Abstract
At our institution, a Natural Language Processing (NLP) tool called MedLEE is used on a daily basis to parse medical texts including complete discharge summaries. MedLEE transforms written text into a generic structured format, which preserves the richness of the underlying natural language expressions by the use of concept modifiers (like change, certainty, degree and status). As a tradeoff, extraction of application-specific medical information is difficult without a clear understanding of how these modifiers combine. We report on a knowledge model for MedLEE modifiers that is helpful for a high level interpretation of NLP data and is used for the generation of two distinct views on NLP-parsed discharge summaries: A physician view offering a condensed overview of the severity of patient problems and a data mining view featuring binary problem states useful for machine learning.
Full text
PDF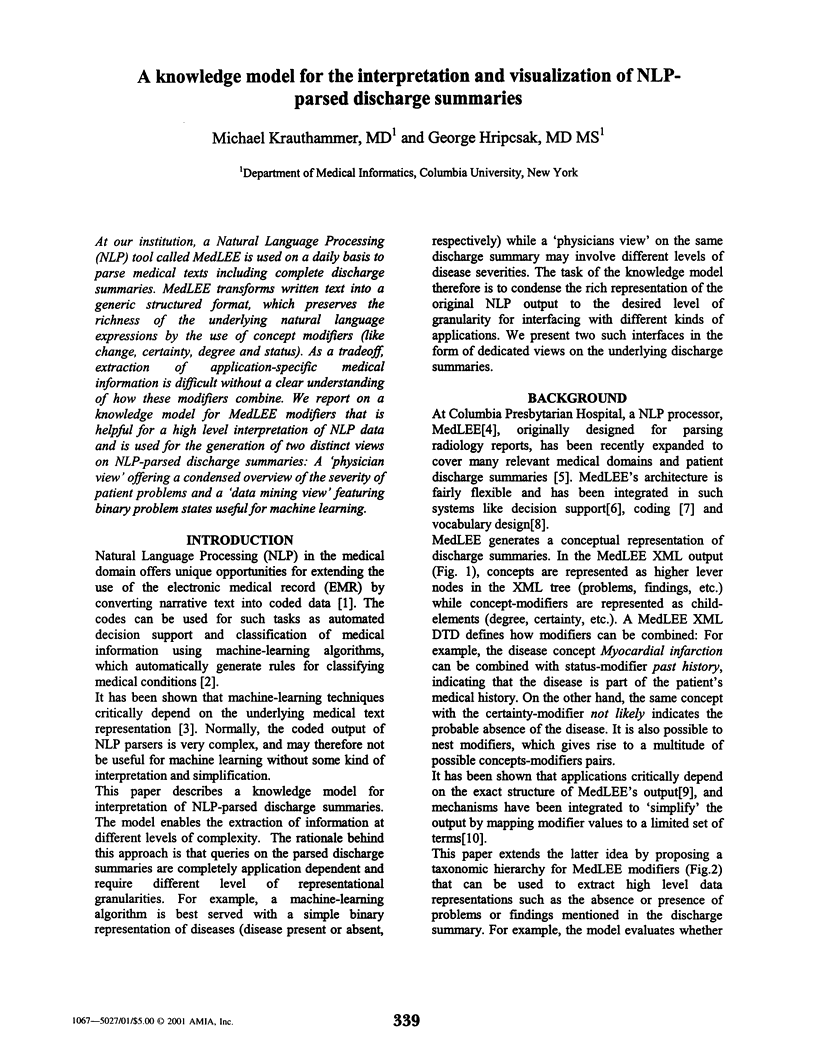
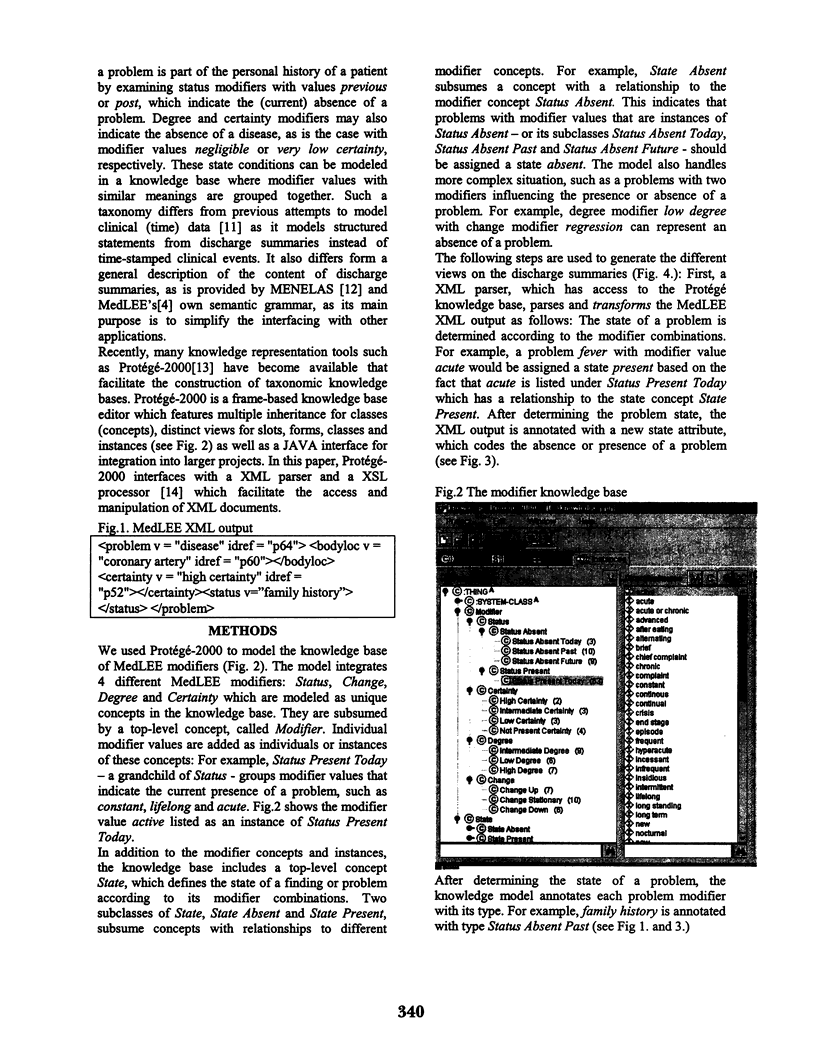
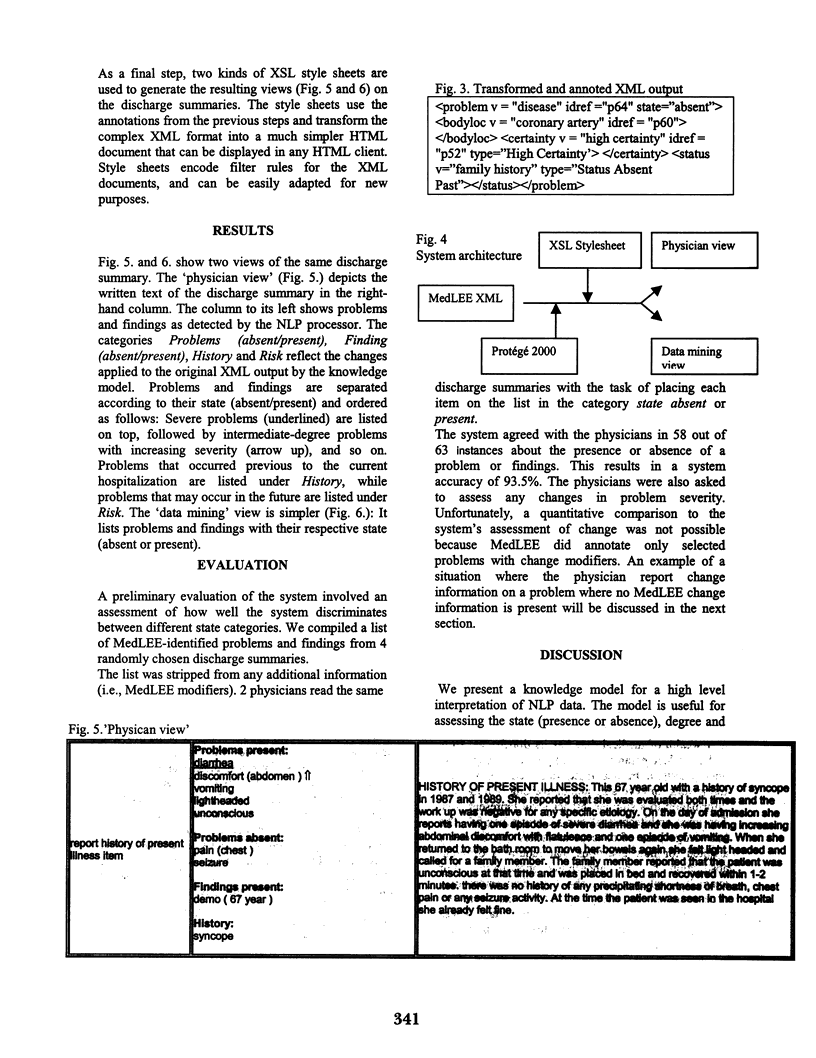
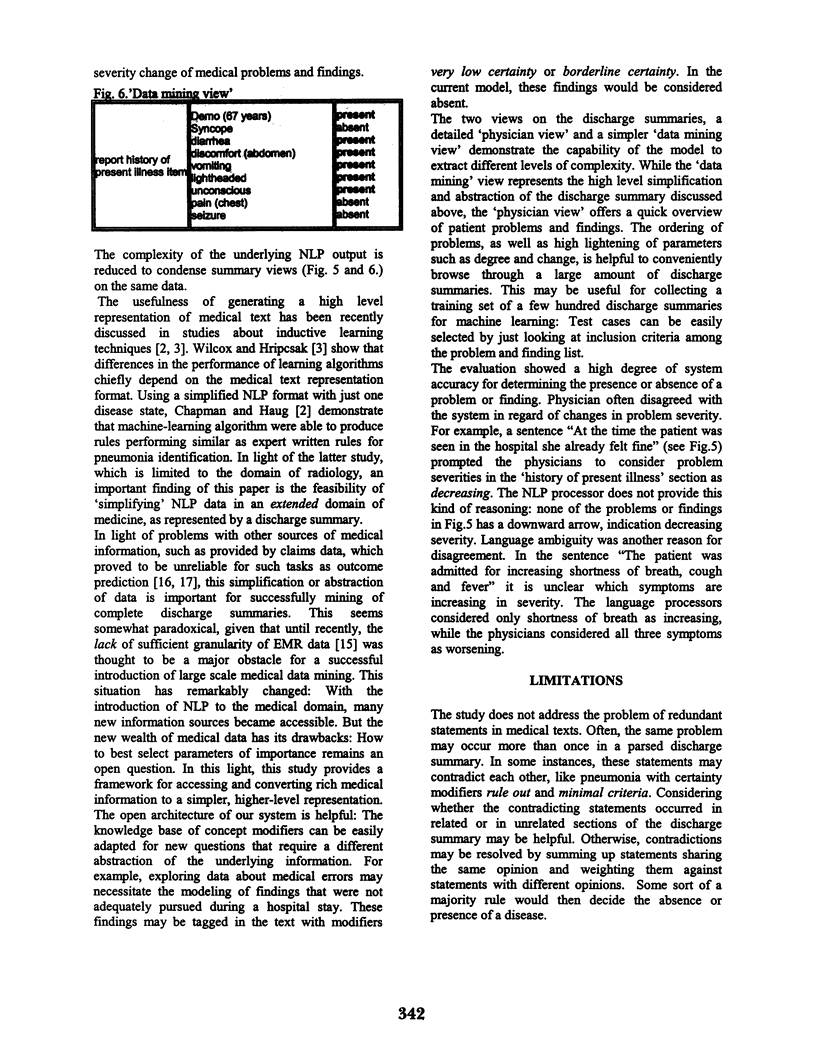
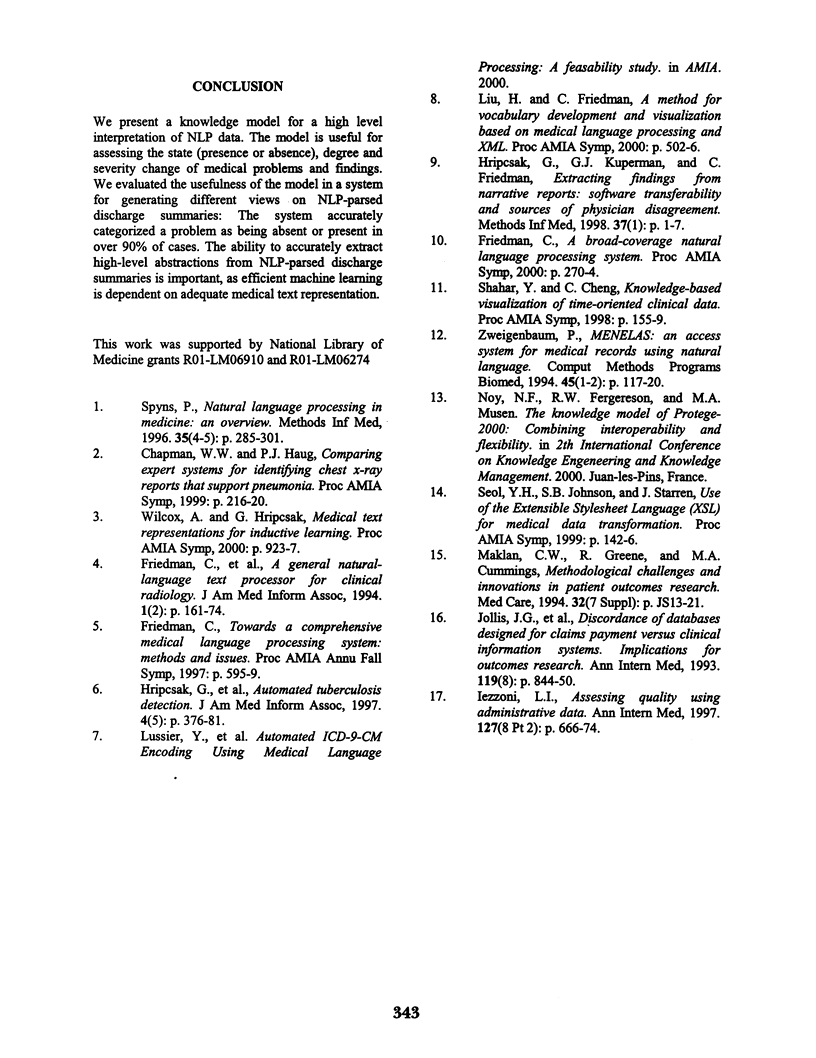
Selected References
These references are in PubMed. This may not be the complete list of references from this article.
- Chapman W. W., Haug P. J. Comparing expert systems for identifying chest x-ray reports that support pneumonia. Proc AMIA Symp. 1999:216–220. [PMC free article] [PubMed] [Google Scholar]
- Friedman C. A broad-coverage natural language processing system. Proc AMIA Symp. 2000:270–274. [PMC free article] [PubMed] [Google Scholar]
- Friedman C., Alderson P. O., Austin J. H., Cimino J. J., Johnson S. B. A general natural-language text processor for clinical radiology. J Am Med Inform Assoc. 1994 Mar-Apr;1(2):161–174. doi: 10.1136/jamia.1994.95236146. [DOI] [PMC free article] [PubMed] [Google Scholar]
- Friedman C. Towards a comprehensive medical language processing system: methods and issues. Proc AMIA Annu Fall Symp. 1997:595–599. [PMC free article] [PubMed] [Google Scholar]
- Hripcsak G., Knirsch C. A., Jain N. L., Pablos-Mendez A. Automated tuberculosis detection. J Am Med Inform Assoc. 1997 Sep-Oct;4(5):376–381. doi: 10.1136/jamia.1997.0040376. [DOI] [PMC free article] [PubMed] [Google Scholar]
- Jollis J. G., Ancukiewicz M., DeLong E. R., Pryor D. B., Muhlbaier L. H., Mark D. B. Discordance of databases designed for claims payment versus clinical information systems. Implications for outcomes research. Ann Intern Med. 1993 Oct 15;119(8):844–850. doi: 10.7326/0003-4819-119-8-199310150-00011. [DOI] [PubMed] [Google Scholar]
- Liu H., Friedman C. A method for vocabulary development and visualization based on medical language processing and XML. Proc AMIA Symp. 2000:502–506. [PMC free article] [PubMed] [Google Scholar]
- Seol Y. H., Johnson S. B., Starren J. Use of the Extensible Stylesheet Language (XSL) for medical data transformation. Proc AMIA Symp. 1999:142–146. [PMC free article] [PubMed] [Google Scholar]
- Shahar Y., Cheng C. Knowledge-based visualization of time-oriented clinical data. Proc AMIA Symp. 1998:155–159. [PMC free article] [PubMed] [Google Scholar]
- Spyns P. Natural language processing in medicine: an overview. Methods Inf Med. 1996 Dec;35(4-5):285–301. [PubMed] [Google Scholar]
- Wilcox A., Hripcsak G. Medical text representations for inductive learning. Proc AMIA Symp. 2000:923–927. [PMC free article] [PubMed] [Google Scholar]
- Zweigenbaum P. MENELAS: an access system for medical records using natural language. Comput Methods Programs Biomed. 1994 Oct;45(1-2):117–120. doi: 10.1016/0169-2607(94)90029-9. [DOI] [PubMed] [Google Scholar]