Abstract
A new method for predicting protein fold-classes and protein domains from sequence data is constructed and used for generating a data base of protein fold-class assignments. Any given sequence of amino acids is assigned a specific prediction of one out of 45 typical protein fold-classes, a prediction of one out of 4 super fold-classes for the content of secondary structures and a profile of fold-class predictions along the sequence. The prediction accuracy for the super fold-classes is around 91% correct and 82% correct for the specific fold-classes. This accuracy is maintained down to a few percent of sequence identity.
Full text
PDF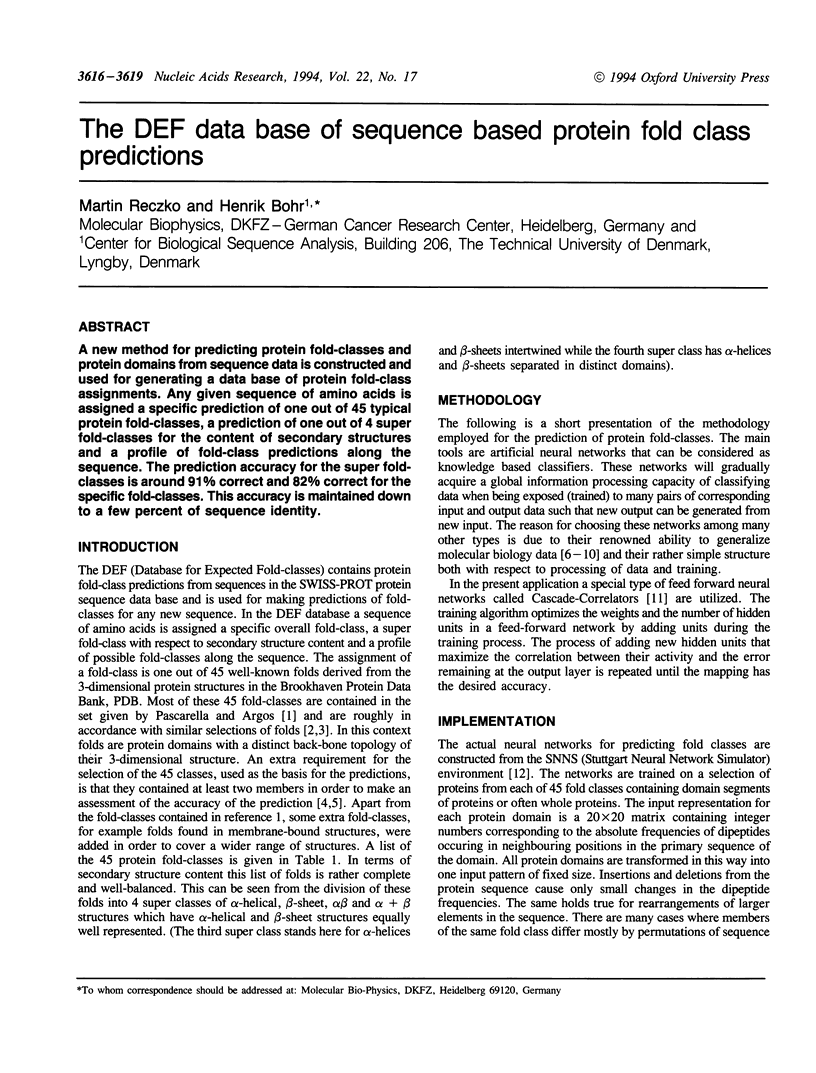
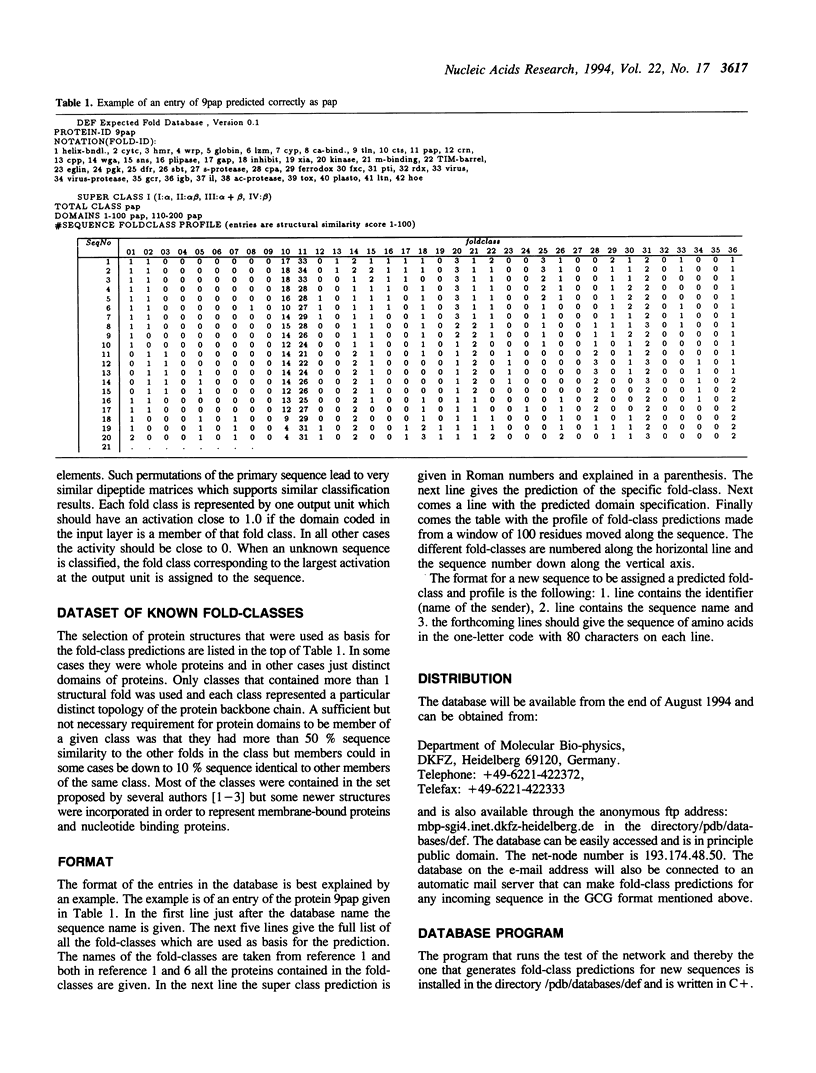
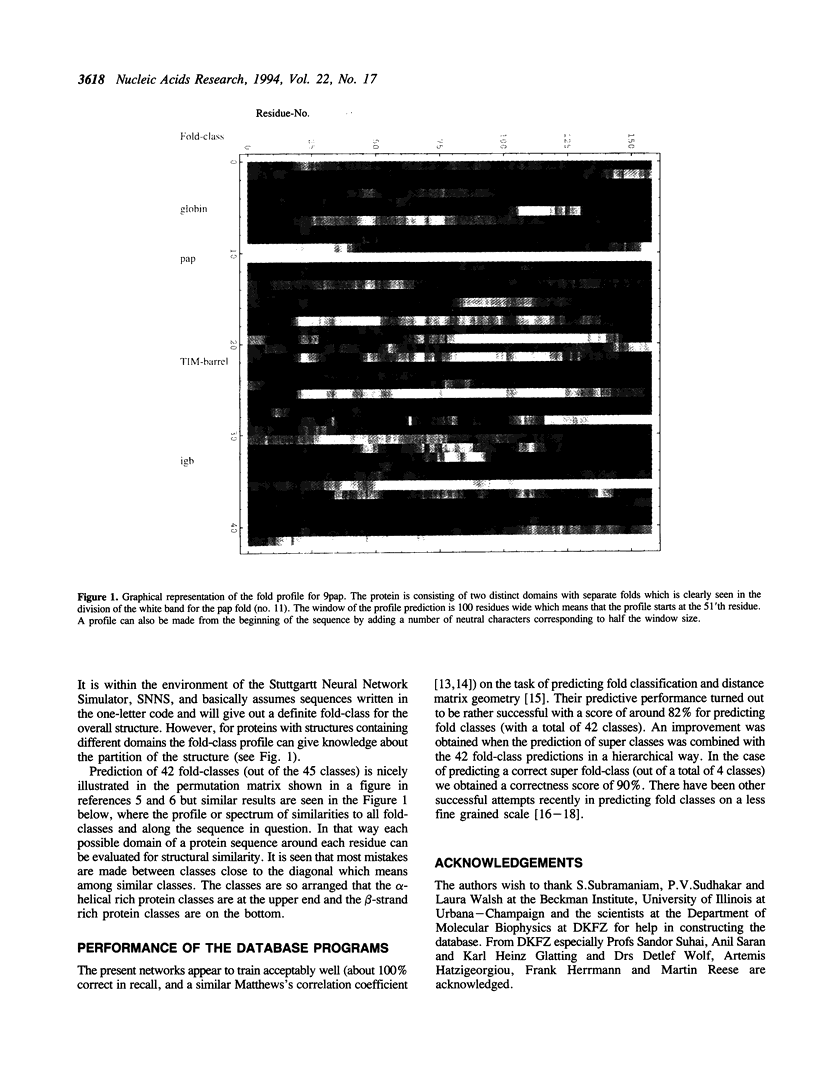
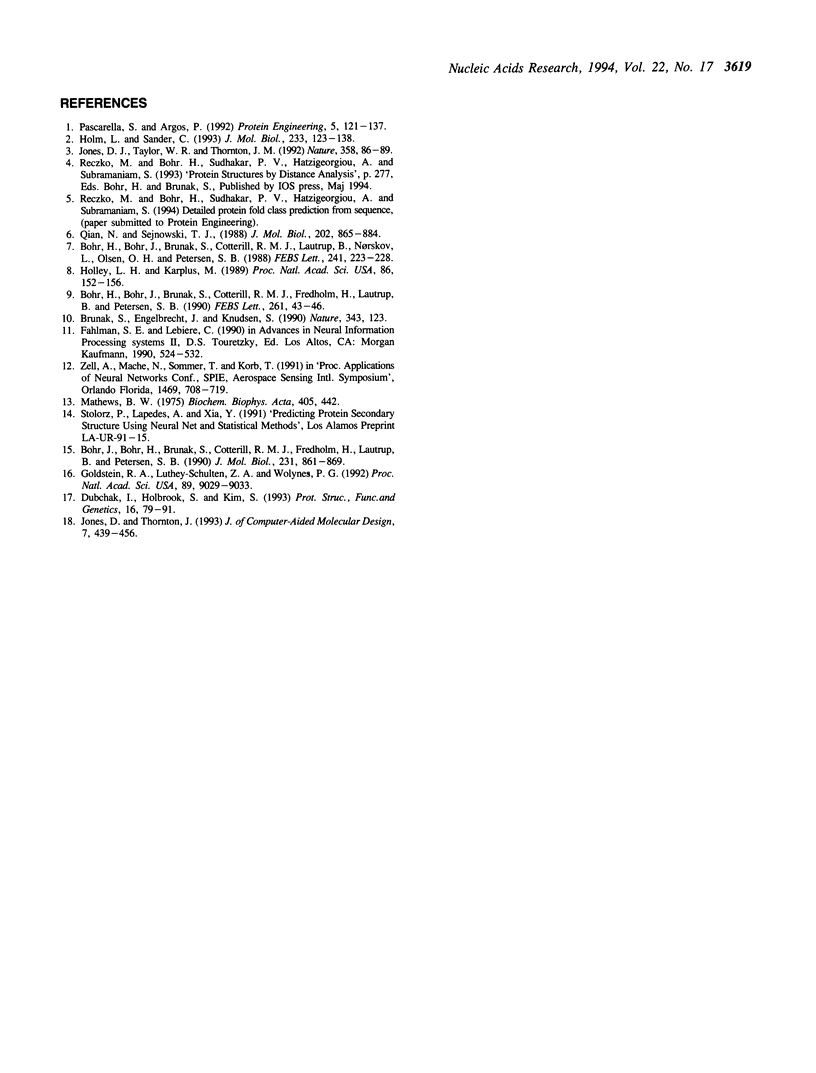
Images in this article
Selected References
These references are in PubMed. This may not be the complete list of references from this article.
- Bohr H., Bohr J., Brunak S., Cotterill R. M., Lautrup B., Nørskov L., Olsen O. H., Petersen S. B. Protein secondary structure and homology by neural networks. The alpha-helices in rhodopsin. FEBS Lett. 1988 Dec 5;241(1-2):223–228. doi: 10.1016/0014-5793(88)81066-4. [DOI] [PubMed] [Google Scholar]
- Bohr J., Bohr H., Brunak S., Cotterill R. M., Fredholm H., Lautrup B., Petersen S. B. Protein structures from distance inequalities. J Mol Biol. 1993 Jun 5;231(3):861–869. doi: 10.1006/jmbi.1993.1332. [DOI] [PubMed] [Google Scholar]
- Brunak S., Engelbrecht J., Knudsen S. Cleaning up gene databases. Nature. 1990 Jan 11;343(6254):123–123. doi: 10.1038/343123a0. [DOI] [PubMed] [Google Scholar]
- Dubchak I., Holbrook S. R., Kim S. H. Prediction of protein folding class from amino acid composition. Proteins. 1993 May;16(1):79–91. doi: 10.1002/prot.340160109. [DOI] [PubMed] [Google Scholar]
- Goldstein R. A., Luthey-Schulten Z. A., Wolynes P. G. Protein tertiary structure recognition using optimized Hamiltonians with local interactions. Proc Natl Acad Sci U S A. 1992 Oct 1;89(19):9029–9033. doi: 10.1073/pnas.89.19.9029. [DOI] [PMC free article] [PubMed] [Google Scholar]
- Holley L. H., Karplus M. Protein secondary structure prediction with a neural network. Proc Natl Acad Sci U S A. 1989 Jan;86(1):152–156. doi: 10.1073/pnas.86.1.152. [DOI] [PMC free article] [PubMed] [Google Scholar]
- Holm L., Sander C. Protein structure comparison by alignment of distance matrices. J Mol Biol. 1993 Sep 5;233(1):123–138. doi: 10.1006/jmbi.1993.1489. [DOI] [PubMed] [Google Scholar]
- Jones D. T., Taylor W. R., Thornton J. M. A new approach to protein fold recognition. Nature. 1992 Jul 2;358(6381):86–89. doi: 10.1038/358086a0. [DOI] [PubMed] [Google Scholar]
- Jones D., Thornton J. Protein fold recognition. J Comput Aided Mol Des. 1993 Aug;7(4):439–456. doi: 10.1007/BF02337560. [DOI] [PubMed] [Google Scholar]
- Matthews B. W. Comparison of the predicted and observed secondary structure of T4 phage lysozyme. Biochim Biophys Acta. 1975 Oct 20;405(2):442–451. doi: 10.1016/0005-2795(75)90109-9. [DOI] [PubMed] [Google Scholar]
- Pascarella S., Argos P. A data bank merging related protein structures and sequences. Protein Eng. 1992 Mar;5(2):121–137. doi: 10.1093/protein/5.2.121. [DOI] [PubMed] [Google Scholar]
- Qian N., Sejnowski T. J. Predicting the secondary structure of globular proteins using neural network models. J Mol Biol. 1988 Aug 20;202(4):865–884. doi: 10.1016/0022-2836(88)90564-5. [DOI] [PubMed] [Google Scholar]