Abstract
Prostate cancer is the second most common malignancy in men in the UK. The disease is unpredictable in its behaviour and, at present, no single investigative method allows clinicians to differentiate between tumours that will progress and those that will remain quiescent. There is an increasing need for novel means to predict prognosis and outcome of the disease. The aim of this study was to assess the value of artificial neural networks in predicting outcome in prostate cancer in comparison with statistical methods, using a combination of conventional and experimental biological markers. Forty-one patients with different stages and grades of prostate cancer undergoing a variety of treatments were analysed. Artificial neural networks were used as follows: eight input neurons consisting of six conventional factors (age, stage, bone scan findings, grade, serum PSA, treatment) and two experimental markers (immunostaining for bcl-2 and p53, which are both apoptosis-regulating genes). Twenty-one patients were used for training and 20 for testing. A total of 80% of the patients were correctly classified regarding outcome using the combination of factors. When both bcl-2 and p53 immunoreactivity were excluded from the analysis, correct prediction of the outcome was achieved in only 60% of the patients (P = 0.0032). This study was able to demonstrate the value of artificial neural networks in the analysis of prognostic markers in prostate cancer. In addition, the potential for using this technology to evaluate novel markers is highlighted. Further large-scale analyses are required to incorporate this methodology into routine clinical practice.
Full text
PDF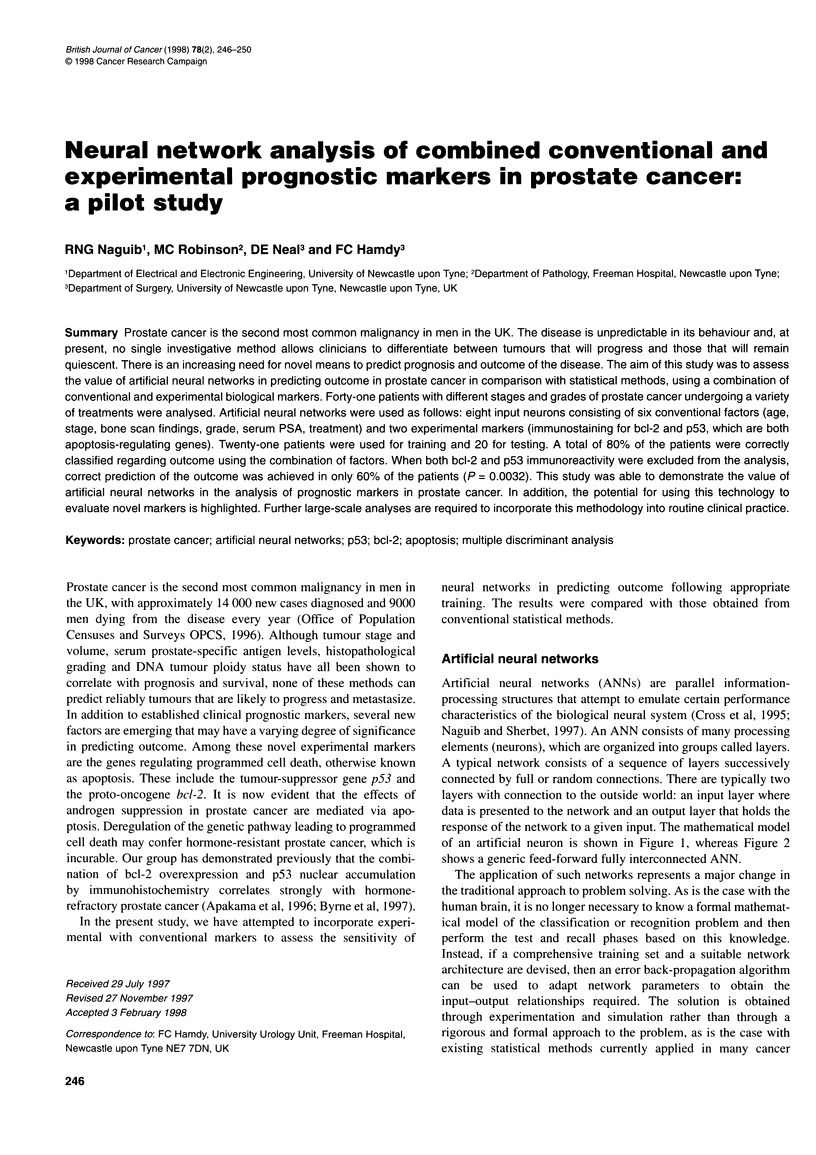
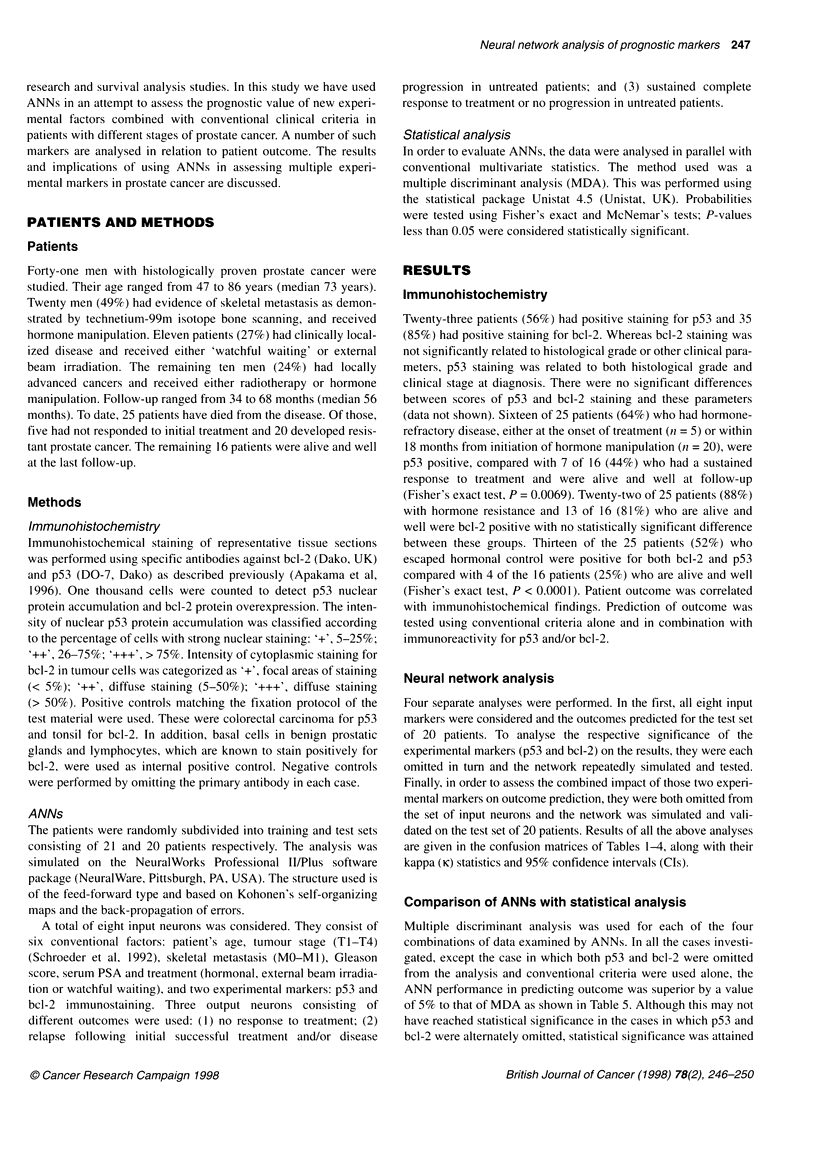
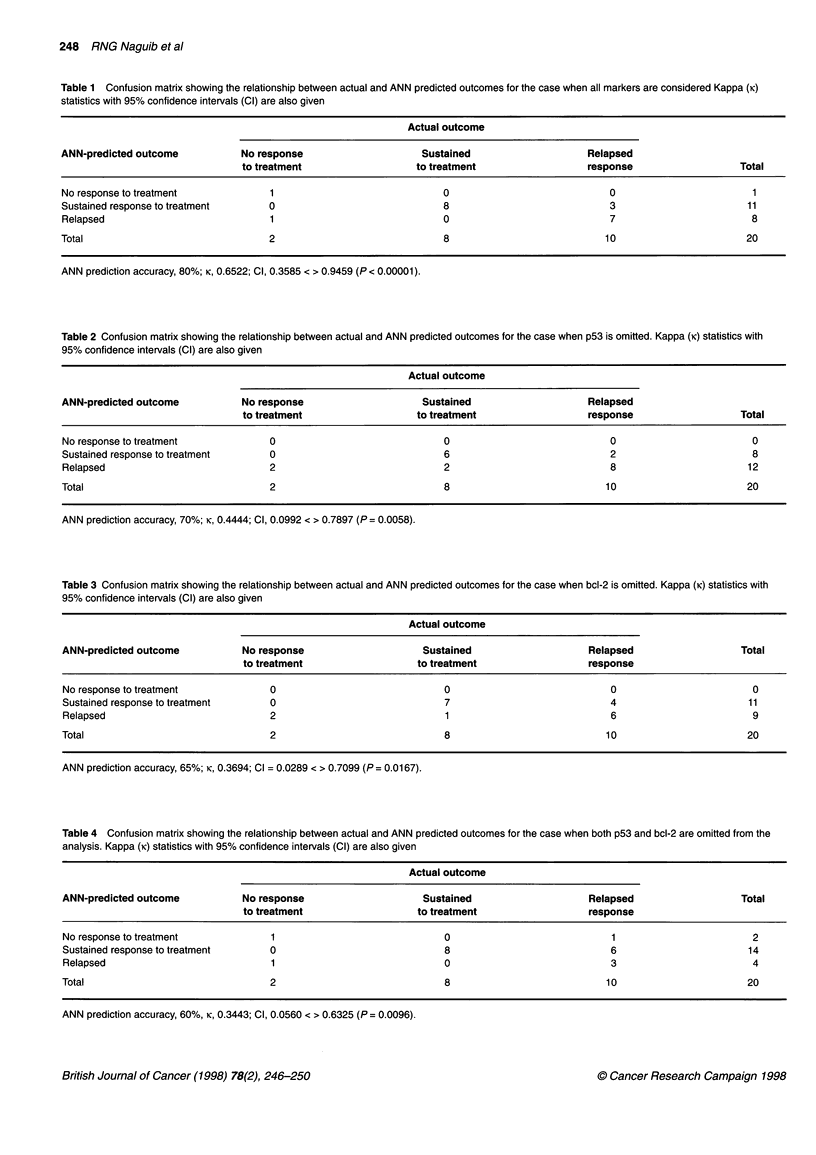
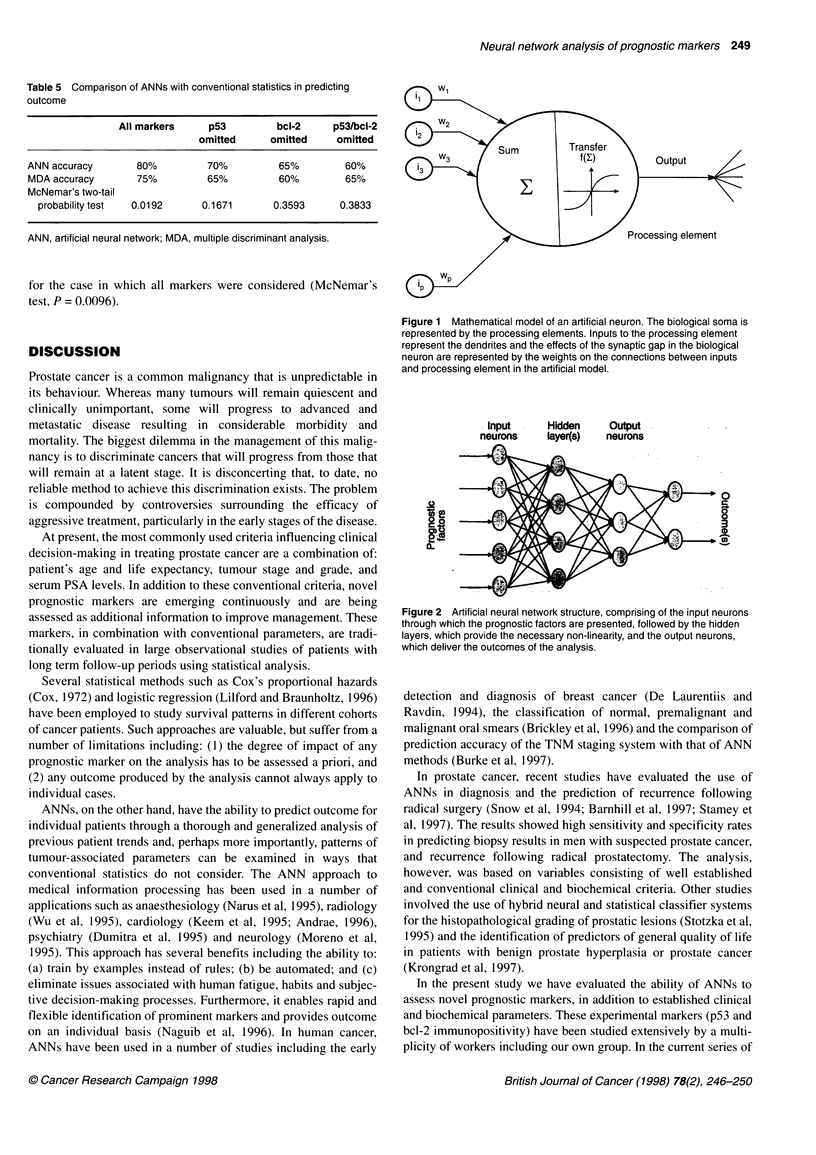
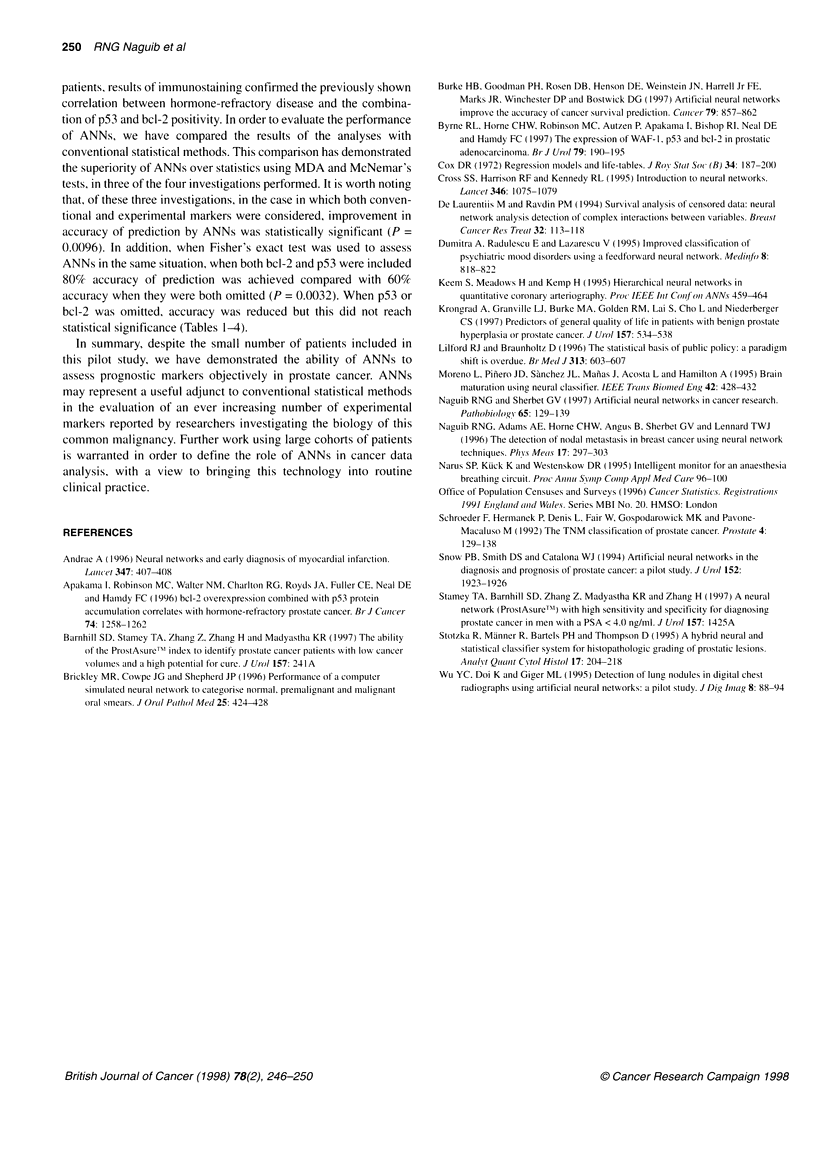
Images in this article
Selected References
These references are in PubMed. This may not be the complete list of references from this article.
- Apakama I., Robinson M. C., Walter N. M., Charlton R. G., Royds J. A., Fuller C. E., Neal D. E., Hamdy F. C. bcl-2 overexpression combined with p53 protein accumulation correlates with hormone-refractory prostate cancer. Br J Cancer. 1996 Oct;74(8):1258–1262. doi: 10.1038/bjc.1996.526. [DOI] [PMC free article] [PubMed] [Google Scholar]
- Burke H. B., Goodman P. H., Rosen D. B., Henson D. E., Weinstein J. N., Harrell F. E., Jr, Marks J. R., Winchester D. P., Bostwick D. G. Artificial neural networks improve the accuracy of cancer survival prediction. Cancer. 1997 Feb 15;79(4):857–862. doi: 10.1002/(sici)1097-0142(19970215)79:4<857::aid-cncr24>3.0.co;2-y. [DOI] [PubMed] [Google Scholar]
- Byrne R. L., Horne C. H., Robinson M. C., Autzen P., Apakama I., Bishop R. I., Neal D. E., Hamdy F. C. The expression of waf-1, p53 and bcl-2 in prostatic adenocarcinoma. Br J Urol. 1997 Feb;79(2):190–195. doi: 10.1046/j.1464-410x.1997.03399.x. [DOI] [PubMed] [Google Scholar]
- Cross S. S., Harrison R. F., Kennedy R. L. Introduction to neural networks. Lancet. 1995 Oct 21;346(8982):1075–1079. doi: 10.1016/s0140-6736(95)91746-2. [DOI] [PubMed] [Google Scholar]
- De Laurentiis M., Ravdin P. M. Survival analysis of censored data: neural network analysis detection of complex interactions between variables. Breast Cancer Res Treat. 1994;32(1):113–118. doi: 10.1007/BF00666212. [DOI] [PubMed] [Google Scholar]
- Dumitra A., Radulescu E., Lazarescu V. Improved classification of psychiatric mood disorders using a feedforward neural network. Medinfo. 1995;8(Pt 1):818–822. [PubMed] [Google Scholar]
- Krongrad A., Granville L. J., Burke M. A., Golden R. M., Lai S., Cho L., Niederberger C. S. Predictors of general quality of life in patients with benign prostate hyperplasia or prostate cancer. J Urol. 1997 Feb;157(2):534–538. [PubMed] [Google Scholar]
- Lilford R. J., Braunholtz D. The statistical basis of public policy: a paradigm shift is overdue. BMJ. 1996 Sep 7;313(7057):603–607. doi: 10.1136/bmj.313.7057.603. [DOI] [PMC free article] [PubMed] [Google Scholar]
- Moreno L., Piñeiro J. D., Sánchez J. L., Mañas S., Merino J., Acosta L., Hamilton A. Brain maturation estimation using neural classifier. IEEE Trans Biomed Eng. 1995 Apr;42(4):428–432. doi: 10.1109/10.376139. [DOI] [PubMed] [Google Scholar]
- Naguib R. N., Adams A. E., Horne C. H., Angus B., Sherbet G. V., Lennard T. W. The detection of nodal metastasis in breast cancer using neural network techniques. Physiol Meas. 1996 Nov;17(4):297–303. doi: 10.1088/0967-3334/17/4/007. [DOI] [PubMed] [Google Scholar]
- Naguib R. N., Sherbet G. V. Artificial neural networks in cancer research. Pathobiology. 1997;65(3):129–139. doi: 10.1159/000164114. [DOI] [PubMed] [Google Scholar]
- Narus S. P., Kück K., Westenskow D. R. Intelligent monitor for an anesthesia breathing circuit. Proc Annu Symp Comput Appl Med Care. 1995:96–100. [PMC free article] [PubMed] [Google Scholar]
- Schröder F. H., Hermanek P., Denis L., Fair W. R., Gospodarowicz M. K., Pavone-Macaluso M. The TNM classification of prostate cancer. Prostate Suppl. 1992;4:129–138. doi: 10.1002/pros.2990210521. [DOI] [PubMed] [Google Scholar]
- Snow P. B., Smith D. S., Catalona W. J. Artificial neural networks in the diagnosis and prognosis of prostate cancer: a pilot study. J Urol. 1994 Nov;152(5 Pt 2):1923–1926. doi: 10.1016/s0022-5347(17)32416-3. [DOI] [PubMed] [Google Scholar]
- Stotzka R., Männer R., Bartels P. H., Thompson D. A hybrid neural and statistical classifier system for histopathologic grading of prostatic lesions. Anal Quant Cytol Histol. 1995 Jun;17(3):204–218. [PubMed] [Google Scholar]
- Wu Y. C., Doi K., Giger M. L. Detection of lung nodules in digital chest radiographs using artificial neural networks: a pilot study. J Digit Imaging. 1995 May;8(2):88–94. doi: 10.1007/BF03168131. [DOI] [PubMed] [Google Scholar]