Abstract
In many neural systems, sensory information is distributed throughout a population of neurons. We study simple neural network models for extracting this information. The inputs to the networks are the stochastic responses of a population of sensory neurons tuned to directional stimuli. The performance of each network model in psychophysical tasks is compared with that of the optimal maximum likelihood procedure. As a model of direction estimation in two dimensions, we consider a linear network that computes a population vector. Its performance depends on the width of the population tuning curves and is maximal for width, which increases with the level of background activity. Although for narrowly tuned neurons the performance of the population vector is significantly inferior to that of maximum likelihood estimation, the difference between the two is small when the tuning is broad. For direction discrimination, we consider two models: a perceptron with fully adaptive weights and a network made by adding an adaptive second layer to the population vector network. We calculate the error rates of these networks after exhaustive training to a particular direction. By testing on the full range of possible directions, the extent of transfer of training to novel stimuli can be calculated. It is found that for threshold linear networks the transfer of perceptual learning is nonmonotonic. Although performance deteriorates away from the training stimulus, it peaks again at an intermediate angle. This nonmonotonicity provides an important psychophysical test of these models.
Full text
PDF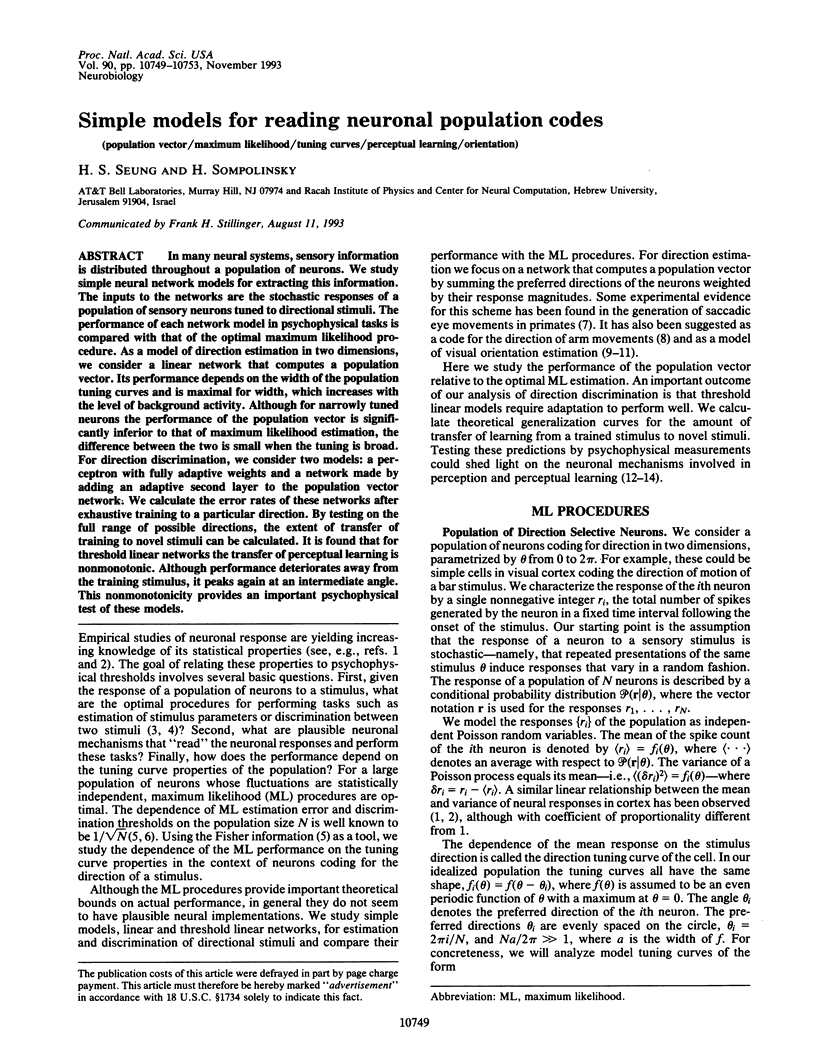
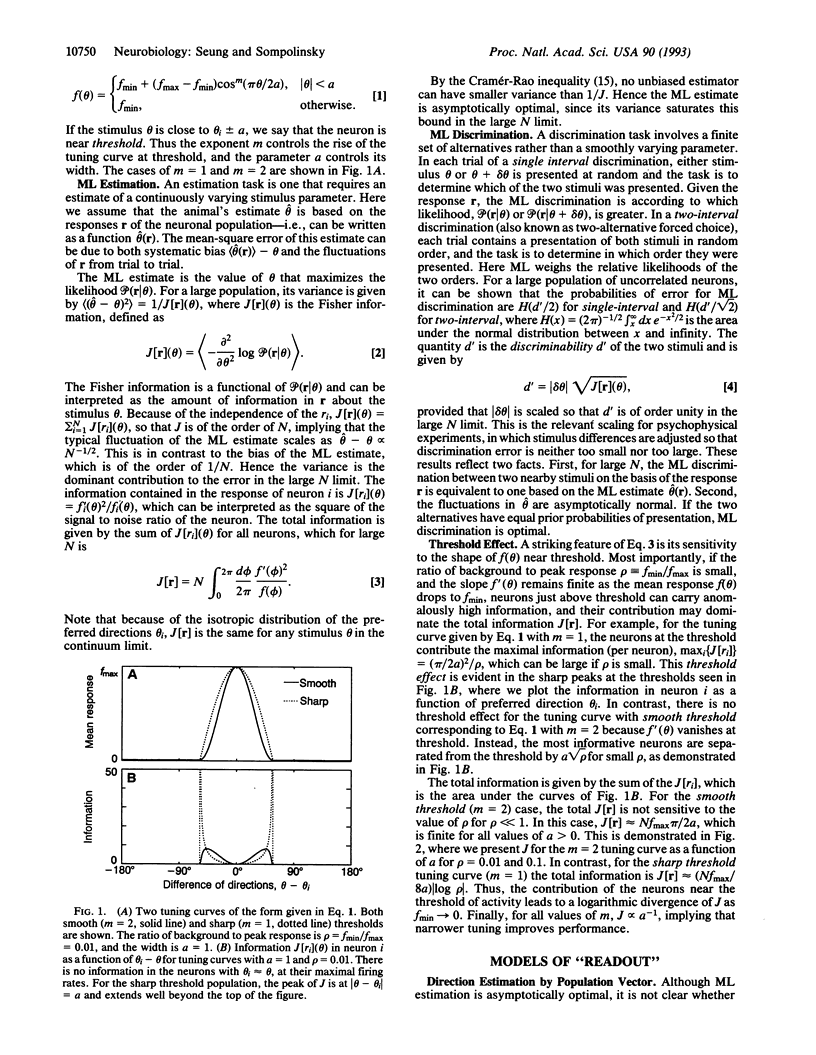
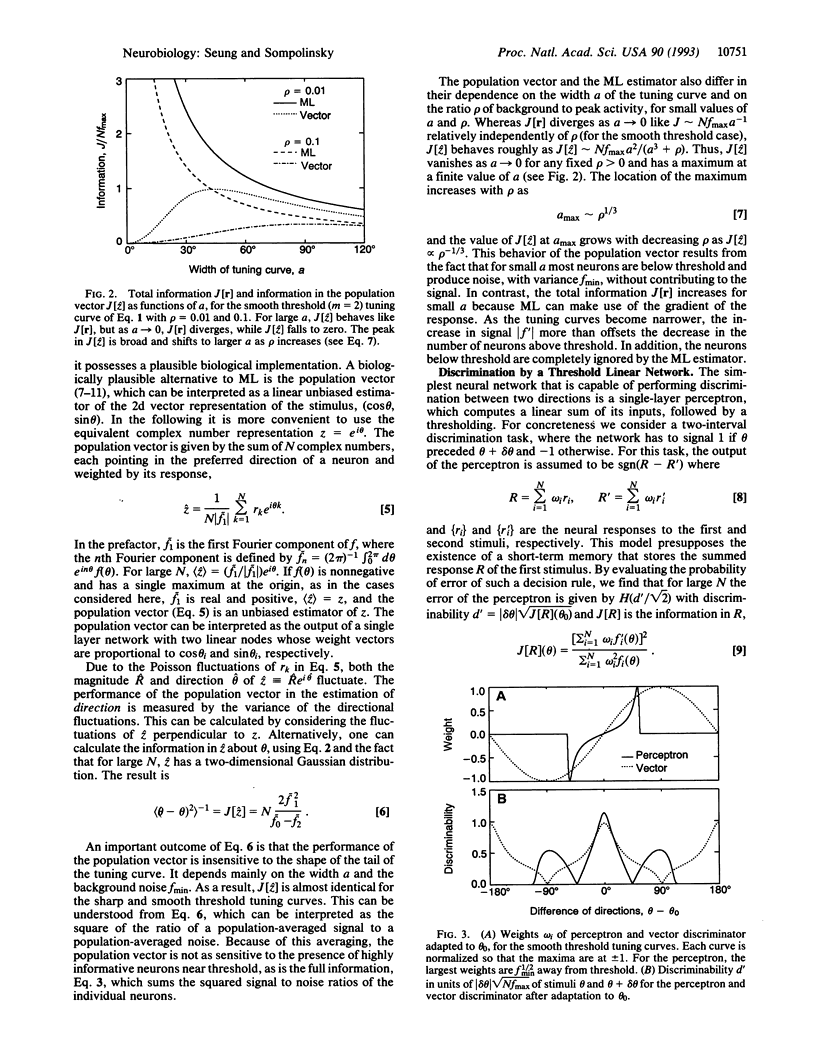
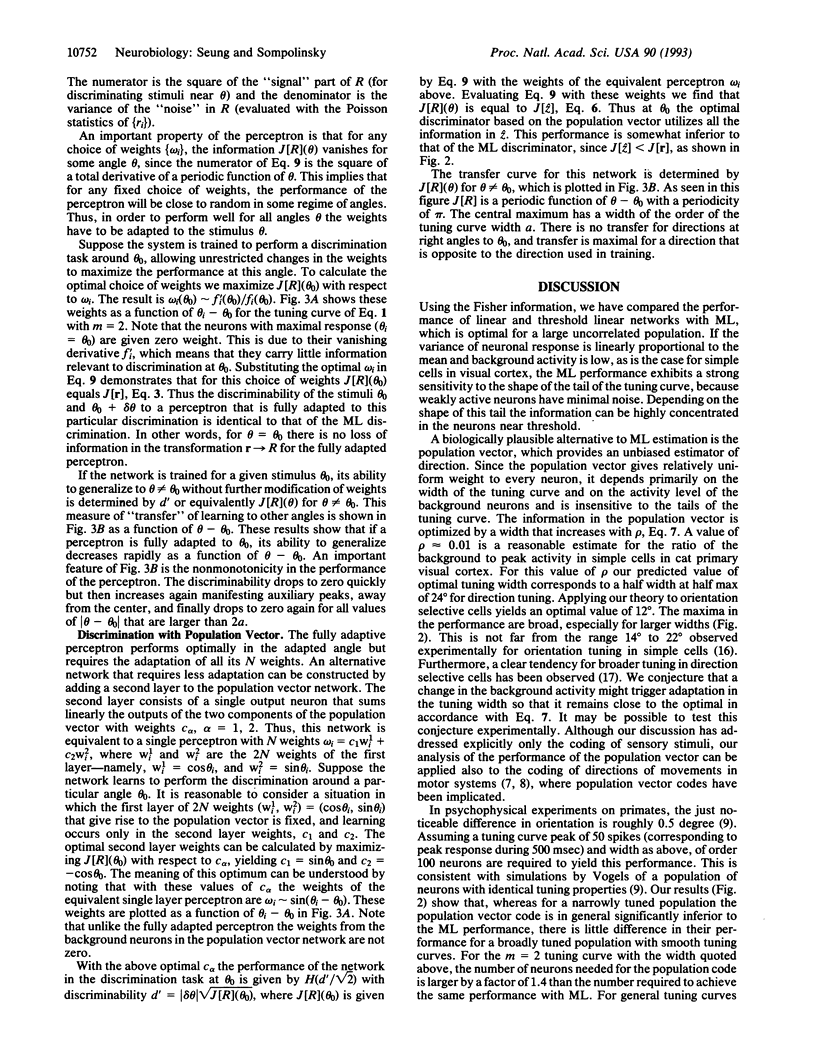
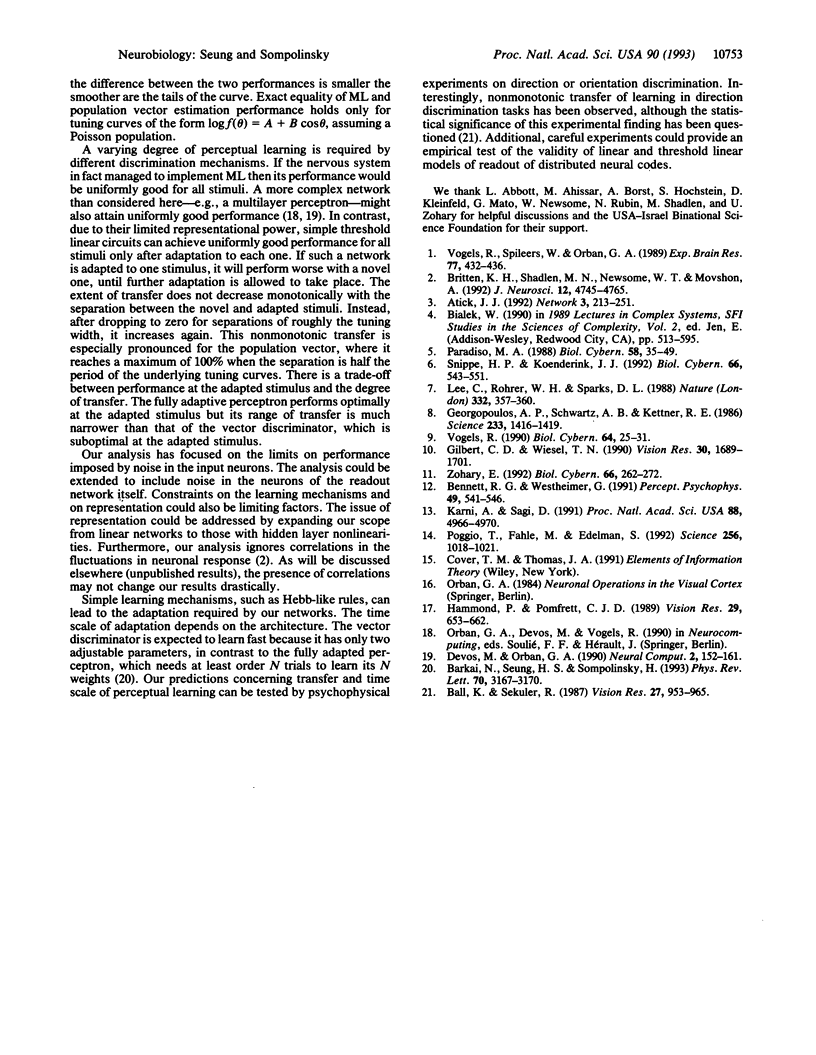
Selected References
These references are in PubMed. This may not be the complete list of references from this article.
- Ball K., Sekuler R. Direction-specific improvement in motion discrimination. Vision Res. 1987;27(6):953–965. doi: 10.1016/0042-6989(87)90011-3. [DOI] [PubMed] [Google Scholar]
- Barkai N, Seung HS, Sompolinsky H. Scaling laws in learning of classification tasks. Phys Rev Lett. 1993 May 17;70(20):3167–3170. doi: 10.1103/PhysRevLett.70.3167. [DOI] [PubMed] [Google Scholar]
- Bennett R. G., Westheimer G. The effect of training on visual alignment discrimination and grating resolution. Percept Psychophys. 1991 Jun;49(6):541–546. doi: 10.3758/bf03212188. [DOI] [PubMed] [Google Scholar]
- Britten K. H., Shadlen M. N., Newsome W. T., Movshon J. A. The analysis of visual motion: a comparison of neuronal and psychophysical performance. J Neurosci. 1992 Dec;12(12):4745–4765. doi: 10.1523/JNEUROSCI.12-12-04745.1992. [DOI] [PMC free article] [PubMed] [Google Scholar]
- Georgopoulos A. P., Schwartz A. B., Kettner R. E. Neuronal population coding of movement direction. Science. 1986 Sep 26;233(4771):1416–1419. doi: 10.1126/science.3749885. [DOI] [PubMed] [Google Scholar]
- Gilbert C. D., Wiesel T. N. The influence of contextual stimuli on the orientation selectivity of cells in primary visual cortex of the cat. Vision Res. 1990;30(11):1689–1701. doi: 10.1016/0042-6989(90)90153-c. [DOI] [PubMed] [Google Scholar]
- Hammond P., Pomfrett C. J. Directional and orientational tuning of feline striate cortical neurones: correlation with neuronal class. Vision Res. 1989;29(6):653–662. doi: 10.1016/0042-6989(89)90027-8. [DOI] [PubMed] [Google Scholar]
- Karni A., Sagi D. Where practice makes perfect in texture discrimination: evidence for primary visual cortex plasticity. Proc Natl Acad Sci U S A. 1991 Jun 1;88(11):4966–4970. doi: 10.1073/pnas.88.11.4966. [DOI] [PMC free article] [PubMed] [Google Scholar]
- Lee C., Rohrer W. H., Sparks D. L. Population coding of saccadic eye movements by neurons in the superior colliculus. Nature. 1988 Mar 24;332(6162):357–360. doi: 10.1038/332357a0. [DOI] [PubMed] [Google Scholar]
- Paradiso M. A. A theory for the use of visual orientation information which exploits the columnar structure of striate cortex. Biol Cybern. 1988;58(1):35–49. doi: 10.1007/BF00363954. [DOI] [PubMed] [Google Scholar]
- Poggio T., Fahle M., Edelman S. Fast perceptual learning in visual hyperacuity. Science. 1992 May 15;256(5059):1018–1021. doi: 10.1126/science.1589770. [DOI] [PubMed] [Google Scholar]
- Vogels R. Population coding of stimulus orientation by striate cortical cells. Biol Cybern. 1990;64(1):25–31. doi: 10.1007/BF00203627. [DOI] [PubMed] [Google Scholar]
- Vogels R., Spileers W., Orban G. A. The response variability of striate cortical neurons in the behaving monkey. Exp Brain Res. 1989;77(2):432–436. doi: 10.1007/BF00275002. [DOI] [PubMed] [Google Scholar]
- Zohary E. Population coding of visual stimuli by cortical neurons tuned to more than one dimension. Biol Cybern. 1992;66(3):265–272. doi: 10.1007/BF00198480. [DOI] [PubMed] [Google Scholar]