Abstract
Learning algorithms have been used both on feed-forward deterministic networks and on feed-back statistical networks to capture input-output relations and do pattern classification. These learning algorithms are examined for a class of problems characterized by noisy or statistical data, in which the networks learn the relation between input data and probability distributions of answers. In simple but nontrivial networks the two learning rules are closely related. Under some circumstances the learning problem for the statistical networks can be solved without Monte Carlo procedures. The usual arbitrary learning goals of feed-forward networks can be given useful probabilistic meaning.
Full text
PDF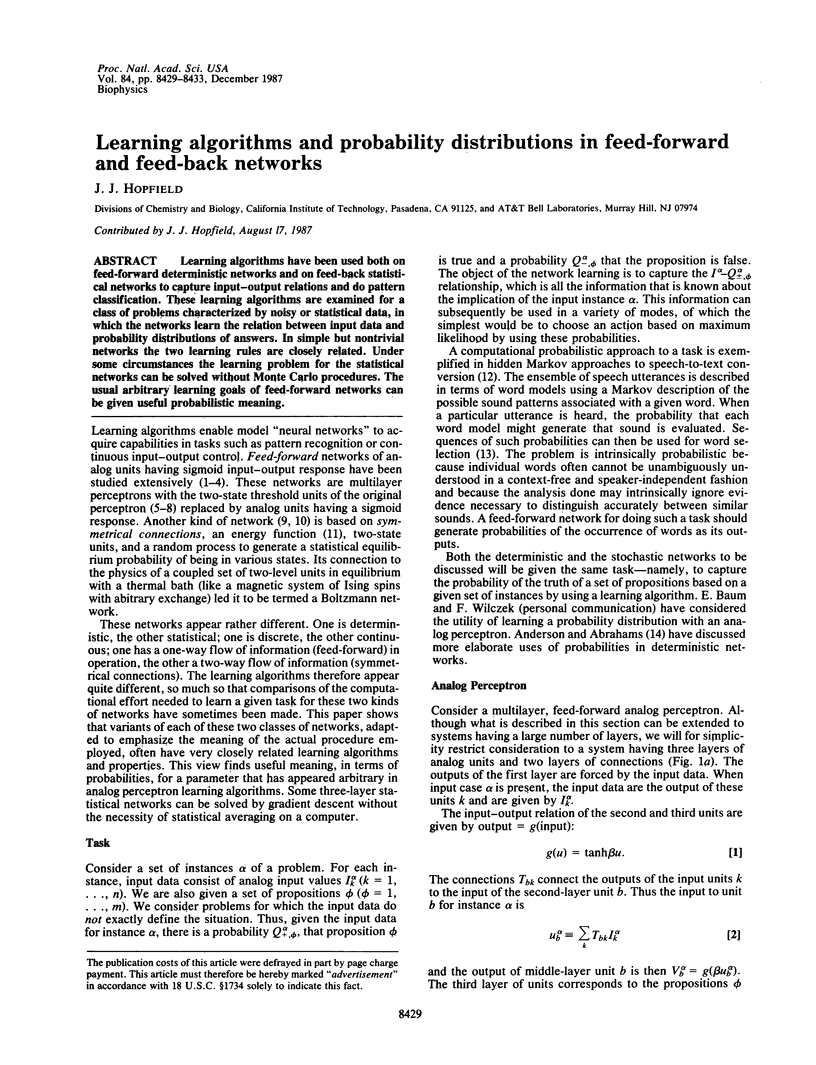
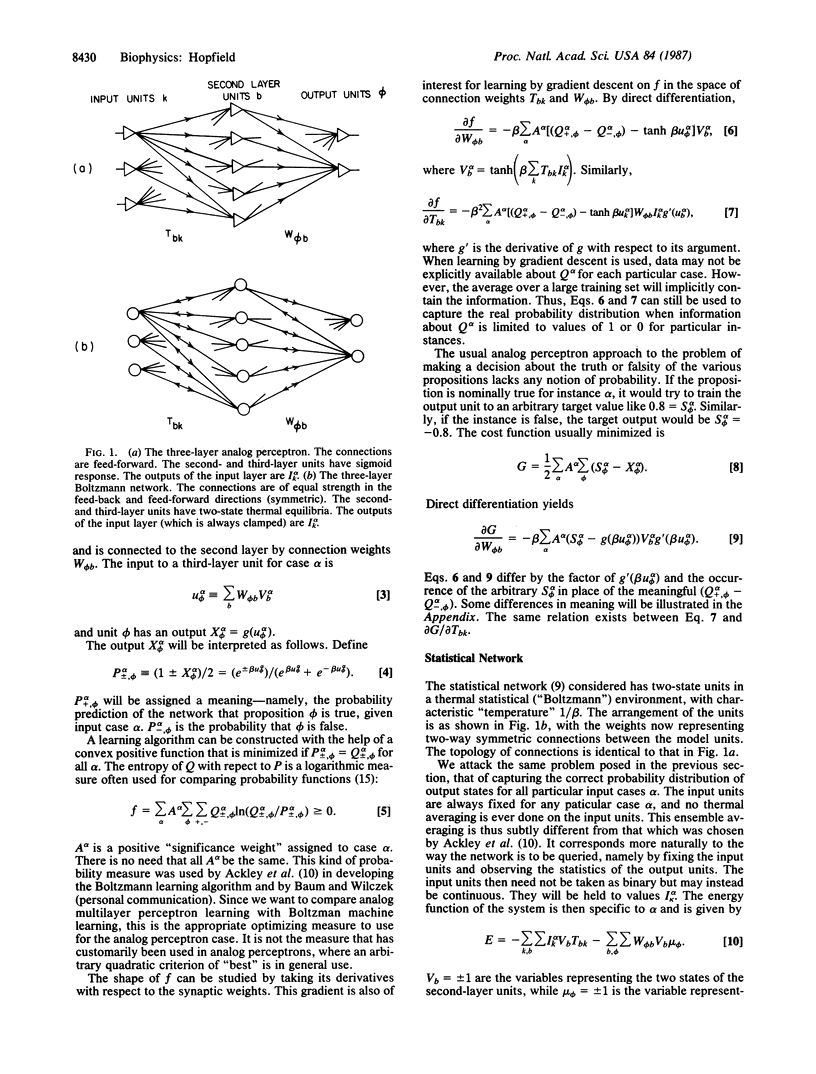
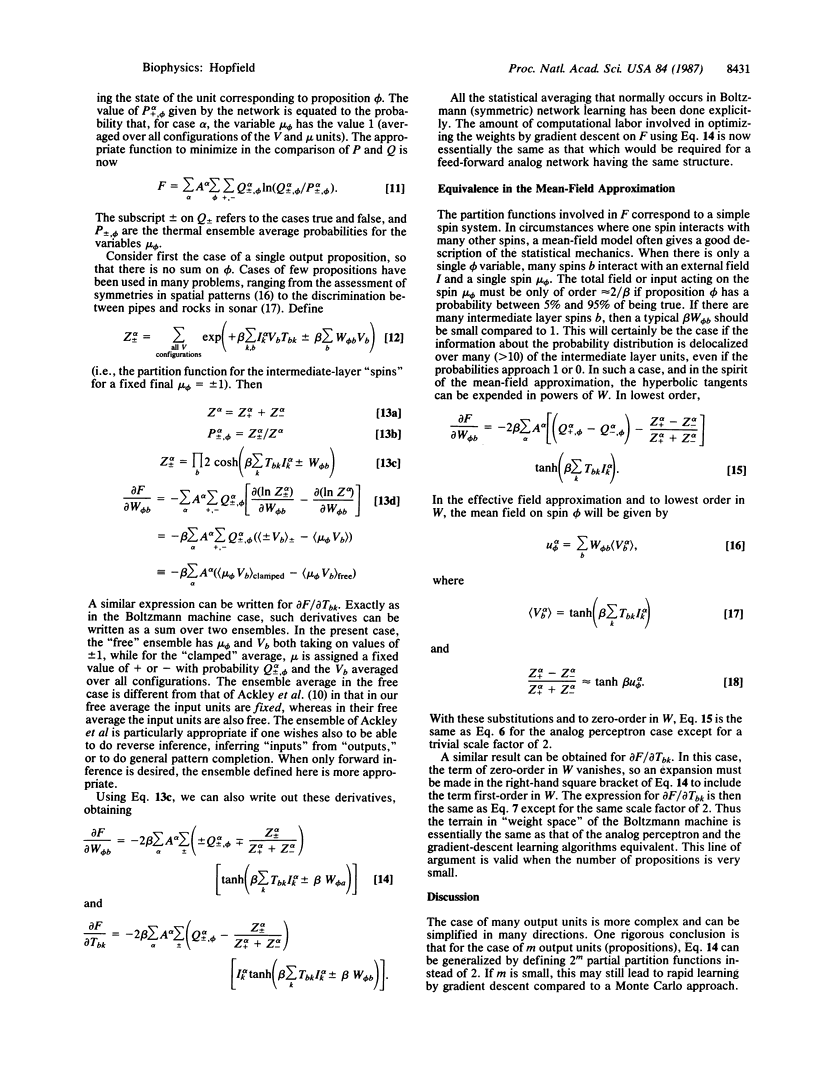
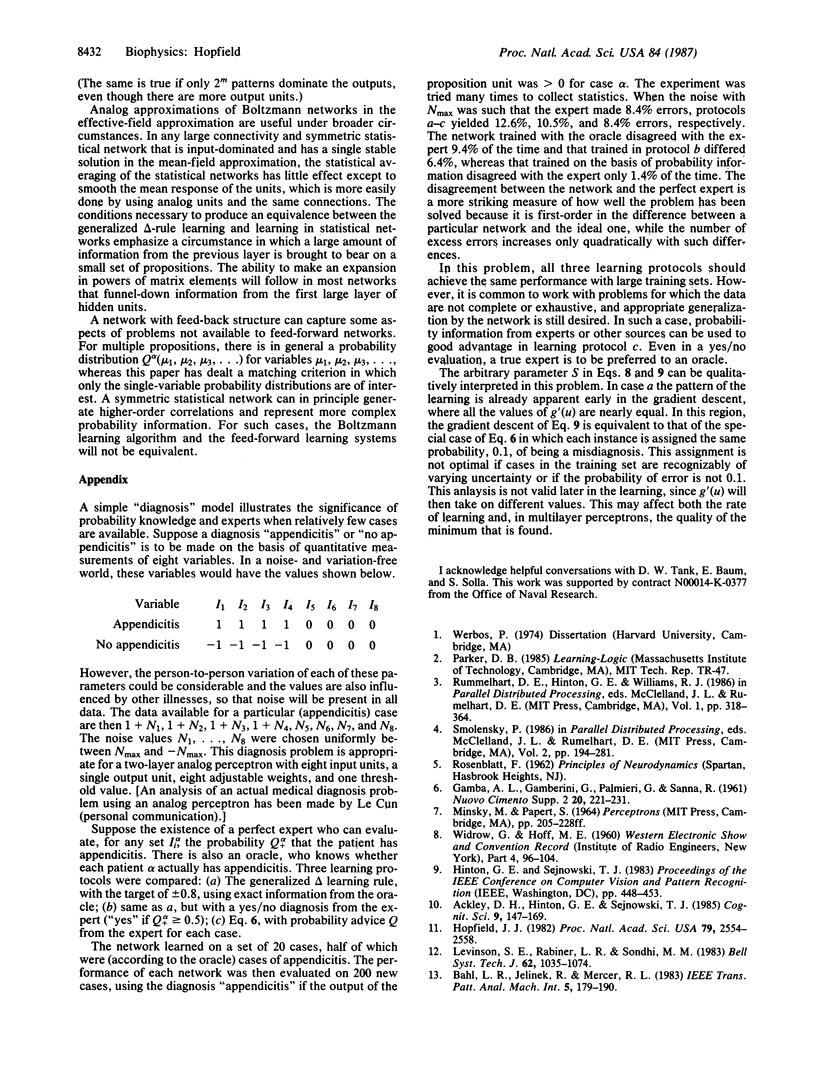
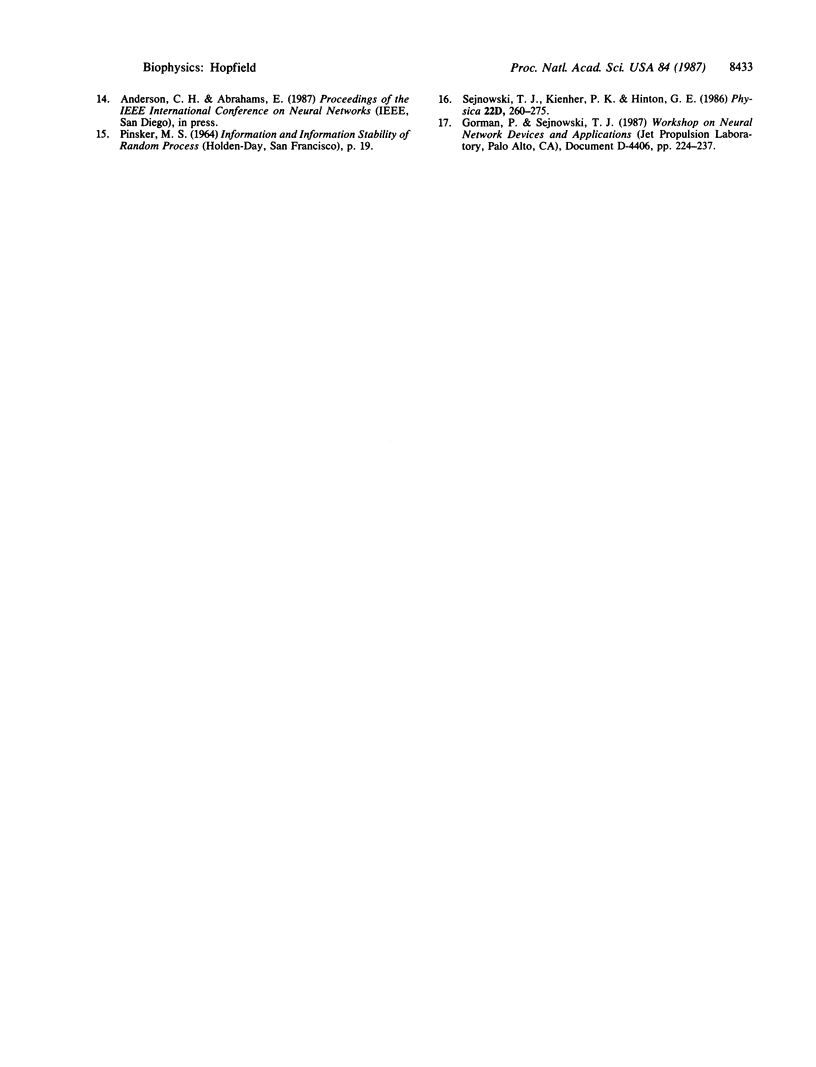
Selected References
These references are in PubMed. This may not be the complete list of references from this article.
- Hopfield J. J. Neural networks and physical systems with emergent collective computational abilities. Proc Natl Acad Sci U S A. 1982 Apr;79(8):2554–2558. doi: 10.1073/pnas.79.8.2554. [DOI] [PMC free article] [PubMed] [Google Scholar]