Abstract
OBJECTIVE: The authors sought to train an artificial neural network to predict early outcomes after orthotopic liver transplantation. SUMMARY BACKGROUND DATA: Reliable prediction of outcomes early after liver transplantation would help improve organ use and could have an impact on patient survival, but remains an elusive goal. Traditional multivariate models have failed to attain the sensitivity and specificity required for practical clinical use. Alternate approaches that can help us model clinical phenomena must be explored. One such approach is the use of artificial neural networks, or connectionist models. These are computation systems that process information in parallel, using large numbers of simple units, and excel in tasks involving pattern recognition. They are capable of adaptive learning and self-organization, and exhibit a high degree of fault tolerance. METHODS: Ten feed-forward, back-propagation neural networks were trained to predict graft outcomes, using data from 155 adult liver transplants. The data included information that was available by the second postoperative day. Ten separate training and testing data subsets were prepared, using random sampling, and the ability of the different networks to predict outcomes successfully was evaluated using receiver operating characteristic (ROC) curve analysis. RESULTS: Four of the networks showed perfect discrimination, with an area under the ROC curve (Az) of 1.0. Two other networks also had excellent performance, with an Az of 0.95. The sensitivity and specificity of the combined networks was 60% and 100%, respectively, when using an output neuron activation of 0.6 as the cutoff point to decide class membership. Lowering the cutoff point to 0.14 increased the sensitivity to 77%, and lowered the specificity to 96%. CONCLUSIONS: These results are encouraging, especially when compared to the performance of more traditional multivariate models on the same data set. The robustness of neural networks, when confronted with noisy data generated by nonlinear processes, and their freedom from a priori assumptions regarding the data, make them promising tools with which to develop predictive clinical models.
Full text
PDF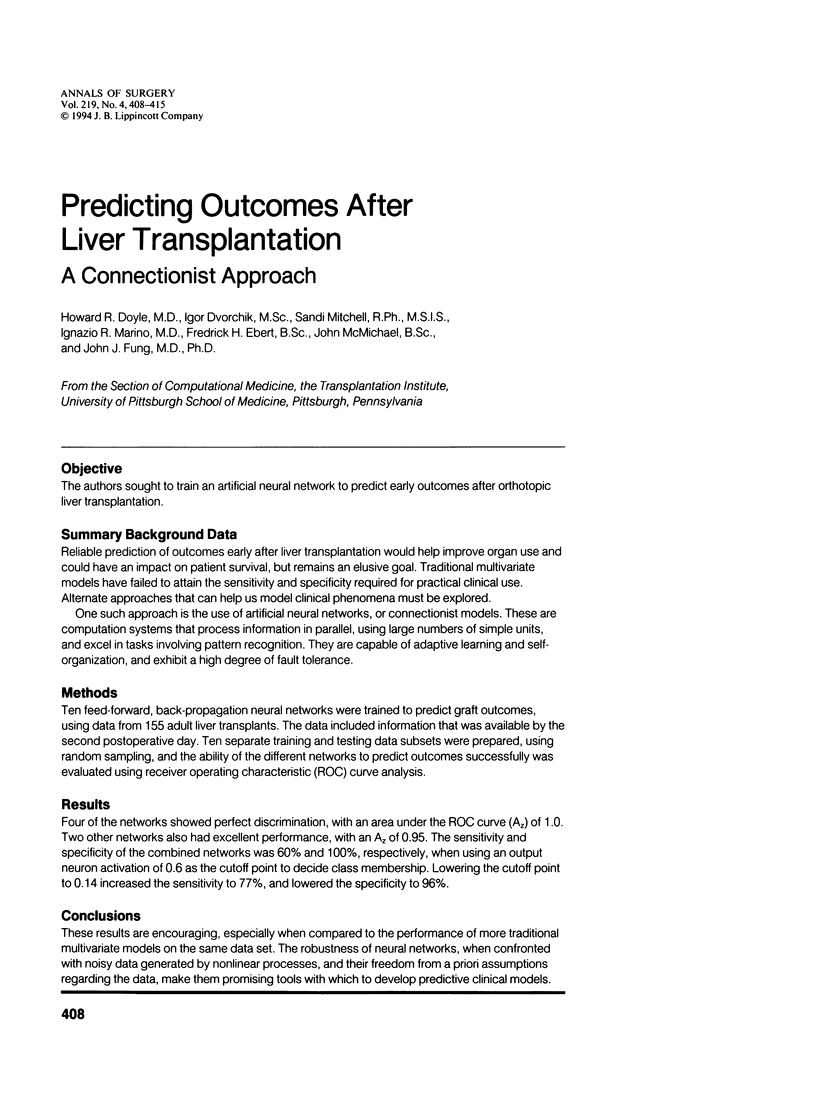
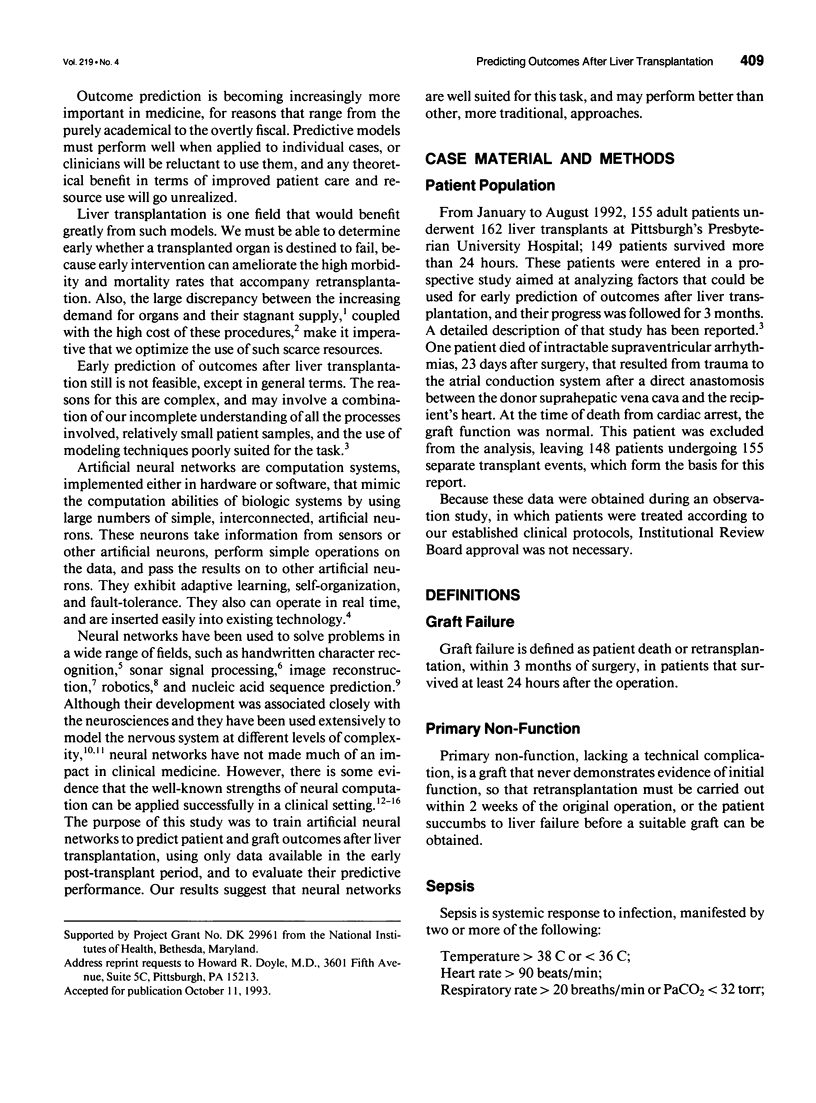
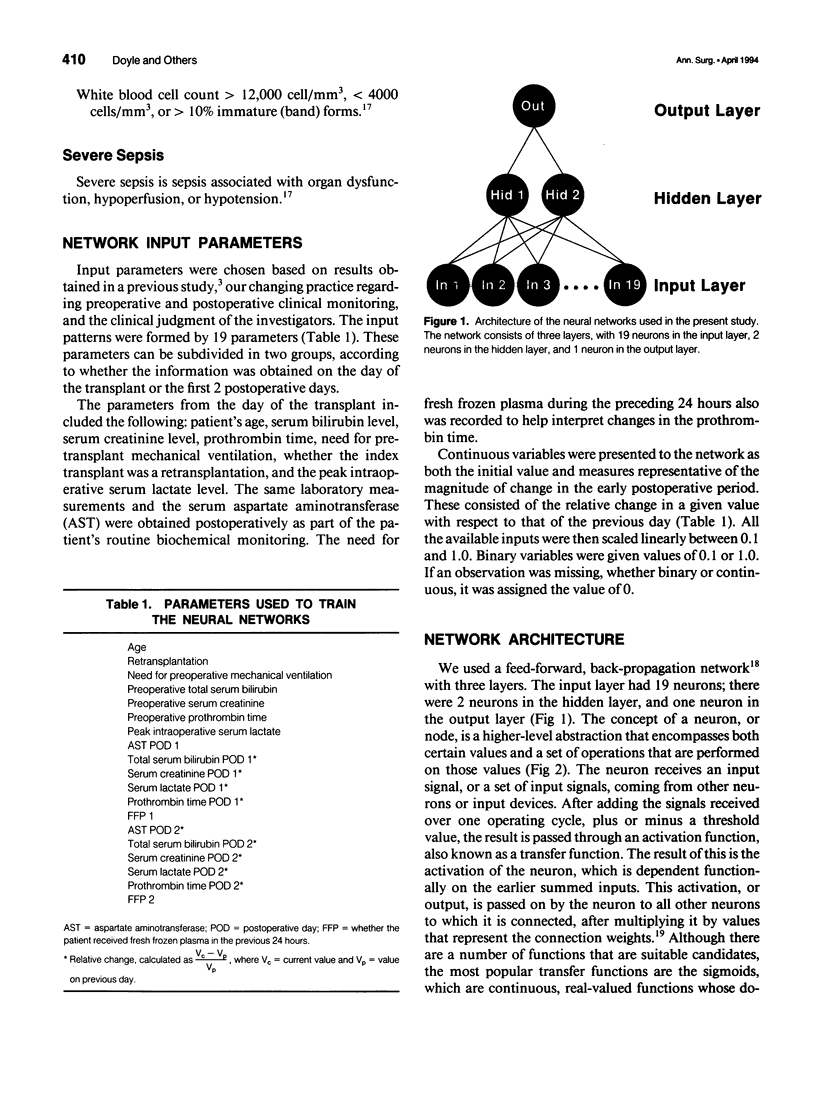
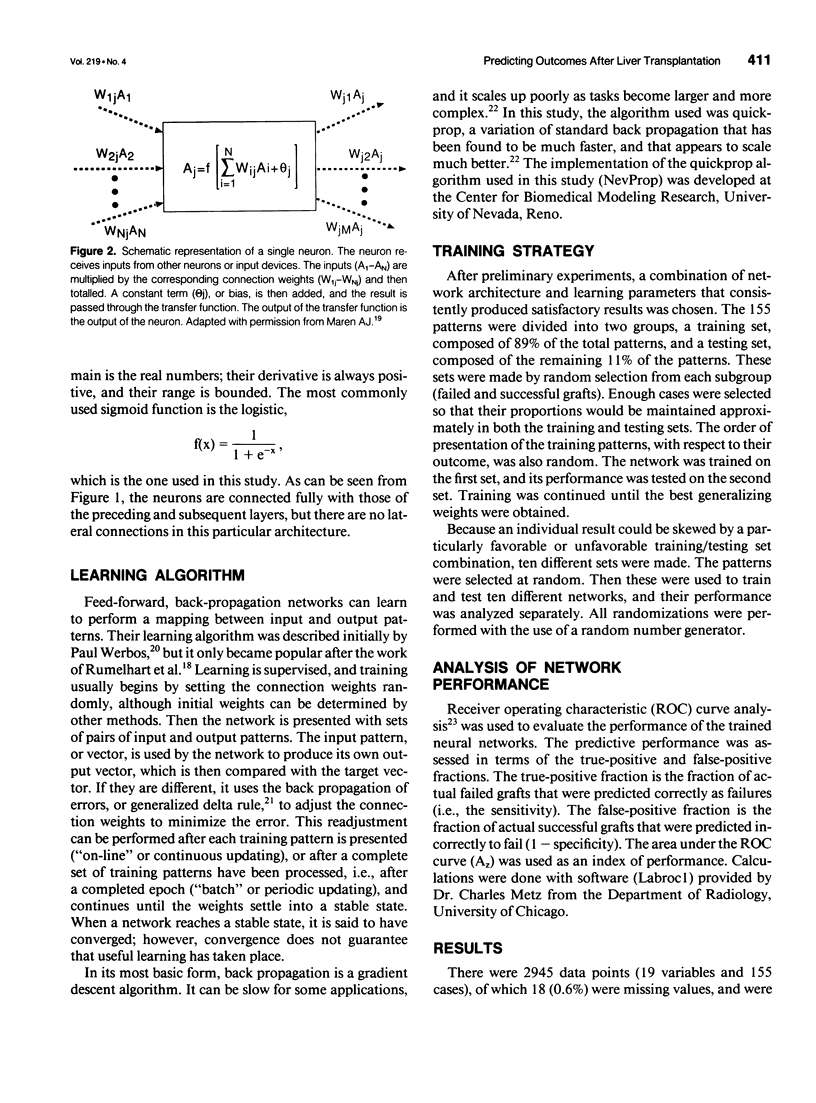
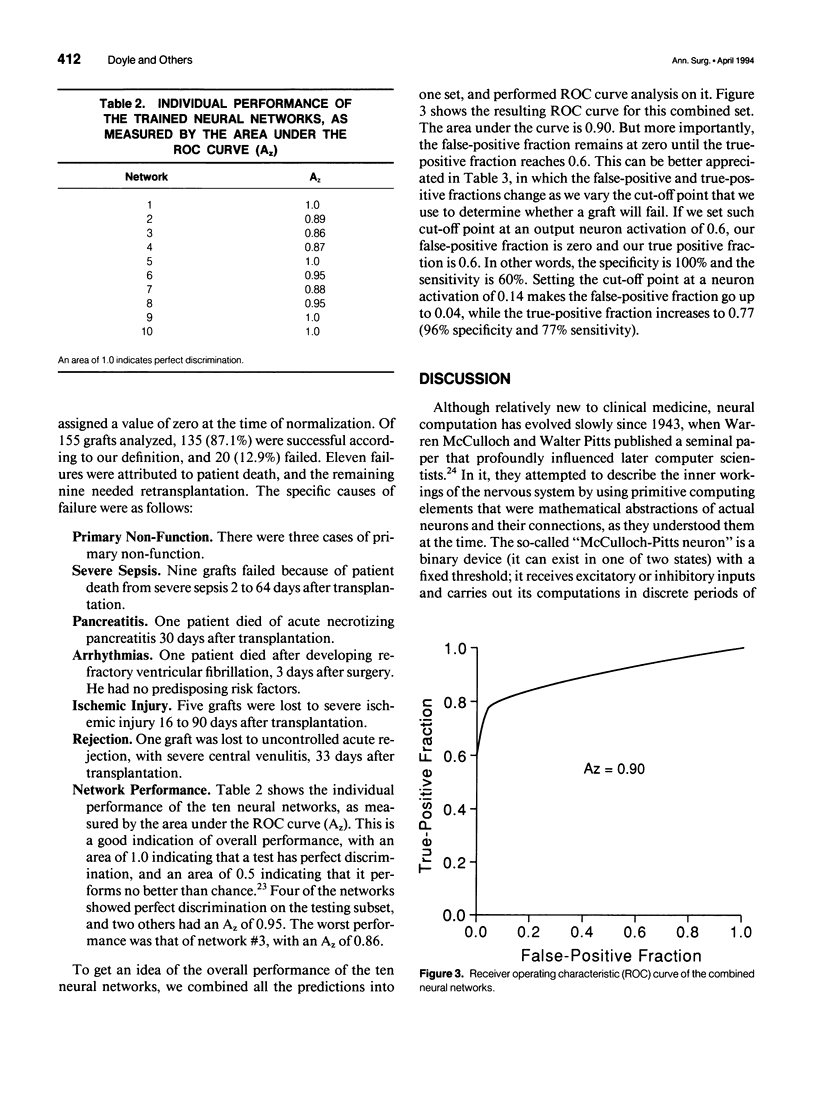
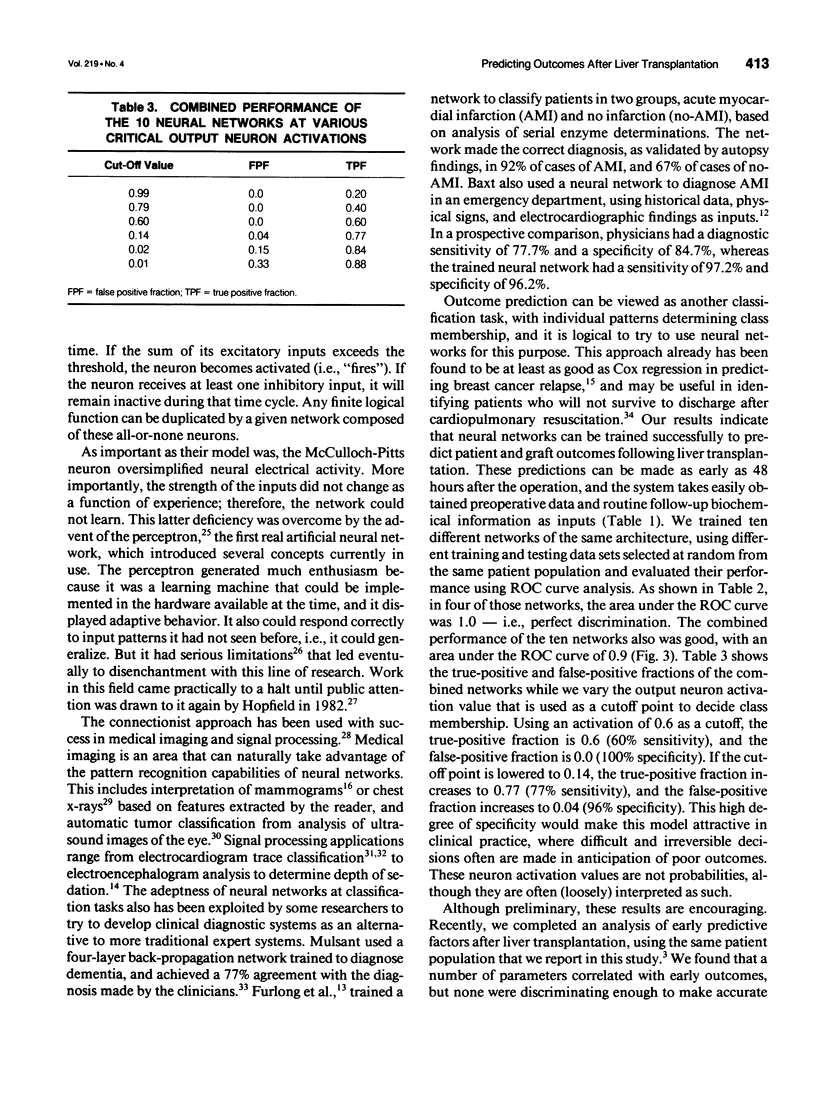
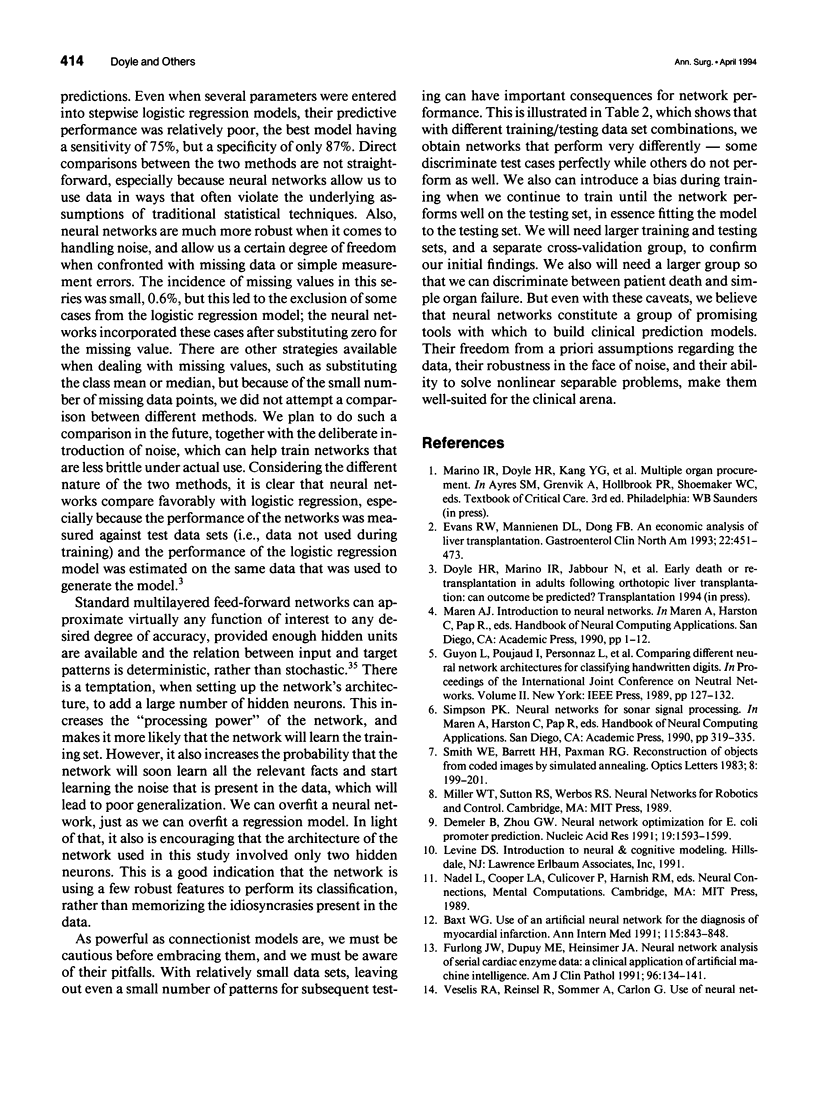
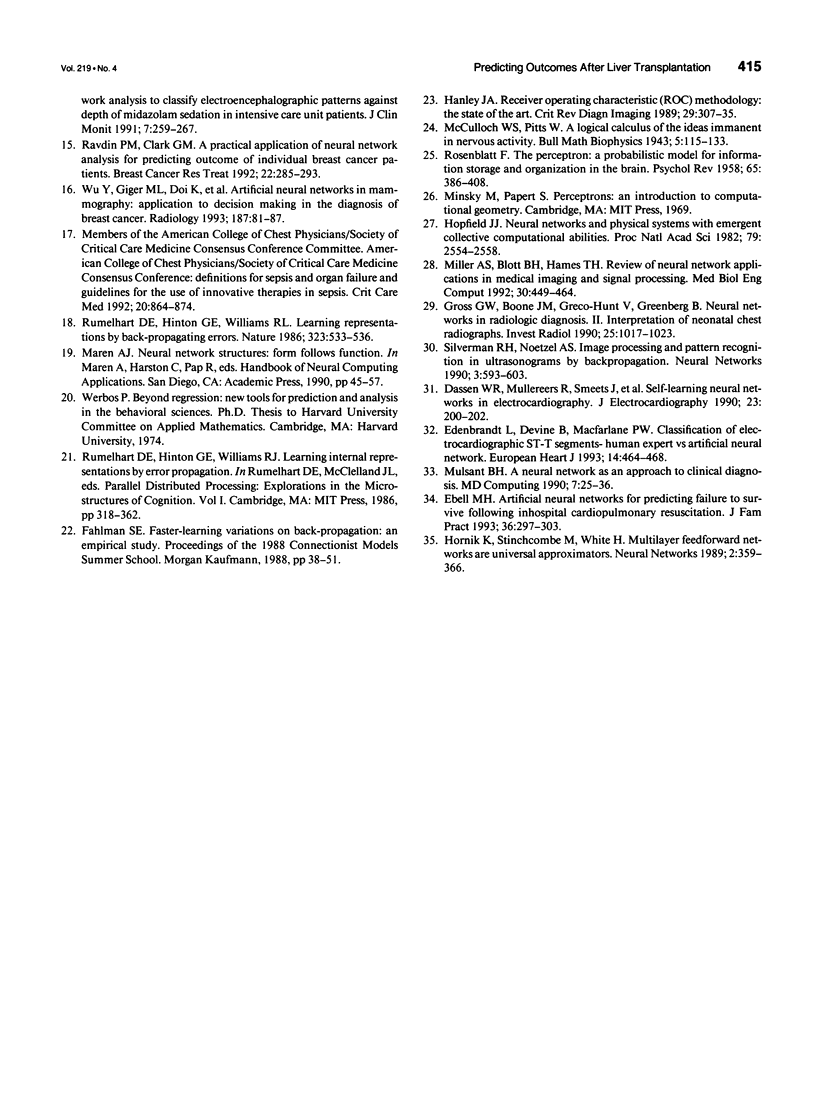
Selected References
These references are in PubMed. This may not be the complete list of references from this article.
- Baxt W. G. Use of an artificial neural network for the diagnosis of myocardial infarction. Ann Intern Med. 1991 Dec 1;115(11):843–848. doi: 10.7326/0003-4819-115-11-843. [DOI] [PubMed] [Google Scholar]
- Dassen W. R., Mulleneers R., Smeets J., den Dulk K., Cruz F., Brugada P., Wellens H. J. Self-learning neural networks in electrocardiography. J Electrocardiol. 1990;23 (Suppl):200–202. doi: 10.1016/0022-0736(90)90102-8. [DOI] [PubMed] [Google Scholar]
- Demeler B., Zhou G. W. Neural network optimization for E. coli promoter prediction. Nucleic Acids Res. 1991 Apr 11;19(7):1593–1599. doi: 10.1093/nar/19.7.1593. [DOI] [PMC free article] [PubMed] [Google Scholar]
- Ebell M. H. Artificial neural networks for predicting failure to survive following in-hospital cardiopulmonary resuscitation. J Fam Pract. 1993 Mar;36(3):297–303. [PubMed] [Google Scholar]
- Edenbrandt L., Devine B., Macfarlane P. W. Classification of electrocardiographic ST-T segments--human expert vs artificial neural network. Eur Heart J. 1993 Apr;14(4):464–468. doi: 10.1093/eurheartj/14.4.464. [DOI] [PubMed] [Google Scholar]
- Evans R. W., Manninen D. L., Dong F. B. An economic analysis of liver transplantation. Costs, insurance coverage, and reimbursement. Gastroenterol Clin North Am. 1993 Jun;22(2):451–473. [PubMed] [Google Scholar]
- Furlong J. W., Dupuy M. E., Heinsimer J. A. Neural network analysis of serial cardiac enzyme data. A clinical application of artificial machine intelligence. Am J Clin Pathol. 1991 Jul;96(1):134–141. doi: 10.1093/ajcp/96.1.134. [DOI] [PubMed] [Google Scholar]
- Gross G. W., Boone J. M., Greco-Hunt V., Greenberg B. Neural networks in radiologic diagnosis. II. Interpretation of neonatal chest radiographs. Invest Radiol. 1990 Sep;25(9):1017–1023. doi: 10.1097/00004424-199009000-00013. [DOI] [PubMed] [Google Scholar]
- Hanley J. A. Receiver operating characteristic (ROC) methodology: the state of the art. Crit Rev Diagn Imaging. 1989;29(3):307–335. [PubMed] [Google Scholar]
- Hopfield J. J. Neural networks and physical systems with emergent collective computational abilities. Proc Natl Acad Sci U S A. 1982 Apr;79(8):2554–2558. doi: 10.1073/pnas.79.8.2554. [DOI] [PMC free article] [PubMed] [Google Scholar]
- Miller A. S., Blott B. H., Hames T. K. Review of neural network applications in medical imaging and signal processing. Med Biol Eng Comput. 1992 Sep;30(5):449–464. doi: 10.1007/BF02457822. [DOI] [PubMed] [Google Scholar]
- Mulsant B. H. A neural network as an approach to clinical diagnosis. MD Comput. 1990 Jan-Feb;7(1):25–36. [PubMed] [Google Scholar]
- ROSENBLATT F. The perceptron: a probabilistic model for information storage and organization in the brain. Psychol Rev. 1958 Nov;65(6):386–408. doi: 10.1037/h0042519. [DOI] [PubMed] [Google Scholar]
- Ravdin P. M., Clark G. M. A practical application of neural network analysis for predicting outcome of individual breast cancer patients. Breast Cancer Res Treat. 1992;22(3):285–293. doi: 10.1007/BF01840841. [DOI] [PubMed] [Google Scholar]
- Veselis R. A., Reinsel R., Sommer S., Carlon G. Use of neural network analysis to classify electroencephalographic patterns against depth of midazolam sedation in intensive care unit patients. J Clin Monit. 1991 Jul;7(3):259–267. doi: 10.1007/BF01619271. [DOI] [PubMed] [Google Scholar]
- Wu Y., Giger M. L., Doi K., Vyborny C. J., Schmidt R. A., Metz C. E. Artificial neural networks in mammography: application to decision making in the diagnosis of breast cancer. Radiology. 1993 Apr;187(1):81–87. doi: 10.1148/radiology.187.1.8451441. [DOI] [PubMed] [Google Scholar]