Abstract
Actual use of regression models in clinical practice depends on model simplicity. Reducing the number of variables in a model contributes to this goal. The quality of a particular selection of variables for a logistic regression model can be defined in terms of the number of variables selected and the model's discriminatory performance, as measured by the area under the ROC curve. A genetic algorithm was applied to search for the best variable combinations for modeling presence of myocardial infarction in a data set of patients with chest pain. Using an external validation set, the resulting model was compared with models constructed with standard backward, forward and stepwise methods of variable selection. The improvement in discriminatory ability yielded by the genetic algorithm variable selection method was statistically significant (p < 0.02).
Full text
PDF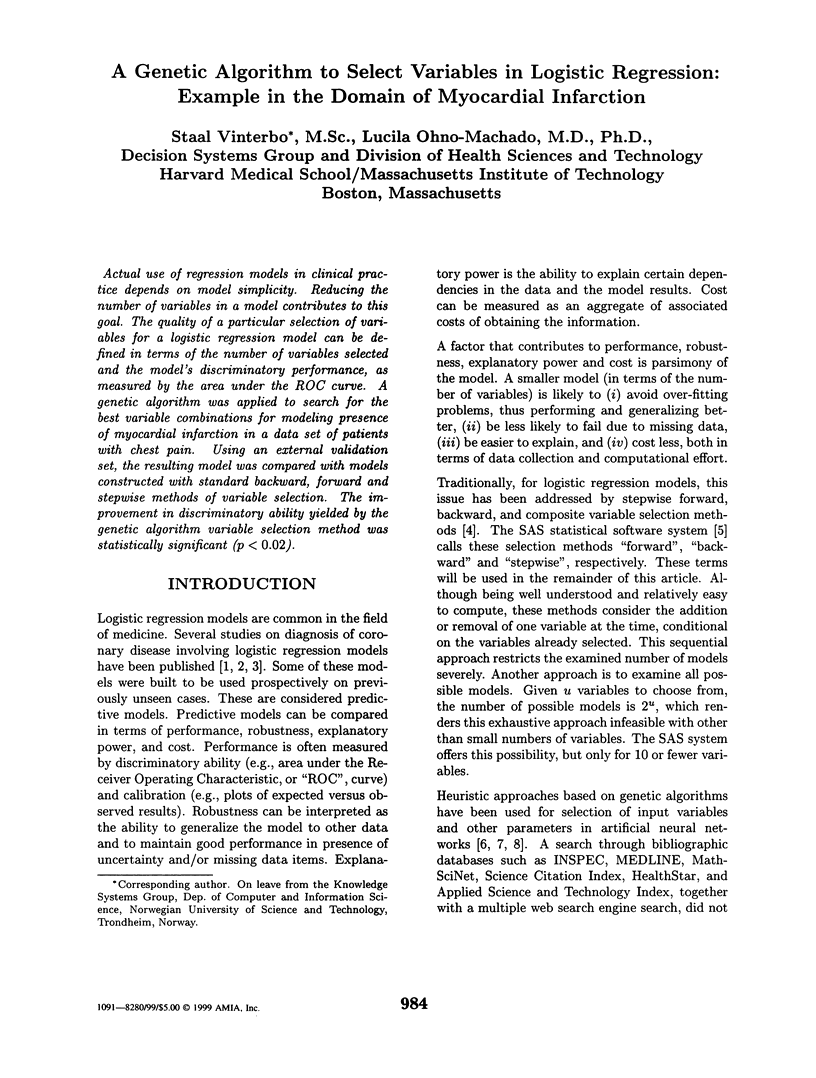
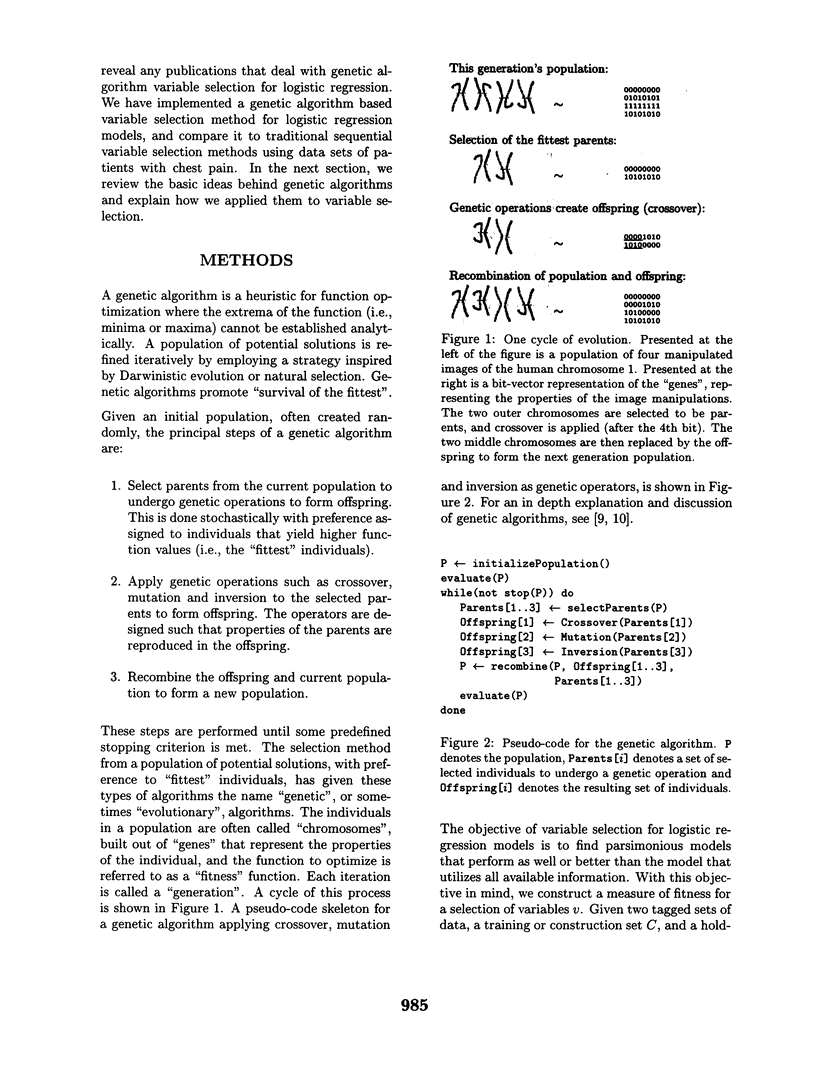
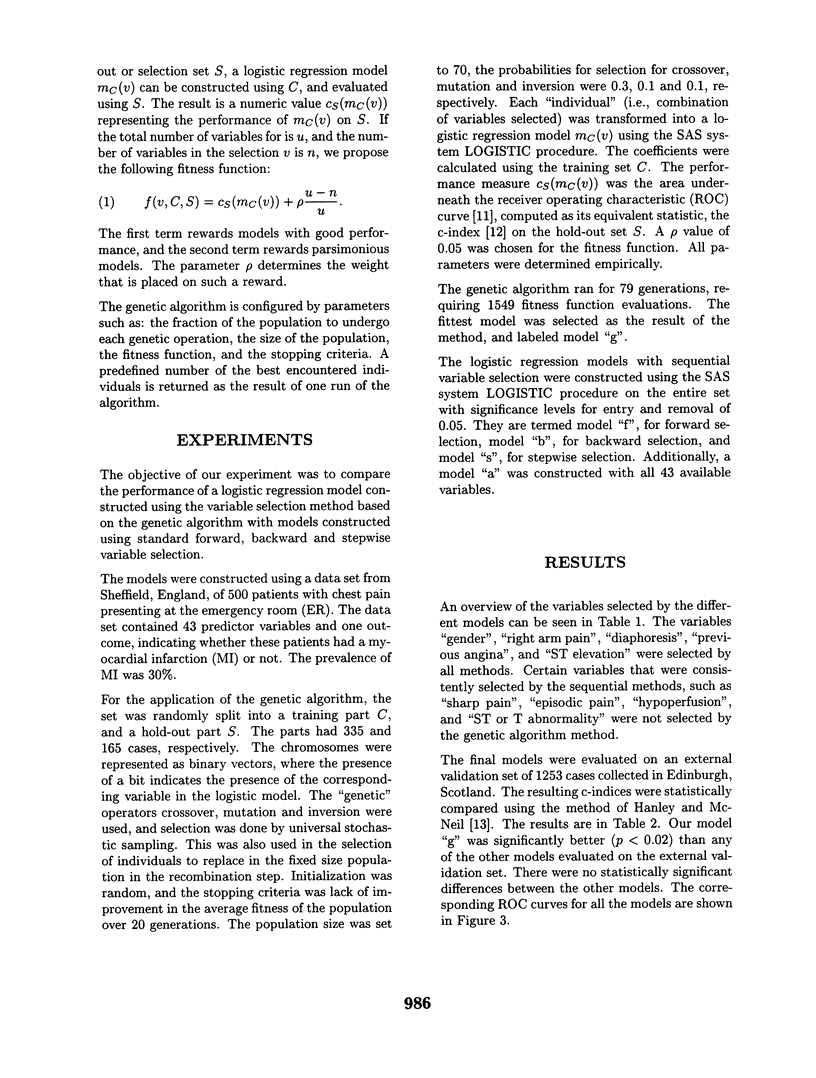
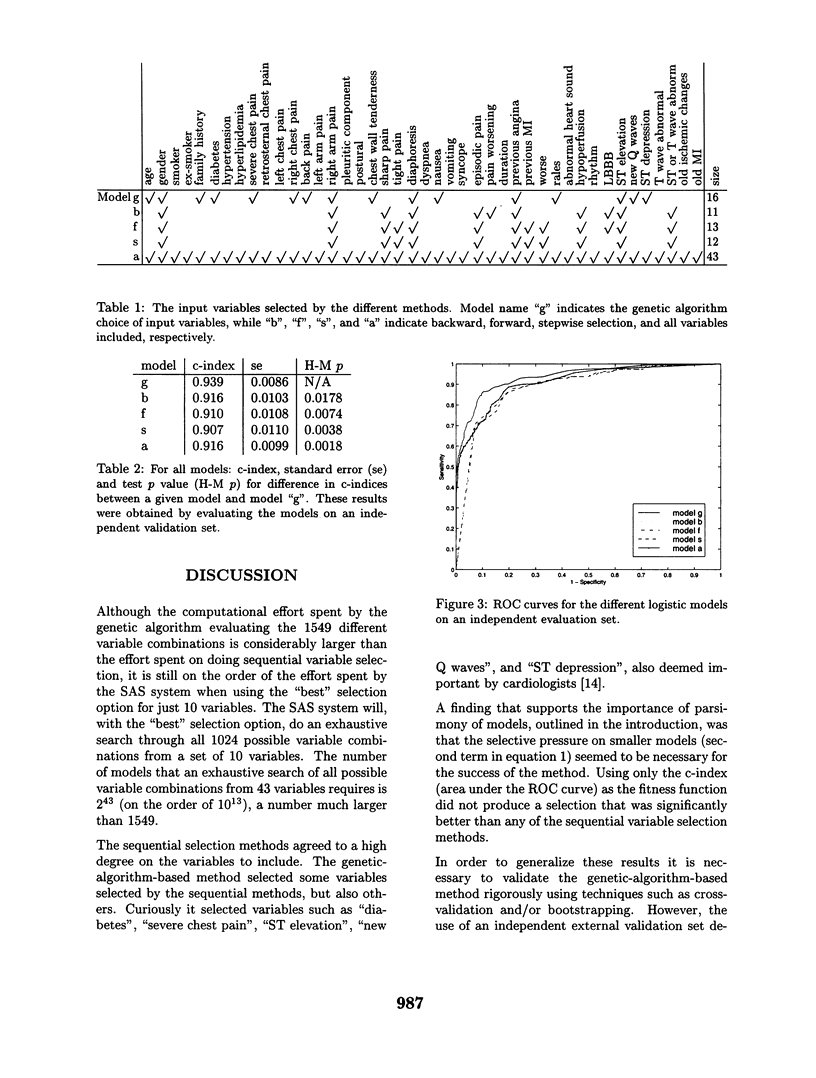
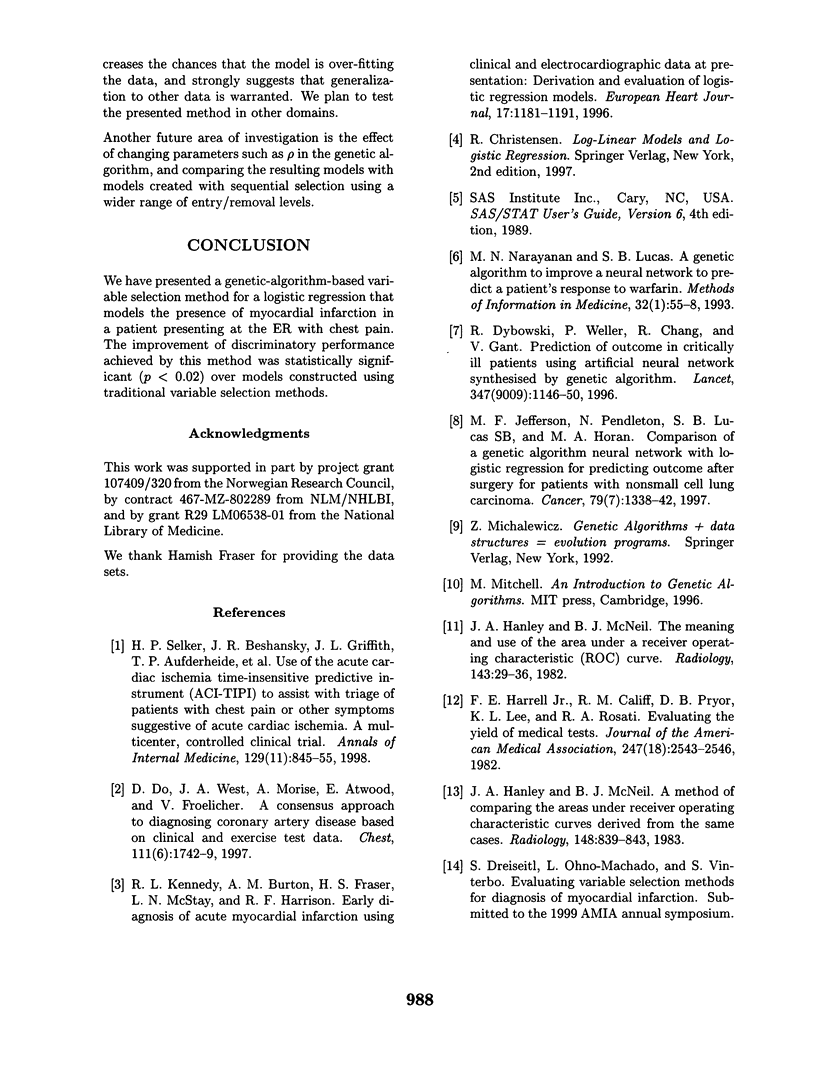
Selected References
These references are in PubMed. This may not be the complete list of references from this article.
- Do D., West J. A., Morise A., Atwood E., Froelicher V. A consensus approach to diagnosing coronary artery disease based on clinical and exercise test data. Chest. 1997 Jun;111(6):1742–1749. doi: 10.1378/chest.111.6.1742. [DOI] [PubMed] [Google Scholar]
- Dybowski R., Weller P., Chang R., Gant V. Prediction of outcome in critically ill patients using artificial neural network synthesised by genetic algorithm. Lancet. 1996 Apr 27;347(9009):1146–1150. doi: 10.1016/s0140-6736(96)90609-1. [DOI] [PubMed] [Google Scholar]
- Hanley J. A., McNeil B. J. A method of comparing the areas under receiver operating characteristic curves derived from the same cases. Radiology. 1983 Sep;148(3):839–843. doi: 10.1148/radiology.148.3.6878708. [DOI] [PubMed] [Google Scholar]
- Hanley J. A., McNeil B. J. The meaning and use of the area under a receiver operating characteristic (ROC) curve. Radiology. 1982 Apr;143(1):29–36. doi: 10.1148/radiology.143.1.7063747. [DOI] [PubMed] [Google Scholar]
- Harrell F. E., Jr, Califf R. M., Pryor D. B., Lee K. L., Rosati R. A. Evaluating the yield of medical tests. JAMA. 1982 May 14;247(18):2543–2546. [PubMed] [Google Scholar]
- Jefferson M. F., Pendleton N., Lucas S. B., Horan M. A. Comparison of a genetic algorithm neural network with logistic regression for predicting outcome after surgery for patients with nonsmall cell lung carcinoma. Cancer. 1997 Apr 1;79(7):1338–1342. doi: 10.1002/(sici)1097-0142(19970401)79:7<1338::aid-cncr10>3.0.co;2-0. [DOI] [PubMed] [Google Scholar]
- Kennedy R. L., Burton A. M., Fraser H. S., McStay L. N., Harrison R. F. Early diagnosis of acute myocardial infarction using clinical and electrocardiographic data at presentation: derivation and evaluation of logistic regression models. Eur Heart J. 1996 Aug;17(8):1181–1191. doi: 10.1093/oxfordjournals.eurheartj.a015035. [DOI] [PubMed] [Google Scholar]