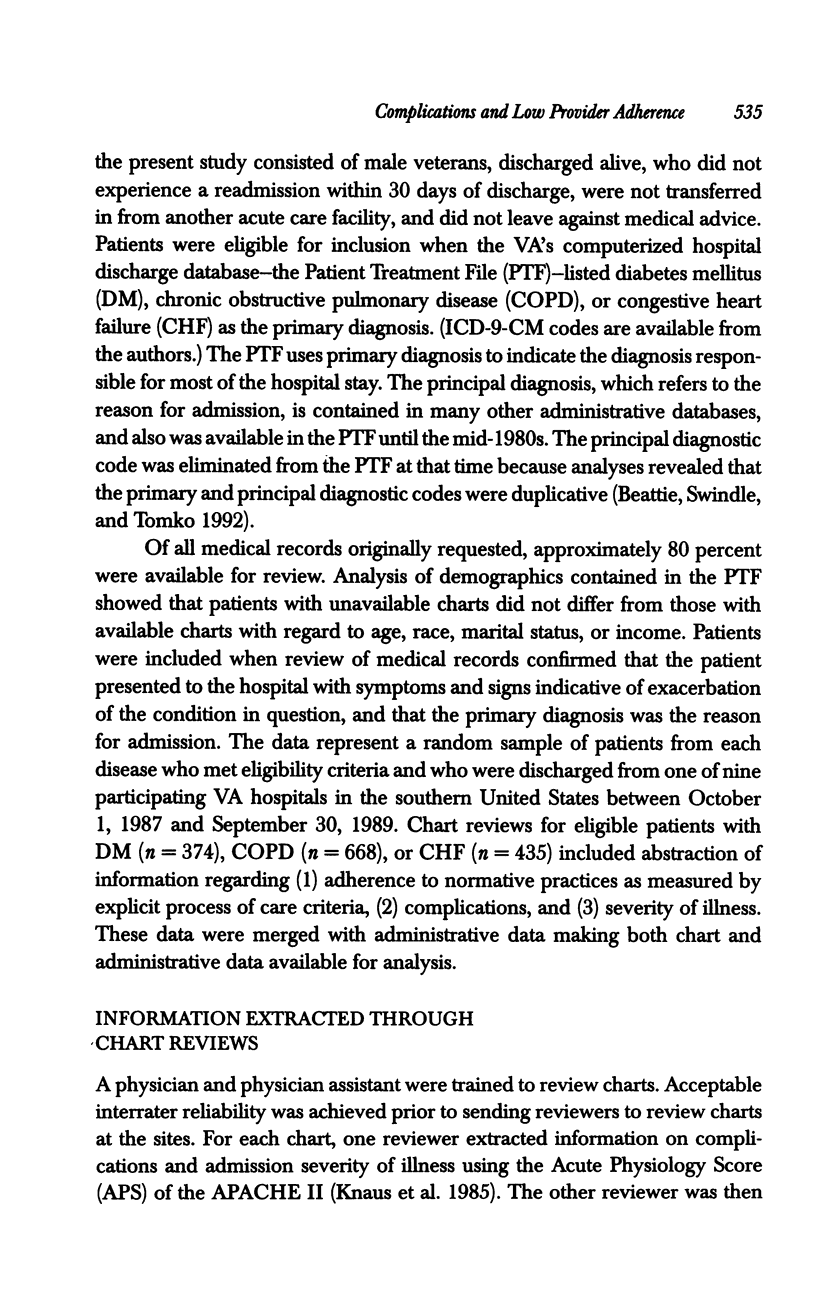
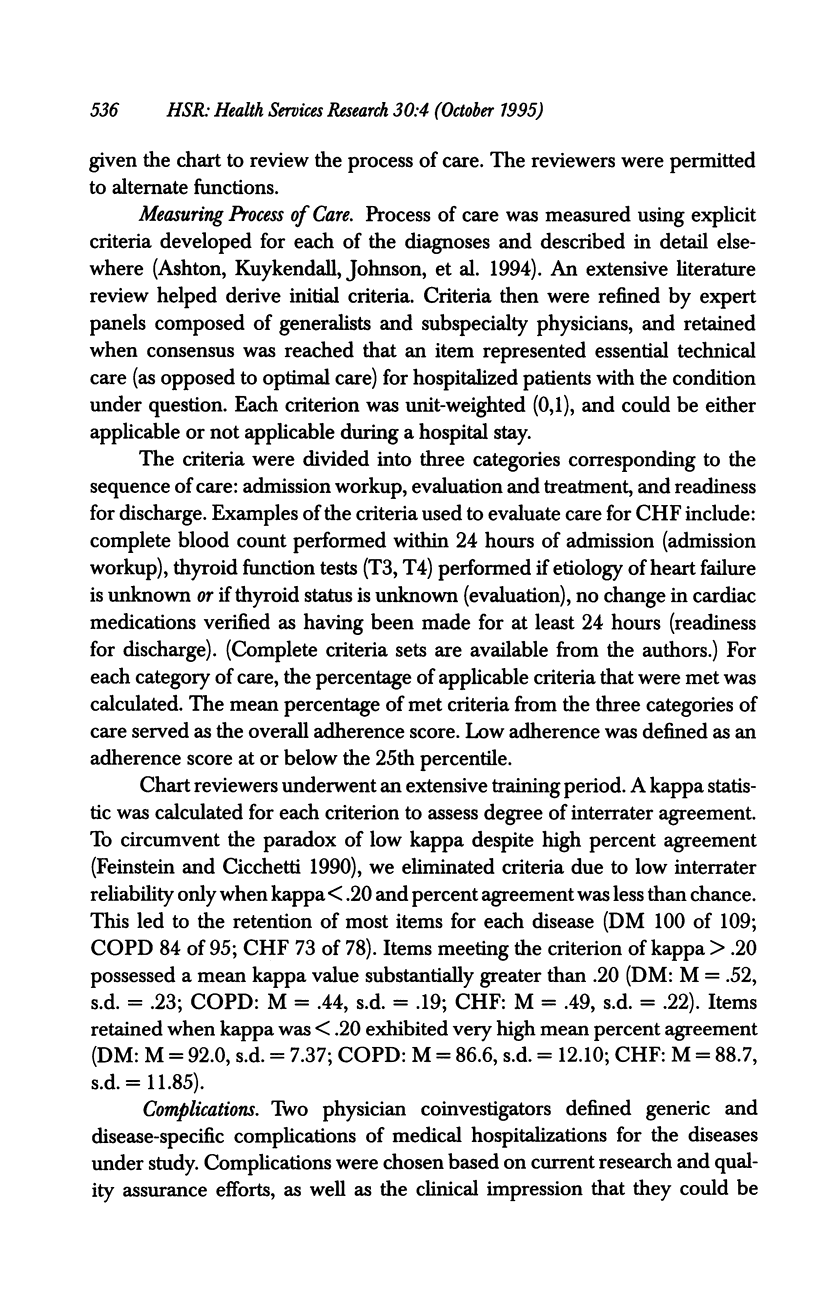
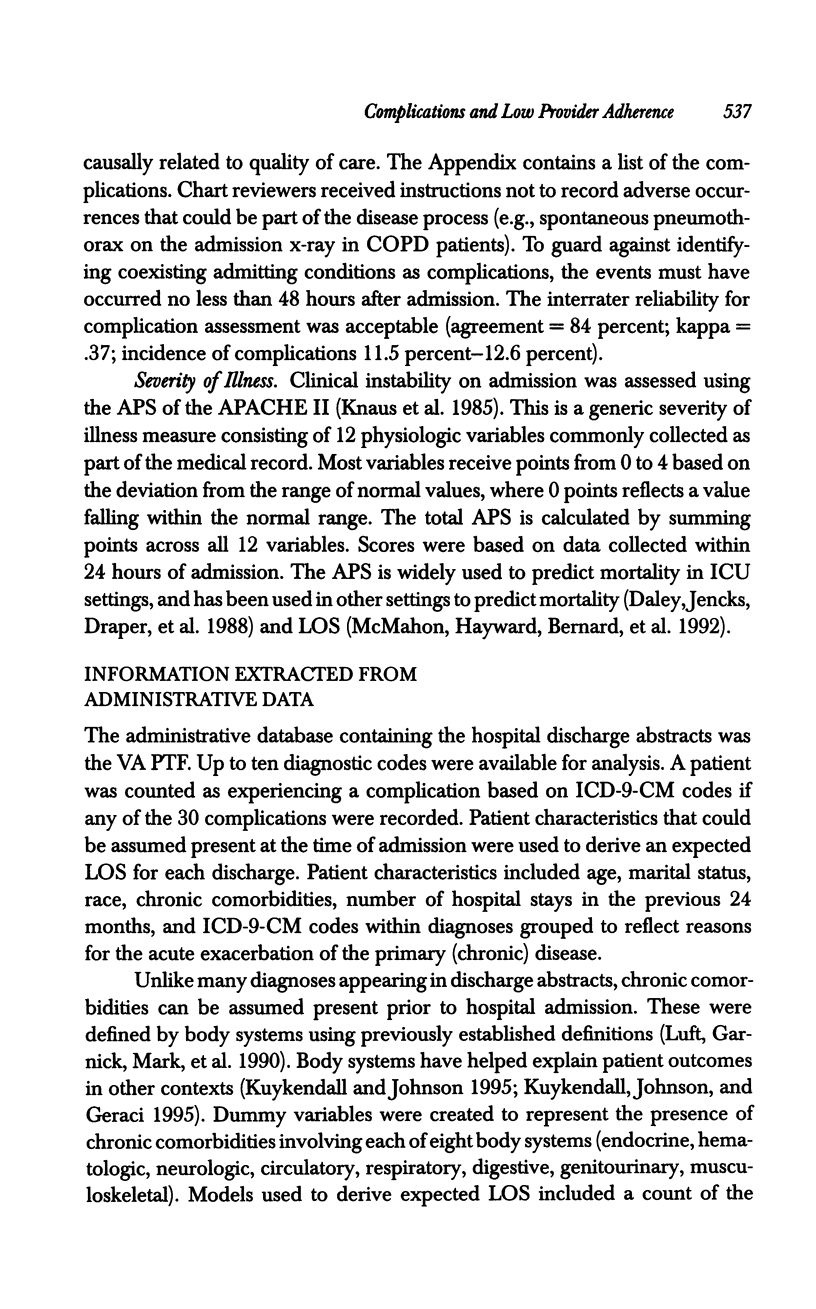
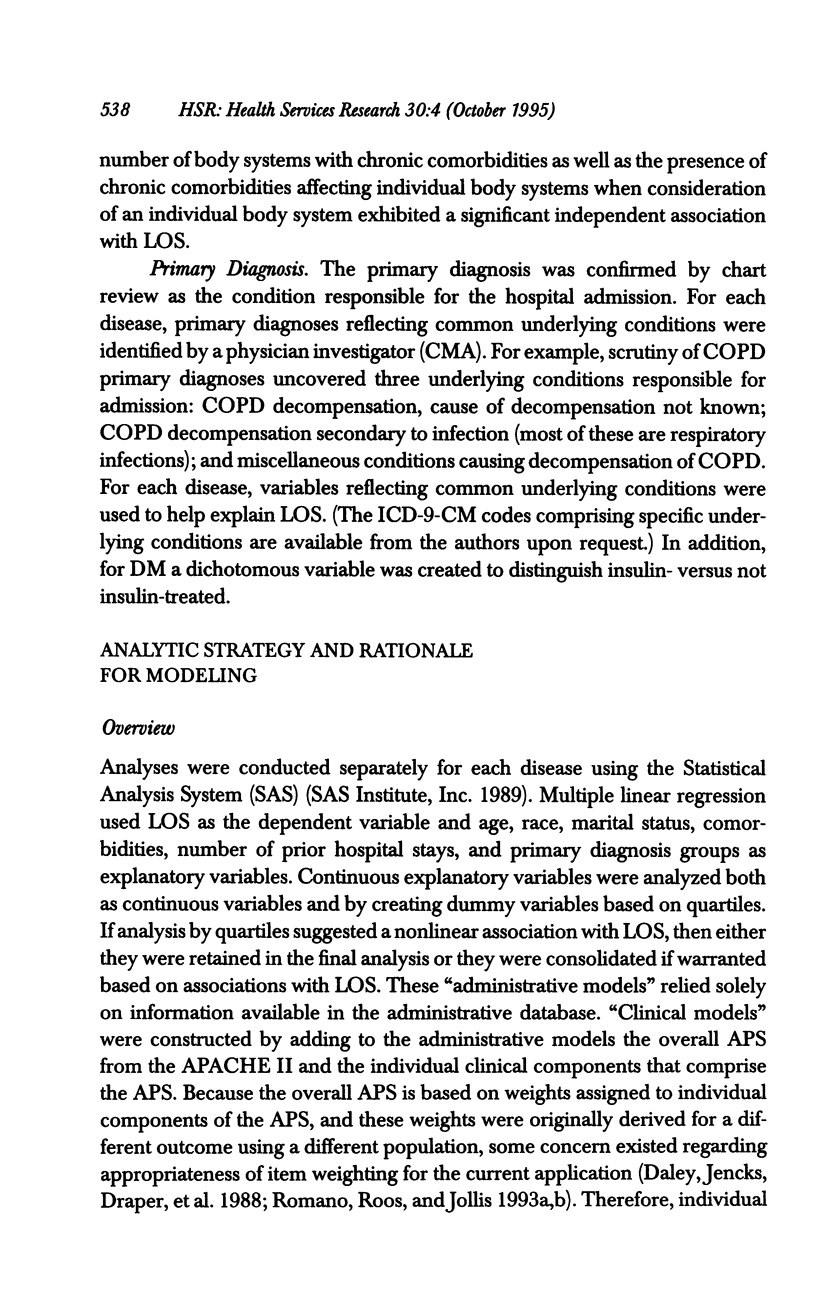
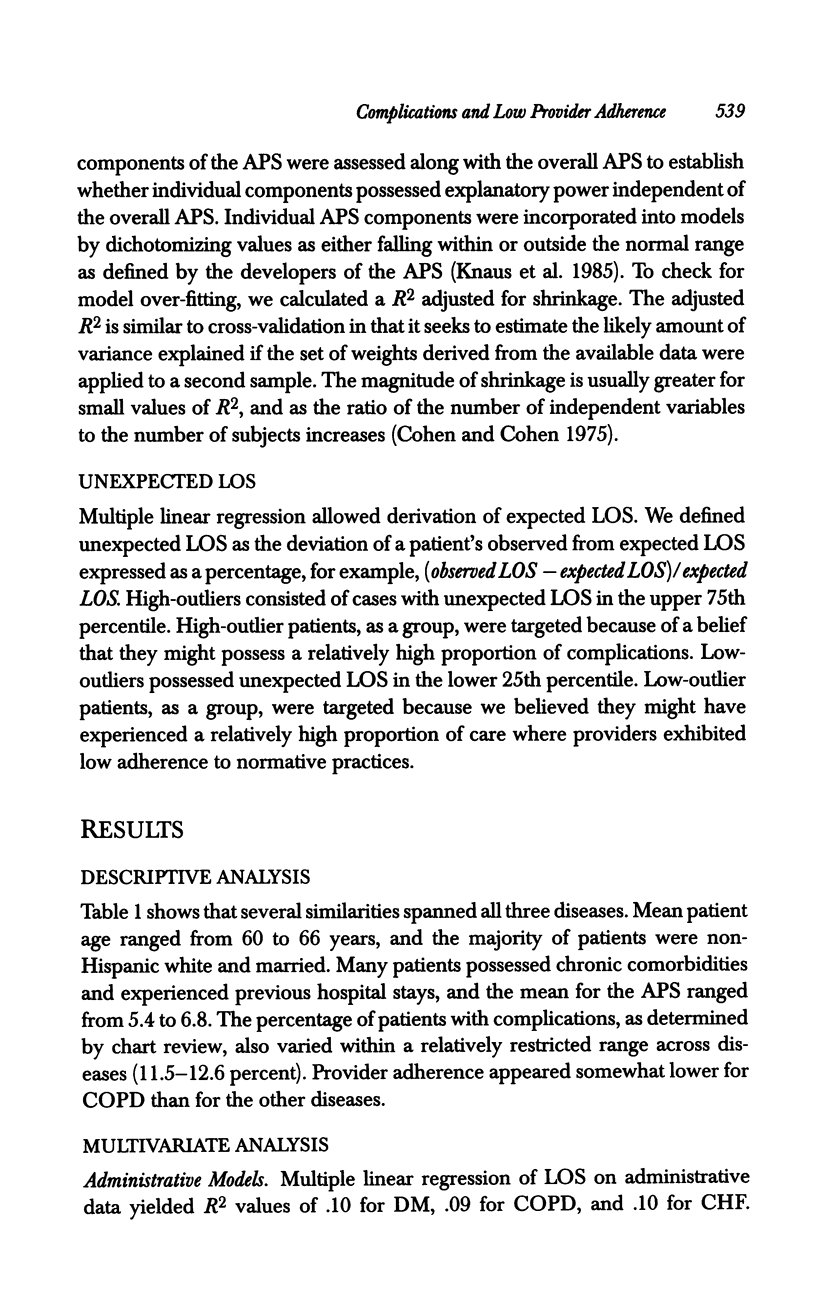
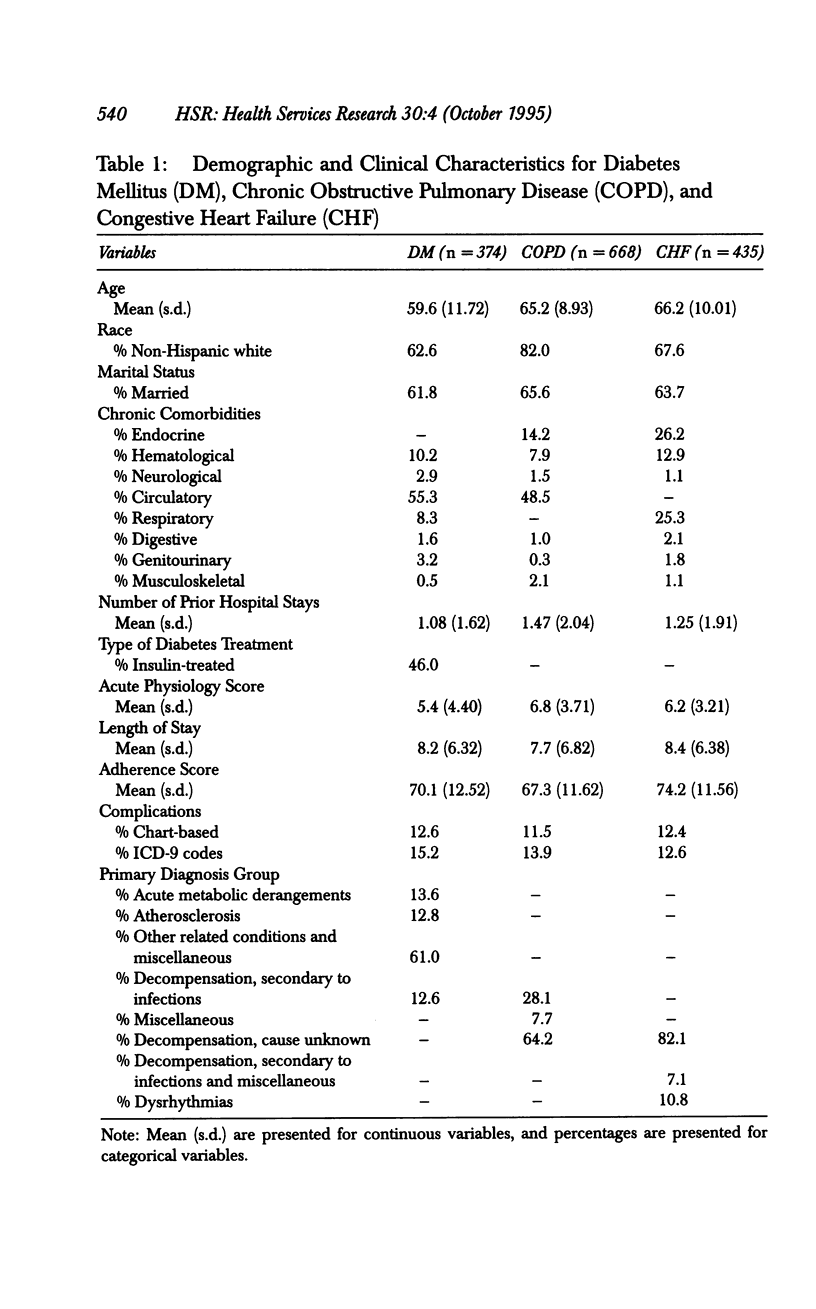
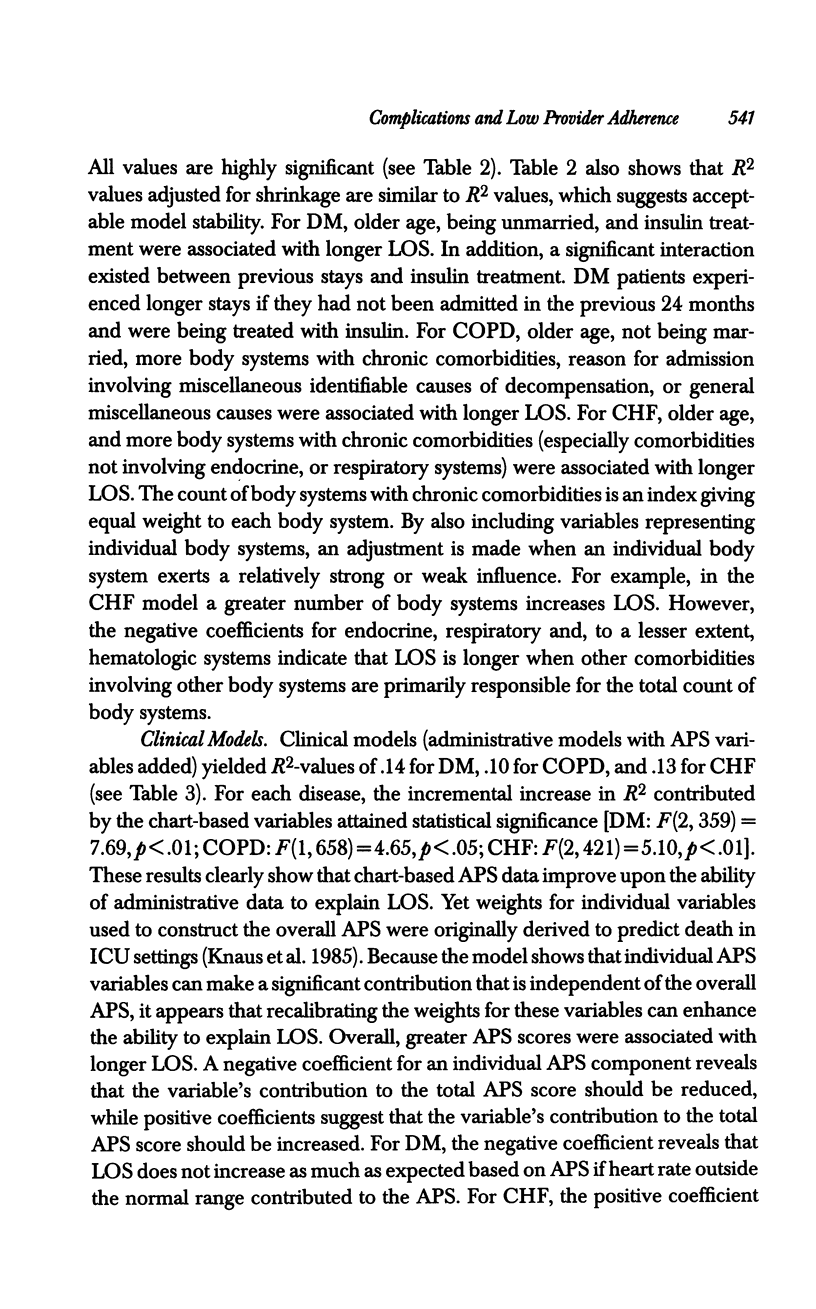
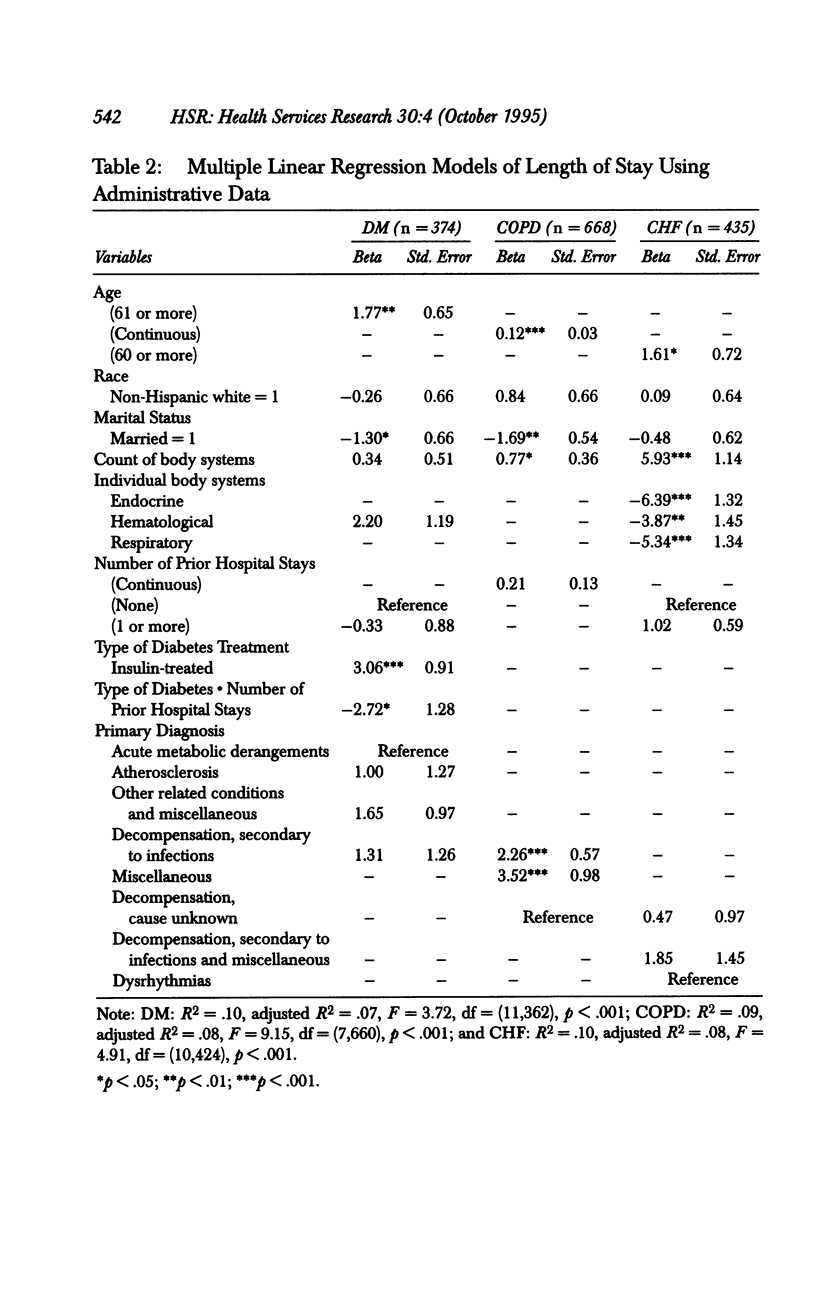
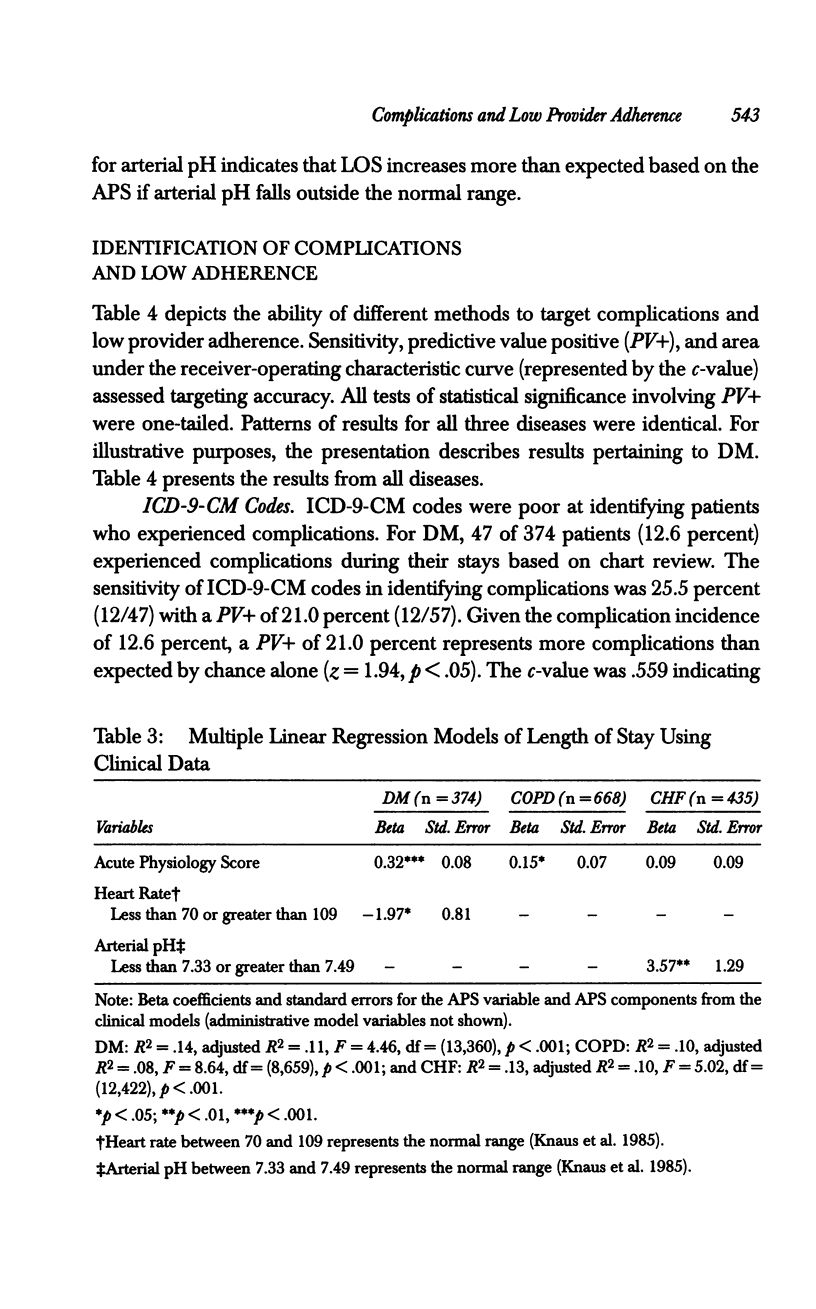
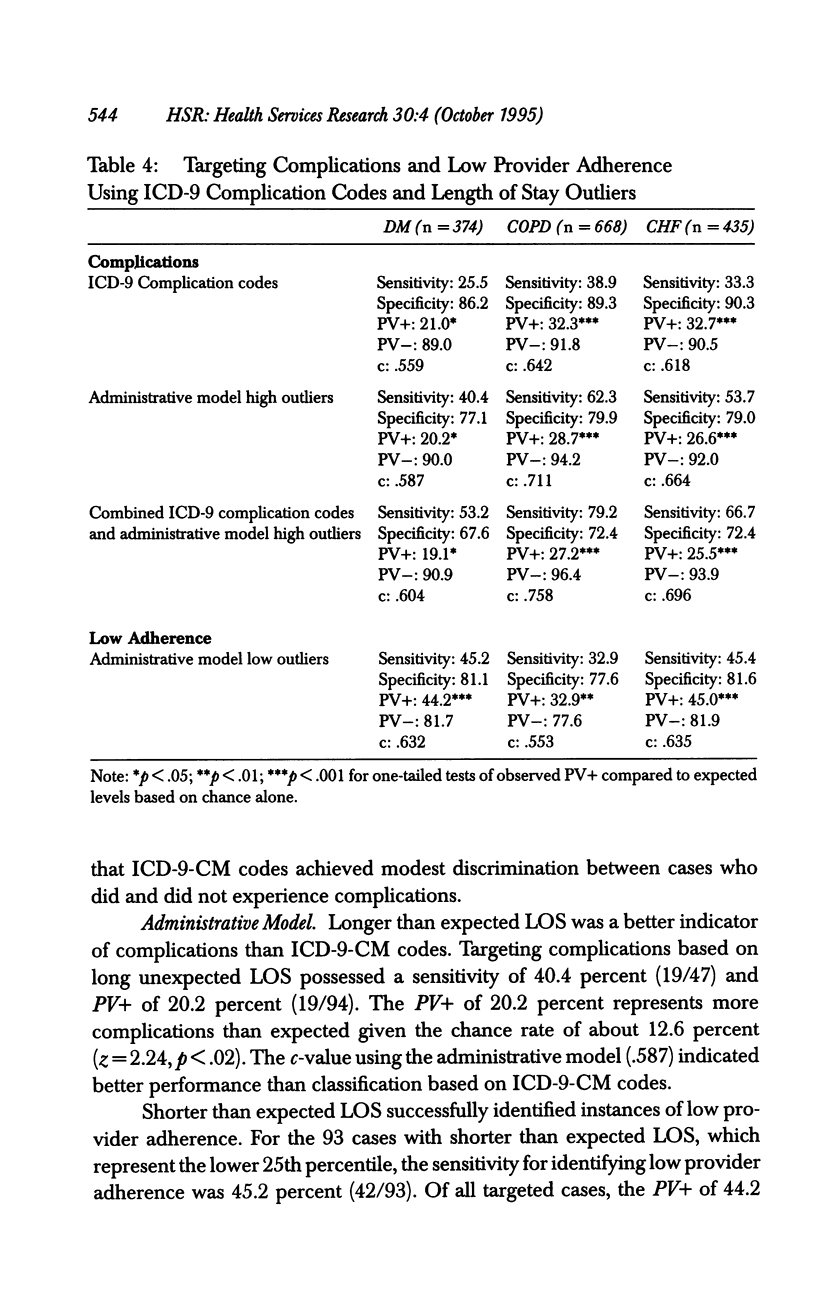
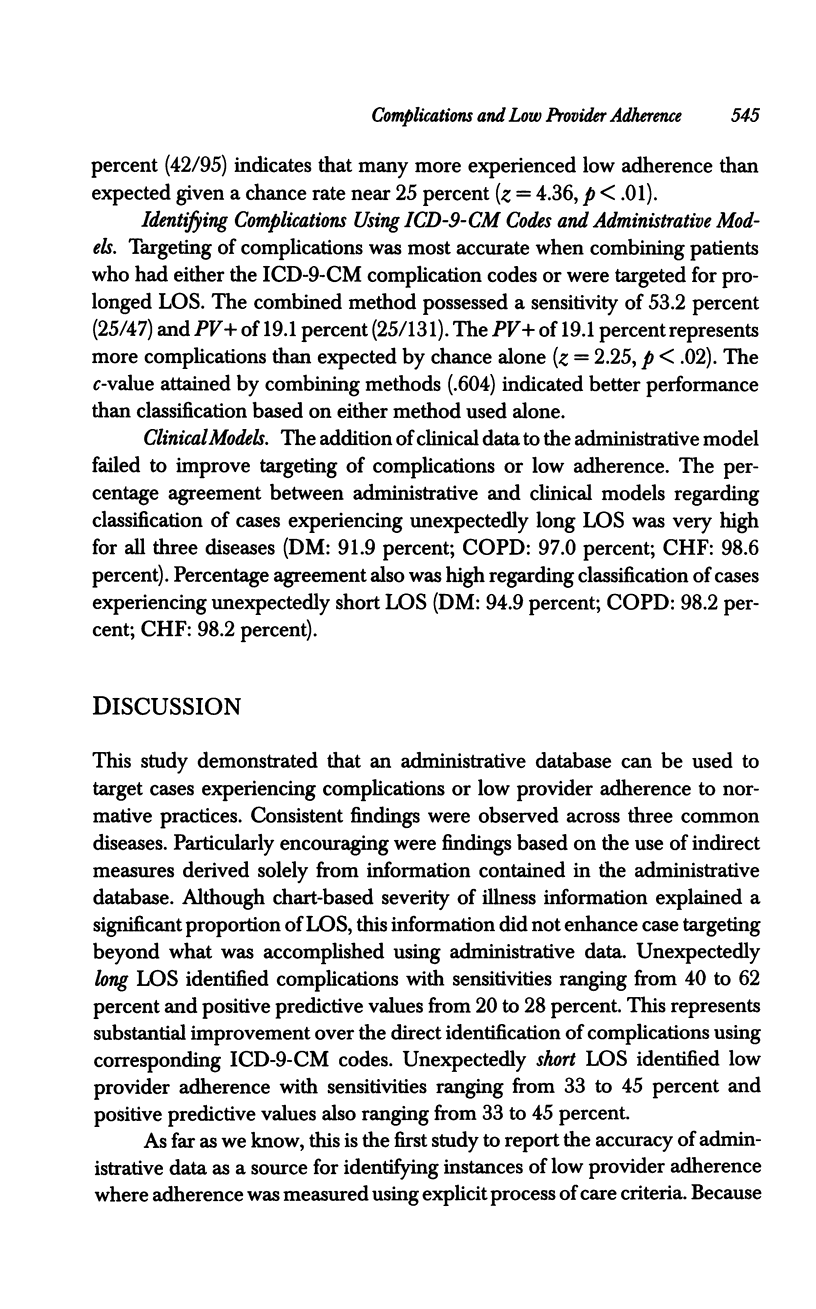
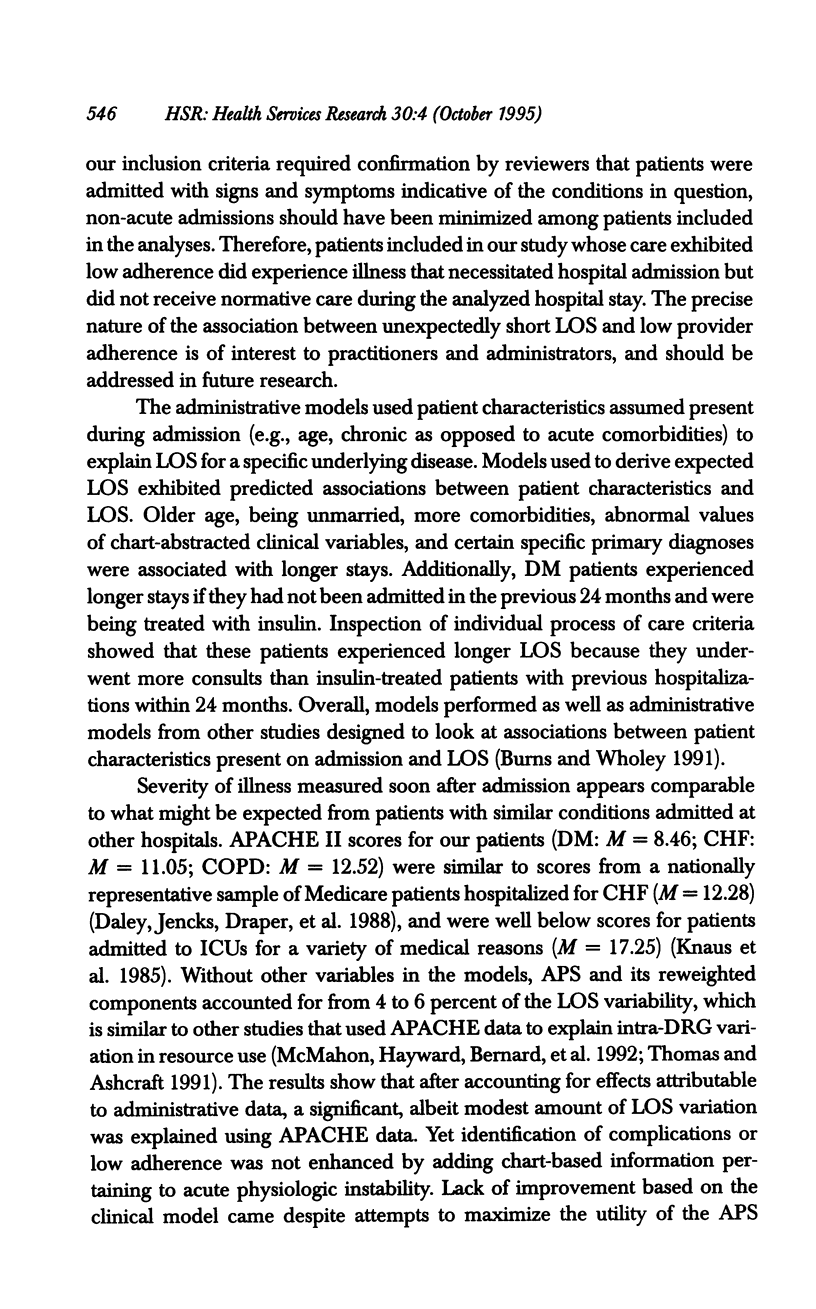
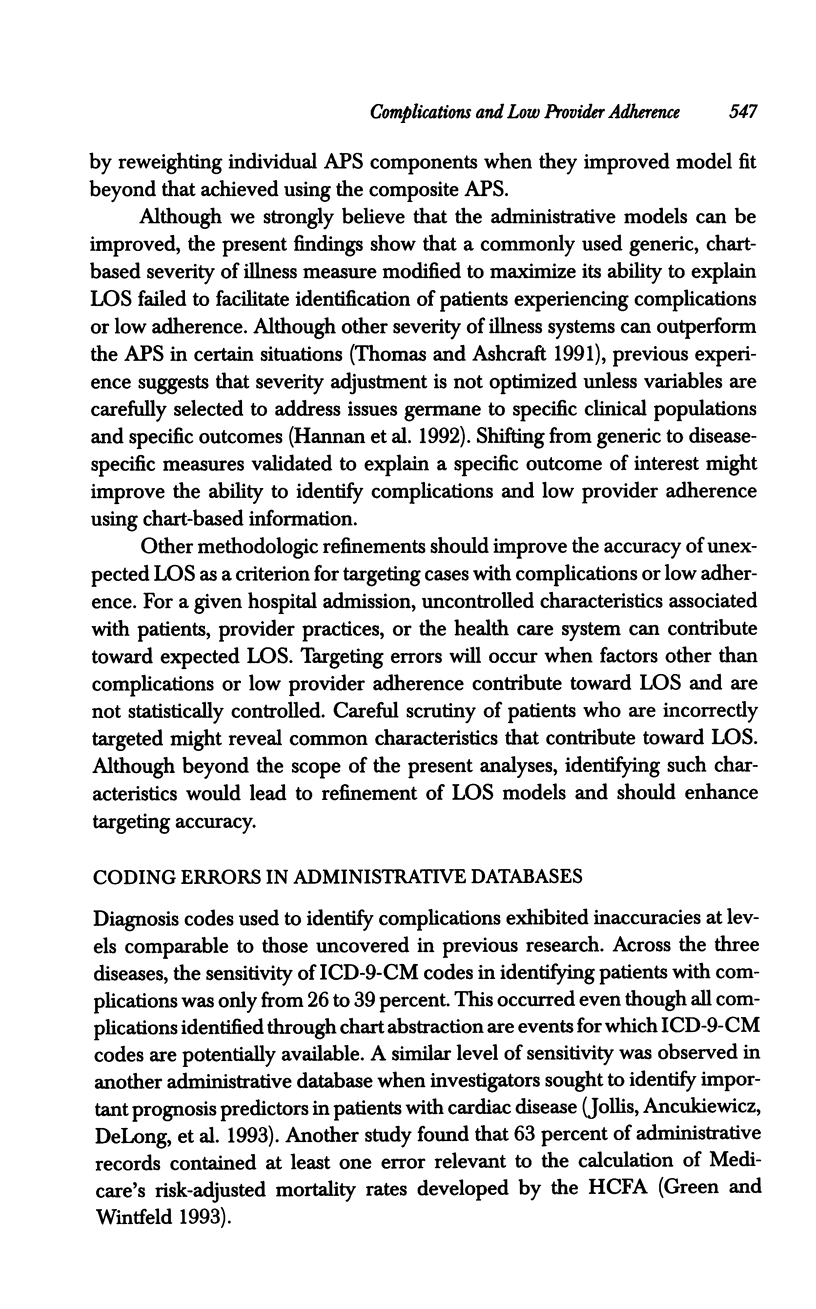
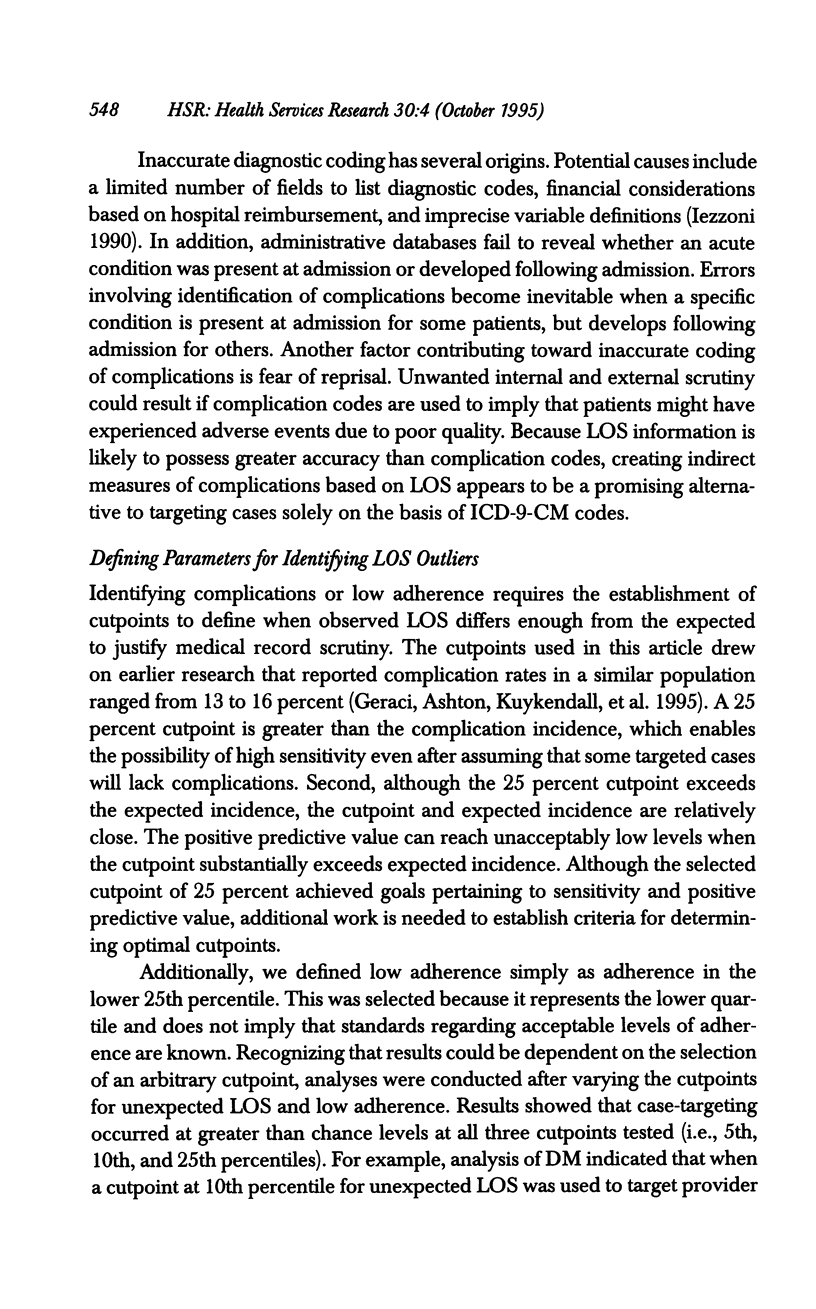
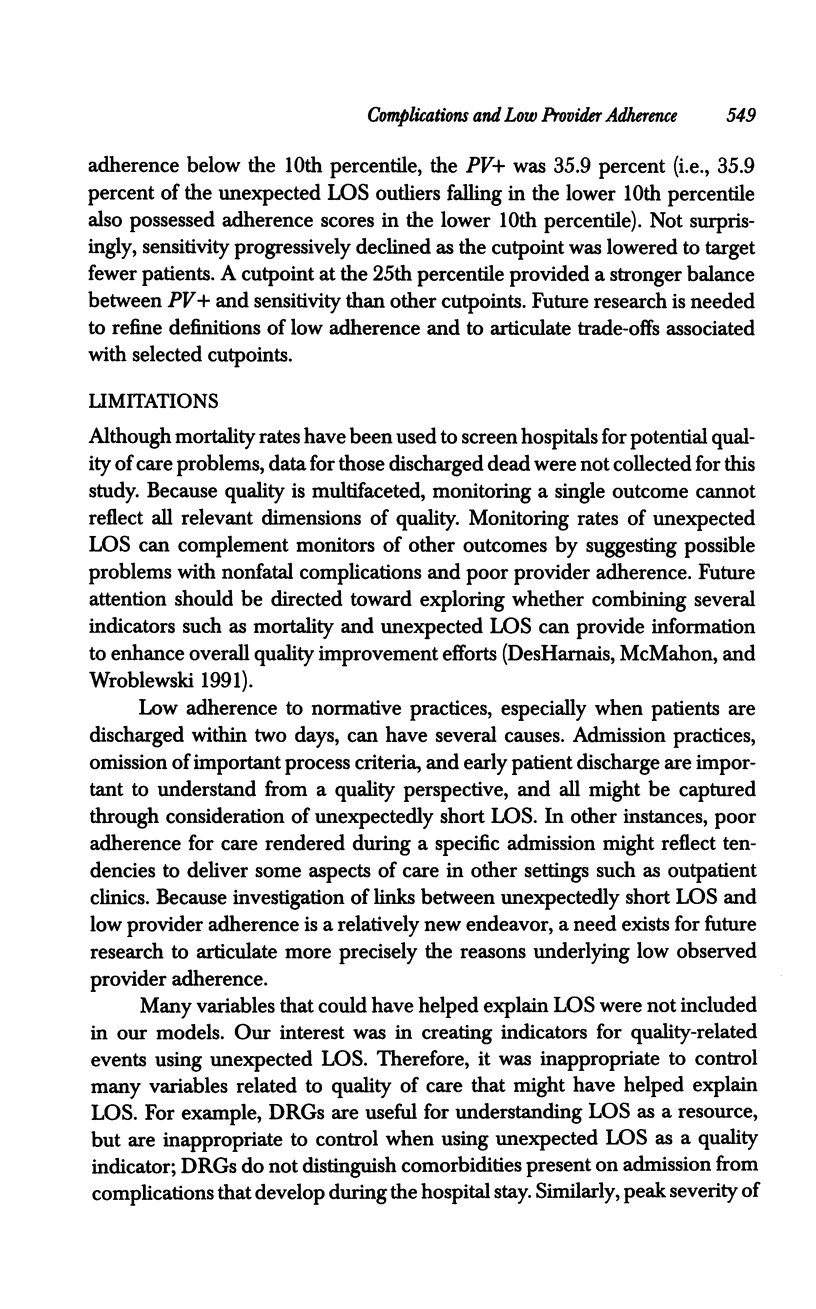
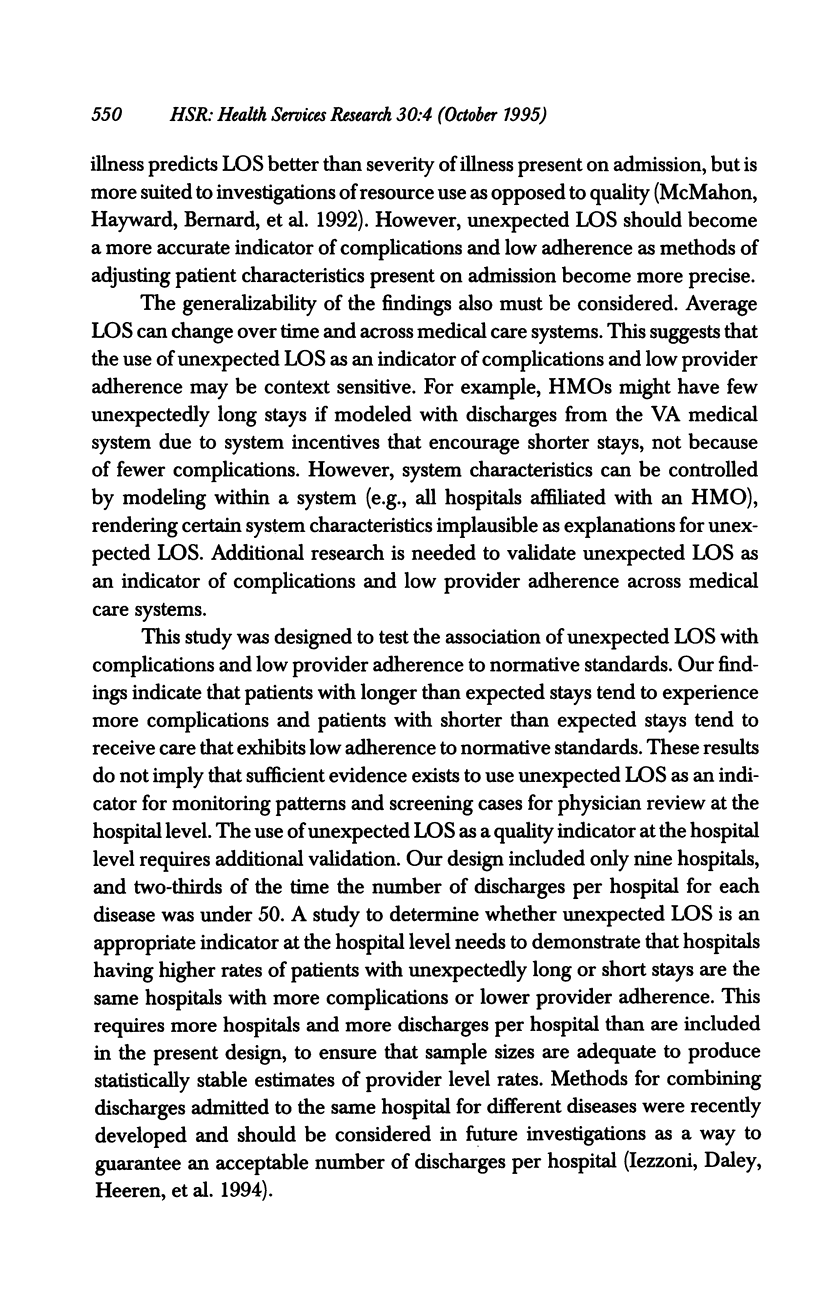
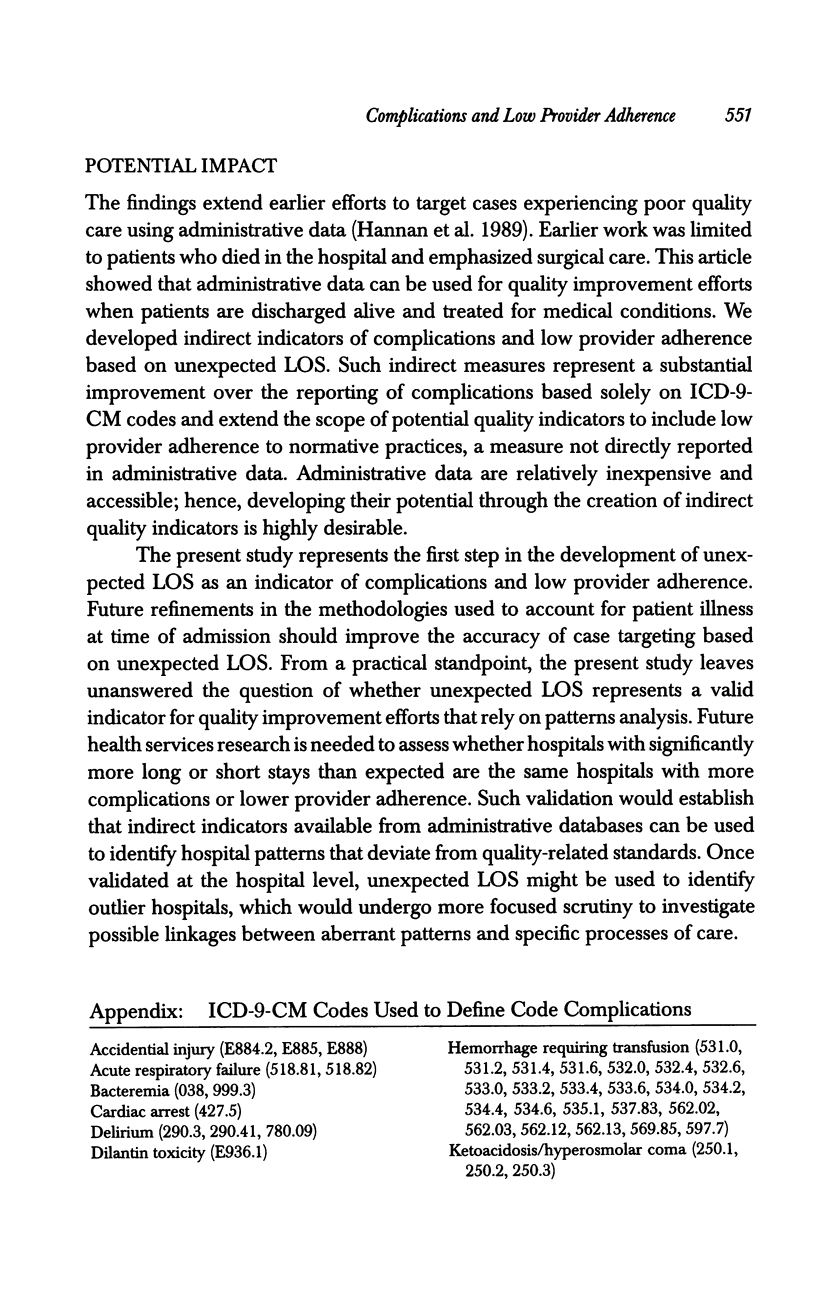
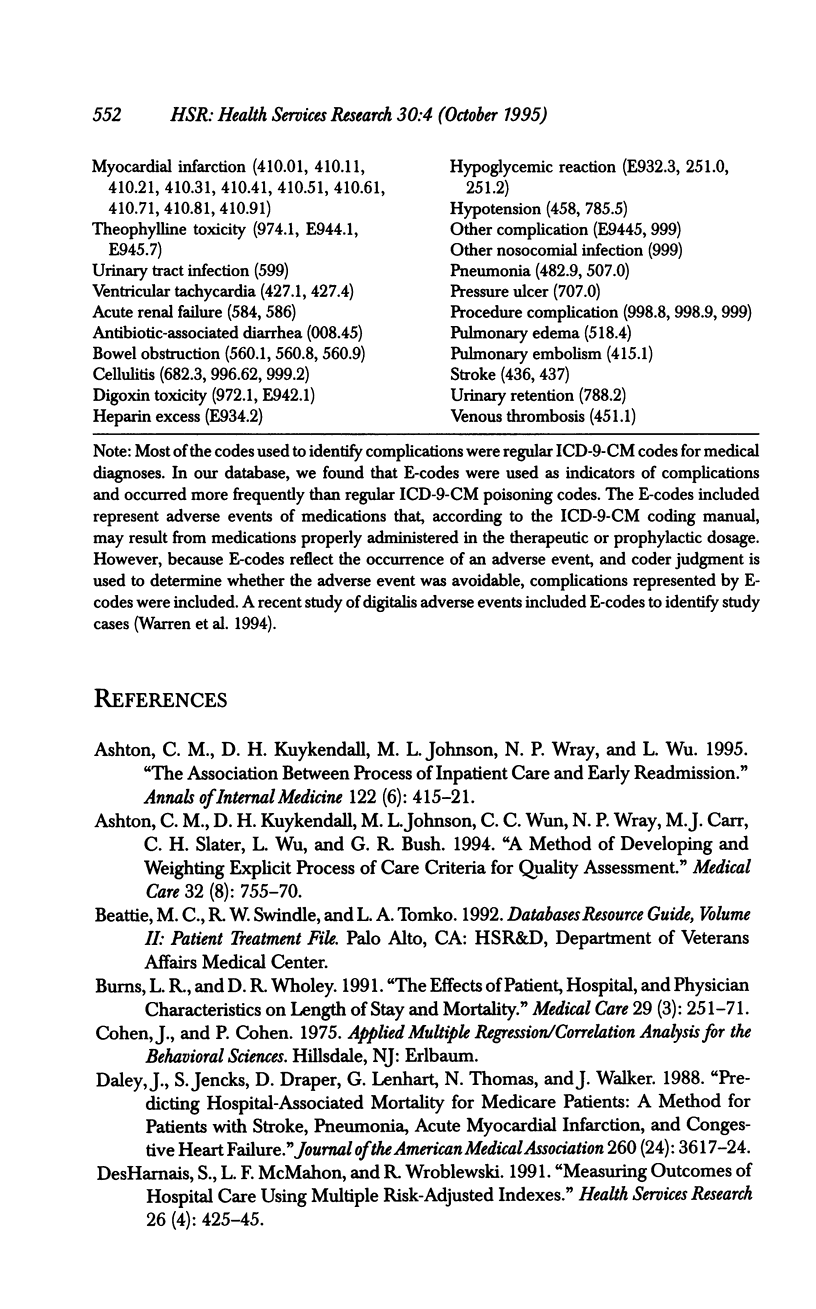
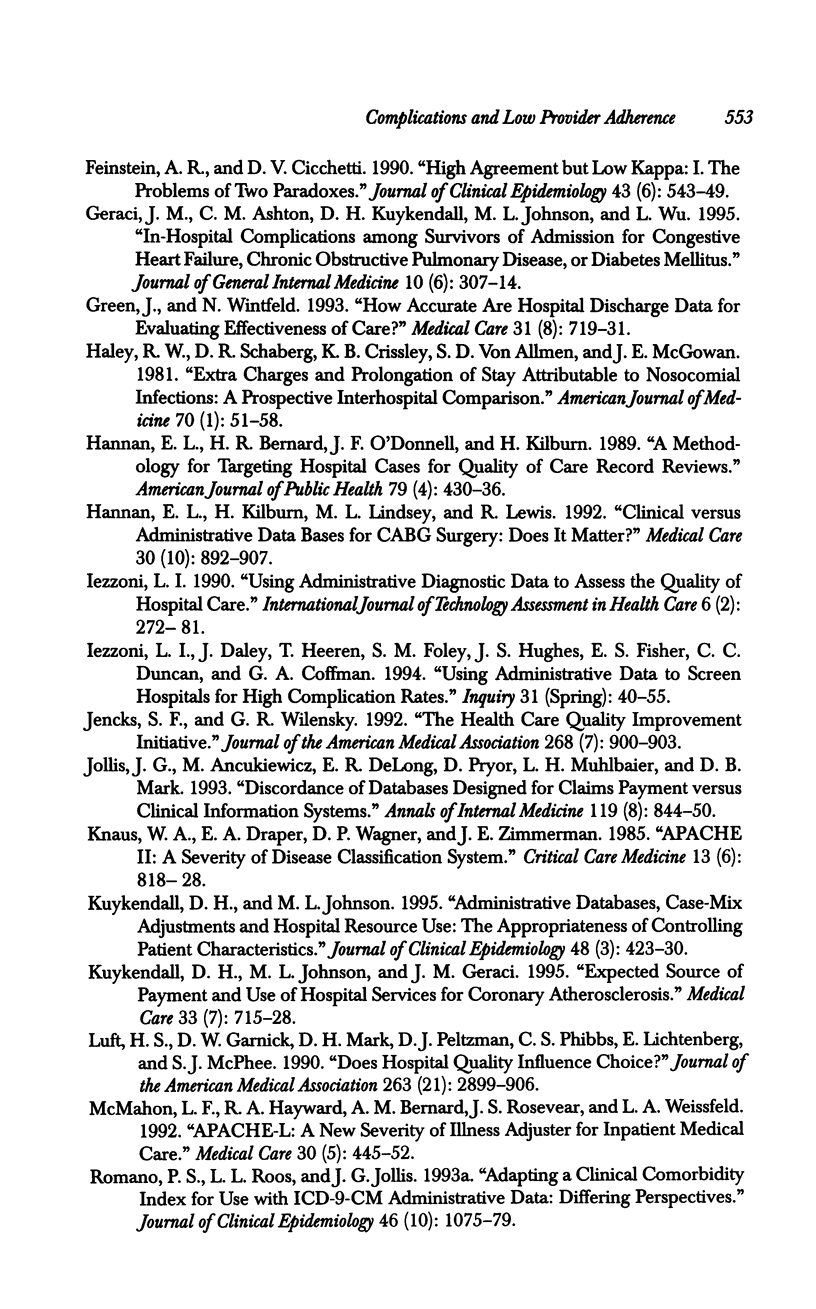
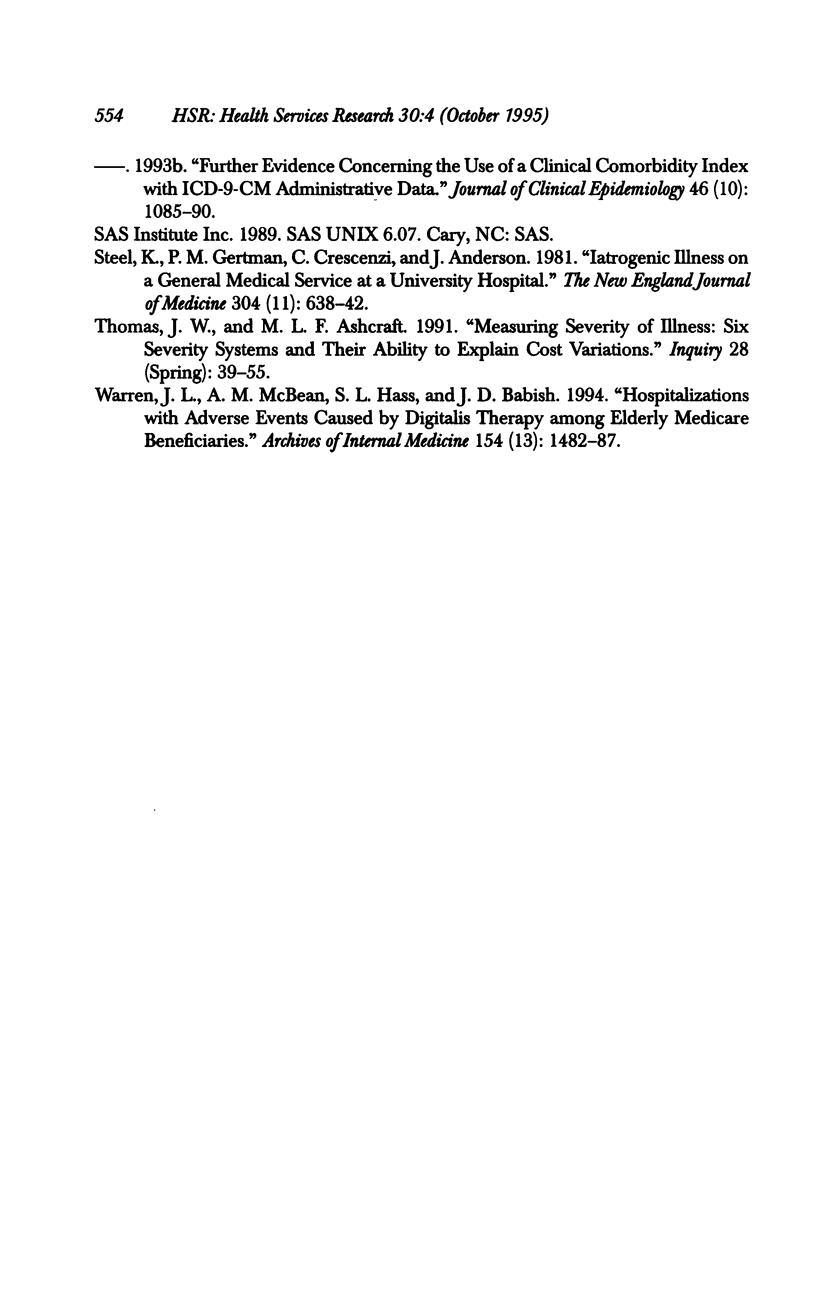
Selected References
These references are in PubMed. This may not be the complete list of references from this article.
- Ashton C. M., Kuykendall D. H., Johnson M. L., Wray N. P., Wu L. The association between the quality of inpatient care and early readmission. Ann Intern Med. 1995 Mar 15;122(6):415–421. doi: 10.7326/0003-4819-122-6-199503150-00003. [DOI] [PubMed] [Google Scholar]
- Ashton C. M., Kuykendall D. H., Johnson M. L., Wun C. C., Wray N. P., Carr M. J., Slater C. H., Wu L., Bush G. R. A method of developing and weighting explicit process of care criteria for quality assessment. Med Care. 1994 Aug;32(8):755–770. doi: 10.1097/00005650-199408000-00001. [DOI] [PubMed] [Google Scholar]
- Daley J., Jencks S., Draper D., Lenhart G., Thomas N., Walker J. Predicting hospital-associated mortality for Medicare patients. A method for patients with stroke, pneumonia, acute myocardial infarction, and congestive heart failure. JAMA. 1988 Dec 23;260(24):3617–3624. doi: 10.1001/jama.260.24.3617. [DOI] [PubMed] [Google Scholar]
- DesHarnais S., McMahon L. F., Jr, Wroblewski R. Measuring outcomes of hospital care using multiple risk-adjusted indexes. Health Serv Res. 1991 Oct;26(4):425–445. [PMC free article] [PubMed] [Google Scholar]
- Feinstein A. R., Cicchetti D. V. High agreement but low kappa: I. The problems of two paradoxes. J Clin Epidemiol. 1990;43(6):543–549. doi: 10.1016/0895-4356(90)90158-l. [DOI] [PubMed] [Google Scholar]
- Geraci J. M., Ashton C. M., Kuykendall D. H., Johnson M. L., Wu L. In-hospital complications among survivors of admission for congestive heart failure, chronic obstructive pulmonary disease, or diabetes mellitus. J Gen Intern Med. 1995 Jun;10(6):307–314. doi: 10.1007/BF02599949. [DOI] [PubMed] [Google Scholar]
- Green J., Wintfeld N. How accurate are hospital discharge data for evaluating effectiveness of care? Med Care. 1993 Aug;31(8):719–731. doi: 10.1097/00005650-199308000-00005. [DOI] [PubMed] [Google Scholar]
- Haley R. W., Schaberg D. R., Crossley K. B., Von Allmen S. D., McGowan J. E., Jr Extra charges and prolongation of stay attributable to nosocomial infections: a prospective interhospital comparison. Am J Med. 1981 Jan;70(1):51–58. doi: 10.1016/0002-9343(81)90411-3. [DOI] [PubMed] [Google Scholar]
- Hannan E. L., Bernard H. R., O'Donnell J. F., Kilburn H., Jr A methodology for targeting hospital cases for quality of care record reviews. Am J Public Health. 1989 Apr;79(4):430–436. doi: 10.2105/ajph.79.4.430. [DOI] [PMC free article] [PubMed] [Google Scholar]
- Hannan E. L., Kilburn H., Jr, Lindsey M. L., Lewis R. Clinical versus administrative data bases for CABG surgery. Does it matter? Med Care. 1992 Oct;30(10):892–907. doi: 10.1097/00005650-199210000-00002. [DOI] [PubMed] [Google Scholar]
- Iezzoni L. I., Daley J., Heeren T., Foley S. M., Hughes J. S., Fisher E. S., Duncan C. C., Coffman G. A. Using administrative data to screen hospitals for high complication rates. Inquiry. 1994 Spring;31(1):40–55. [PubMed] [Google Scholar]
- Iezzoni L. I. Using administrative diagnostic data to assess the quality of hospital care. Pitfalls and potential of ICD-9-CM. Int J Technol Assess Health Care. 1990;6(2):272–281. doi: 10.1017/s0266462300000799. [DOI] [PubMed] [Google Scholar]
- Jencks S. F., Wilensky G. R. The health care quality improvement initiative. A new approach to quality assurance in Medicare. JAMA. 1992 Aug 19;268(7):900–903. [PubMed] [Google Scholar]
- Jollis J. G., Ancukiewicz M., DeLong E. R., Pryor D. B., Muhlbaier L. H., Mark D. B. Discordance of databases designed for claims payment versus clinical information systems. Implications for outcomes research. Ann Intern Med. 1993 Oct 15;119(8):844–850. doi: 10.7326/0003-4819-119-8-199310150-00011. [DOI] [PubMed] [Google Scholar]
- Knaus W. A., Draper E. A., Wagner D. P., Zimmerman J. E. APACHE II: a severity of disease classification system. Crit Care Med. 1985 Oct;13(10):818–829. [PubMed] [Google Scholar]
- Kuykendall D. H., Johnson M. L. Administrative databases, case-mix adjustments and hospital resource use: the appropriateness of controlling patient characteristics. J Clin Epidemiol. 1995 Mar;48(3):423–430. doi: 10.1016/0895-4356(94)00140-l. [DOI] [PubMed] [Google Scholar]
- Kuykendall D. H., Johnson M. L., Geraci J. M. Expected source of payment and use of hospital services for coronary atherosclerosis. Med Care. 1995 Jul;33(7):715–728. doi: 10.1097/00005650-199507000-00007. [DOI] [PubMed] [Google Scholar]
- Luft H. S., Garnick D. W., Mark D. H., Peltzman D. J., Phibbs C. S., Lichtenberg E., McPhee S. J. Does quality influence choice of hospital? JAMA. 1990 Jun 6;263(21):2899–2906. [PubMed] [Google Scholar]
- McMahon L. F., Jr, Hayward R. A., Bernard A. M., Rosevear J. S., Weissfeld L. A. APACHE-L: a new severity of illness adjuster for inpatient medical care. Med Care. 1992 May;30(5):445–452. doi: 10.1097/00005650-199205000-00006. [DOI] [PubMed] [Google Scholar]
- Romano P. S., Roos L. L., Jollis J. G. Adapting a clinical comorbidity index for use with ICD-9-CM administrative data: differing perspectives. J Clin Epidemiol. 1993 Oct;46(10):1075–1090. doi: 10.1016/0895-4356(93)90103-8. [DOI] [PubMed] [Google Scholar]
- Steel K., Gertman P. M., Crescenzi C., Anderson J. Iatrogenic illness on a general medical service at a university hospital. N Engl J Med. 1981 Mar 12;304(11):638–642. doi: 10.1056/NEJM198103123041104. [DOI] [PubMed] [Google Scholar]
- Thomas J. W., Ashcraft M. L. Measuring severity of illness: six severity systems and their ability to explain cost variations. Inquiry. 1991 Spring;28(1):39–55. [PubMed] [Google Scholar]
- Warren J. L., McBean A. M., Hass S. L., Babish J. D. Hospitalizations with adverse events caused by digitalis therapy among elderly Medicare beneficiaries. Arch Intern Med. 1994 Jul 11;154(13):1482–1487. [PubMed] [Google Scholar]