Abstract
A methodology based on the fuzzy set theory and the convolution neural network (CNN) architecture is proposed to tackle the problem of reducing false-positive rate in automatic lung nodule detection. The CNN which simulates human visual mechanism was trained by a supervised back-propagation algorithm based on fuzzy membership functions. The training and testing database consists of image blocks (each 32 x 32 pixels) of suspected lung nodule areas (nodule candidates) which were generated from our pre-scanning program [1]. A linguistic label was assigned to each nodule candidate of the training set, then the label was converted to a membership value through a pre-defined membership function and used as teaching signal (desired outputs) during the network learning. Before the nodule candidate was fed to the network input, it was pre-processed to reduce the complex background noise and the contrast discrepancy resulted from film development. During the network testing phase, a defuzzification process was applied to decipher the trained network's output triggered by the nodule candidate in the testing set. Finally, a Receiver Operating Characteristic (ROC) analysis was used to evaluate the CNN's performance based on the defuzzified output of the testing database. Preliminary results showed an average Az (the performance index) of 0.84 which is equivalent to 0.80 true-positive detection (sensitivity) with an average 2-3 false-positive detections per chest image.
Full text
PDF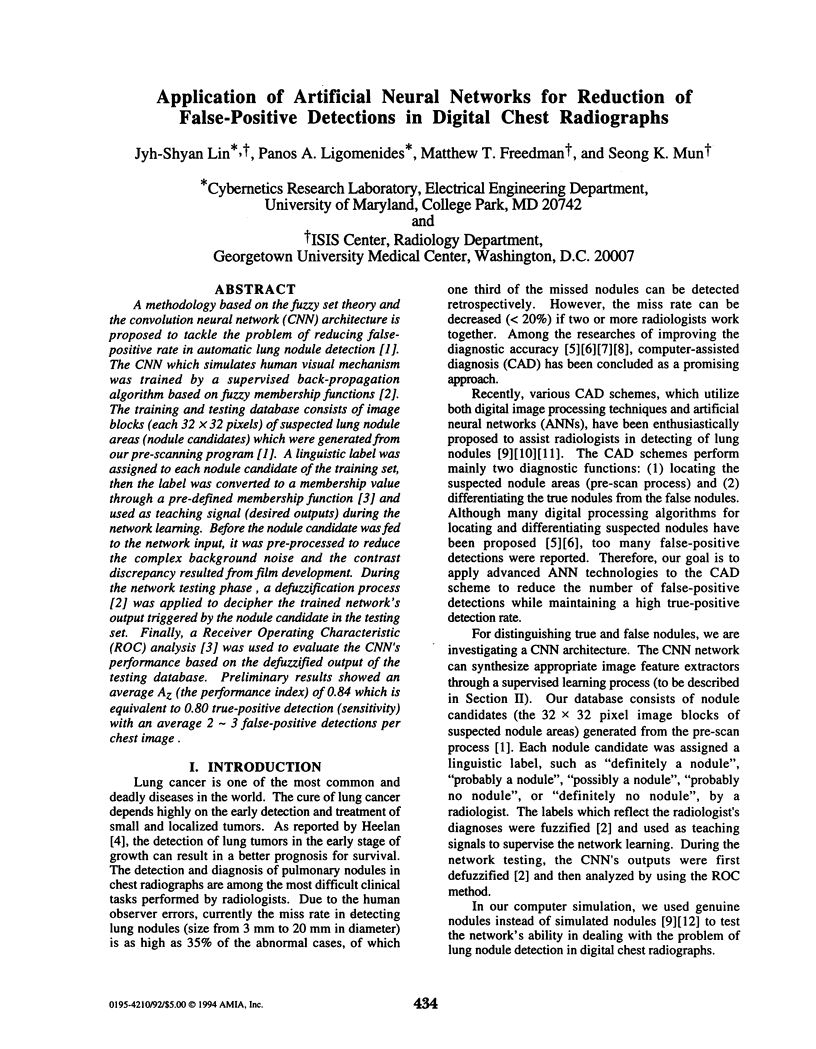
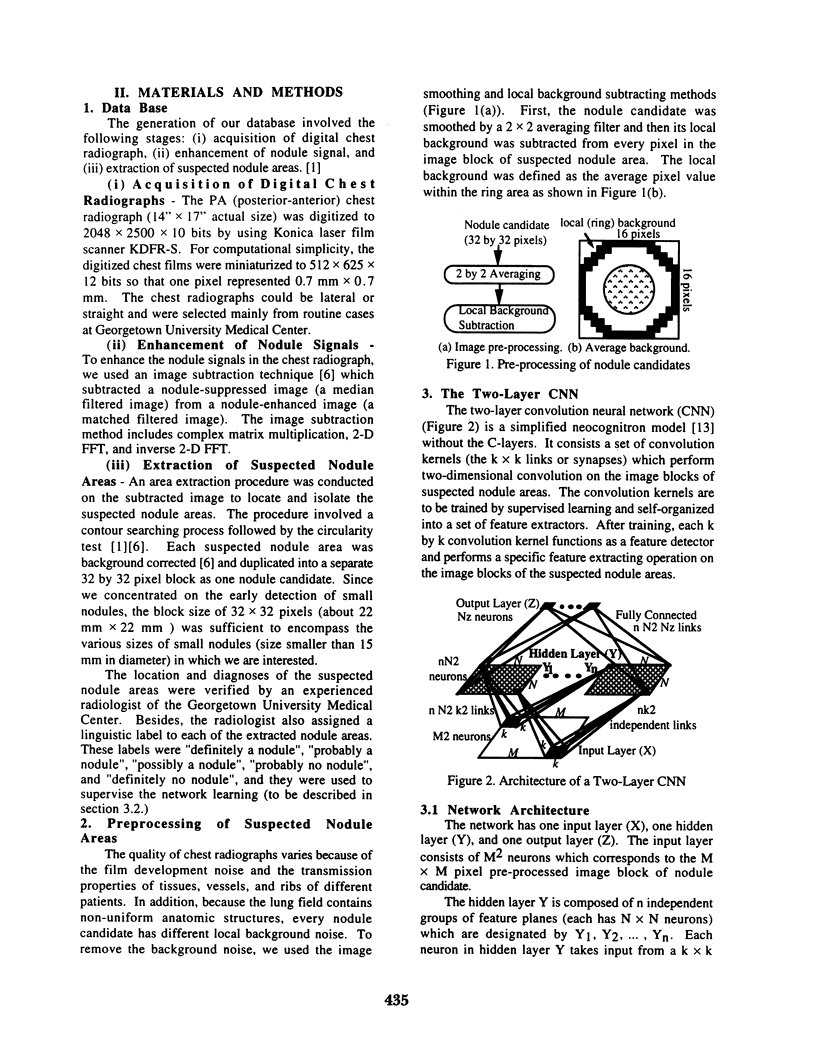
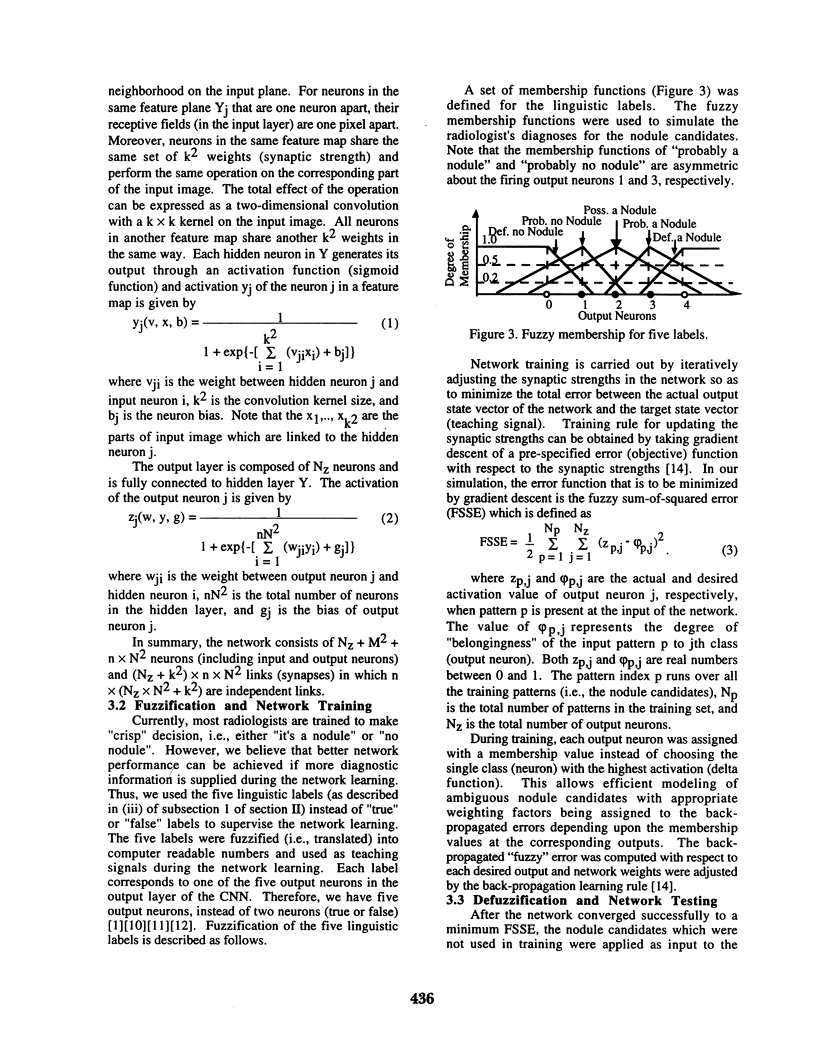
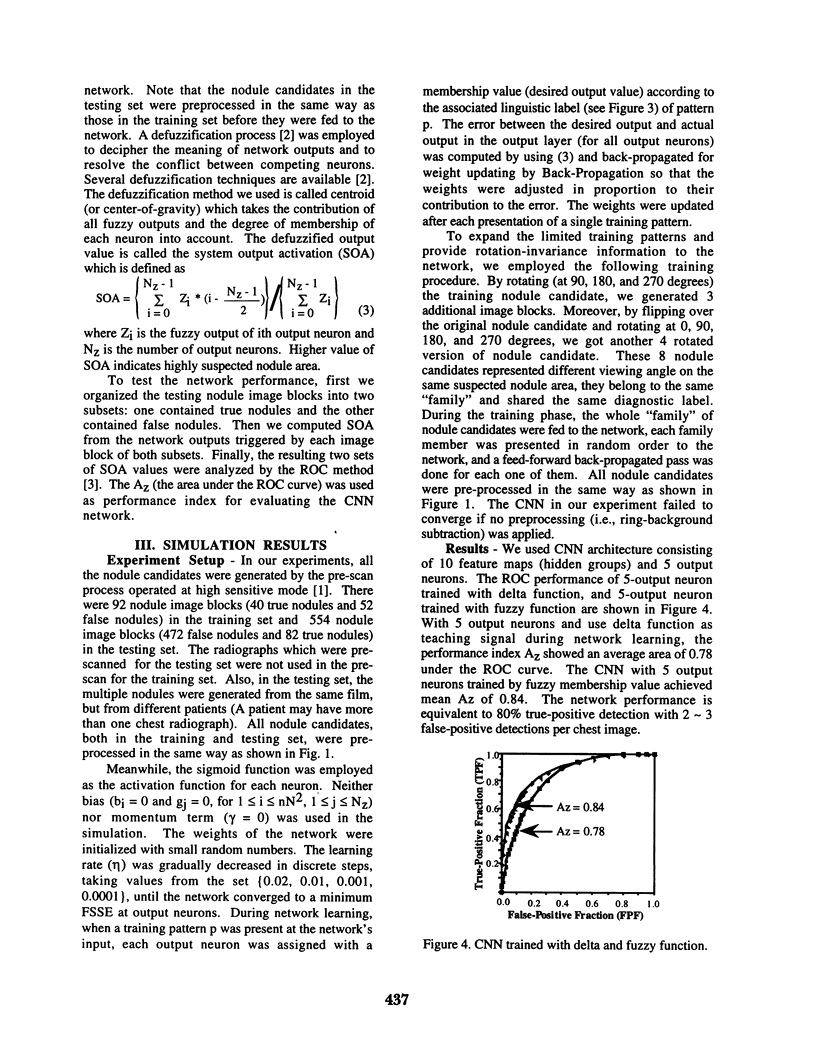
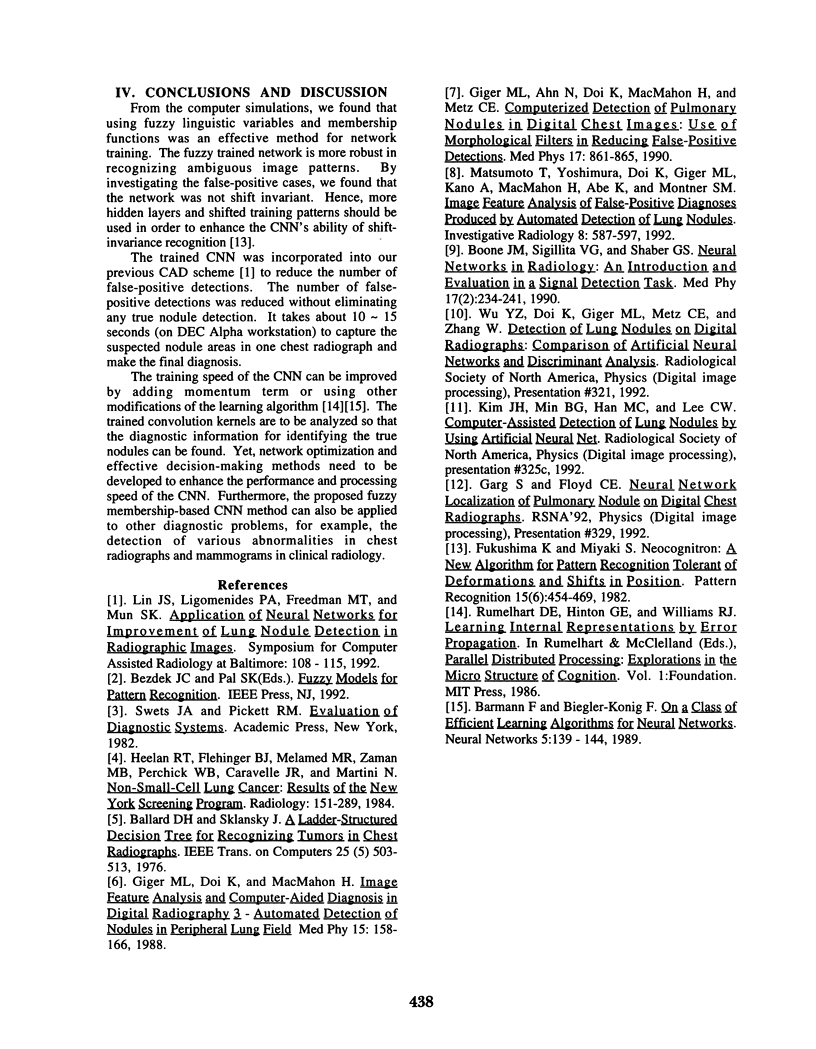
Selected References
These references are in PubMed. This may not be the complete list of references from this article.
- Boone J. M., Sigillito V. G., Shaber G. S. Neural networks in radiology: an introduction and evaluation in a signal detection task. Med Phys. 1990 Mar-Apr;17(2):234–241. doi: 10.1118/1.596501. [DOI] [PubMed] [Google Scholar]
- Giger M. L., Ahn N., Doi K., MacMahon H., Metz C. E. Computerized detection of pulmonary nodules in digital chest images: use of morphological filters in reducing false-positive detections. Med Phys. 1990 Sep-Oct;17(5):861–865. doi: 10.1118/1.596478. [DOI] [PubMed] [Google Scholar]
- Giger M. L., Doi K., MacMahon H. Image feature analysis and computer-aided diagnosis in digital radiography. 3. Automated detection of nodules in peripheral lung fields. Med Phys. 1988 Mar-Apr;15(2):158–166. doi: 10.1118/1.596247. [DOI] [PubMed] [Google Scholar]
- Heelan R. T., Flehinger B. J., Melamed M. R., Zaman M. B., Perchick W. B., Caravelli J. F., Martini N. Non-small-cell lung cancer: results of the New York screening program. Radiology. 1984 May;151(2):289–293. doi: 10.1148/radiology.151.2.6324279. [DOI] [PubMed] [Google Scholar]