Abstract
Since the introduction by Shepp and Vardi [Shepp, L. A. & Vardi, Y. (1982) IEEE Trans. Med. Imaging 1, 113-121] of the expectation-maximization algorithm for the generation of maximum-likelihood images in emission tomography, a number of investigators have applied the maximum-likelihood method to imaging problems. Though this approach is promising, it is now well known that the unconstrained maximum-likelihood approach has two major drawbacks: (i) the algorithm is computationally demanding, resulting in reconstruction times that are not acceptable for routine clinical application, and (ii) the unconstrained maximum-likelihood estimator has a fundamental noise artifact that worsens as the iterative algorithm climbs the likelihood hill. In this paper the computation issue is addressed by proposing an implementation on the class of massively parallel single-instruction, multiple-data architectures. By restructuring the superposition integrals required for the expectation-maximization algorithm as the solutions of partial differential equations, the local data passage required for efficient computation on this class of machines is satisfied. For dealing with the "noise artifact" a Markov random field prior determined by Good's rotationally invariant roughness penalty is incorporated. These methods are demonstrated on the single-instruction multiple-data class of parallel processors, with the computation times compared with those on conventional and hypercube architectures.
Full text
PDF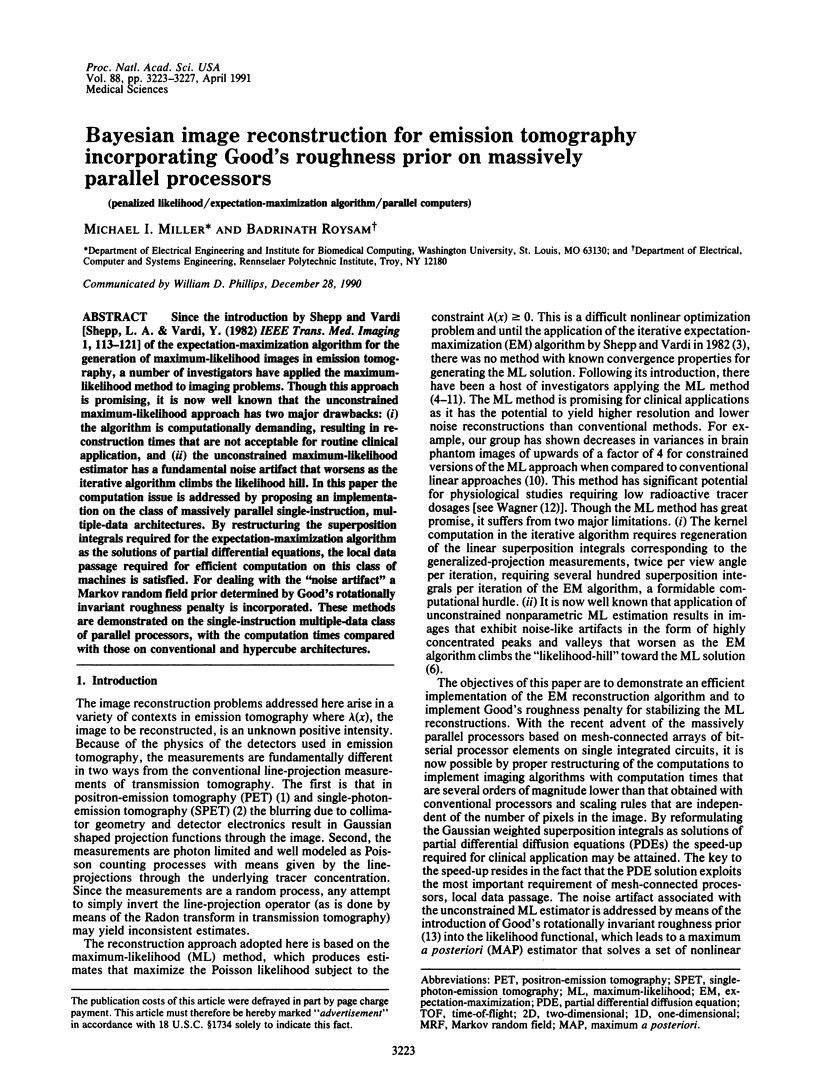
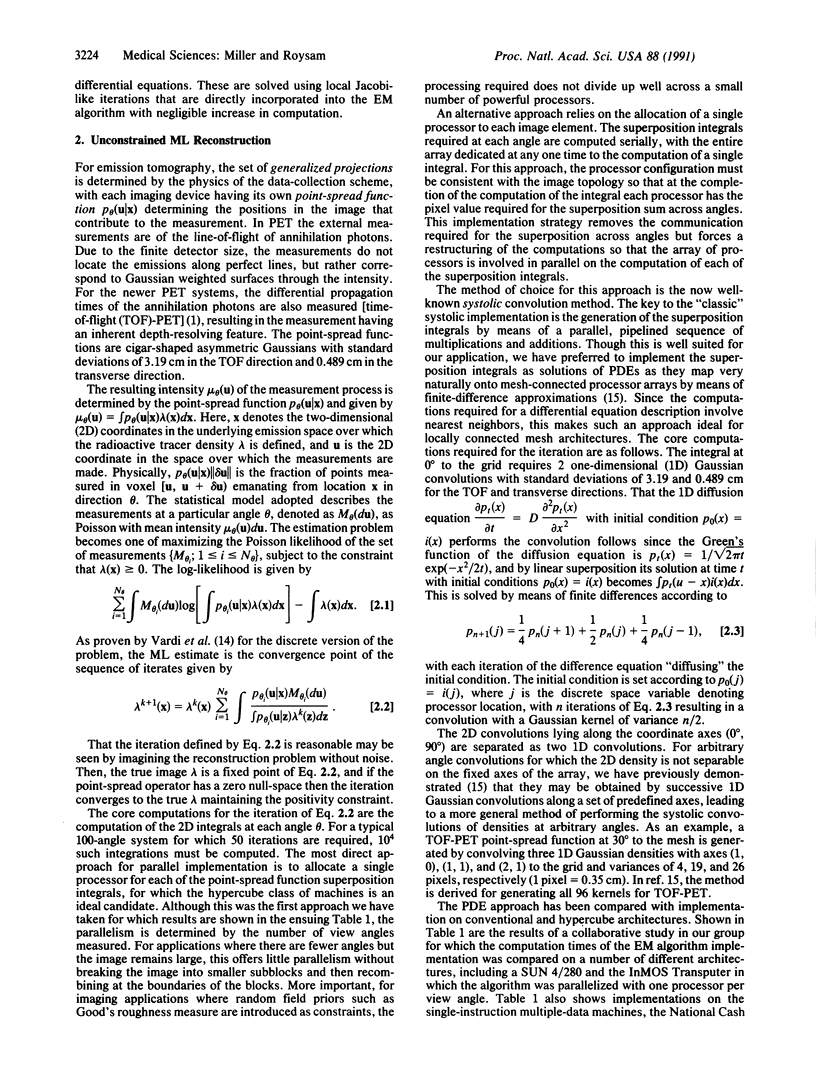
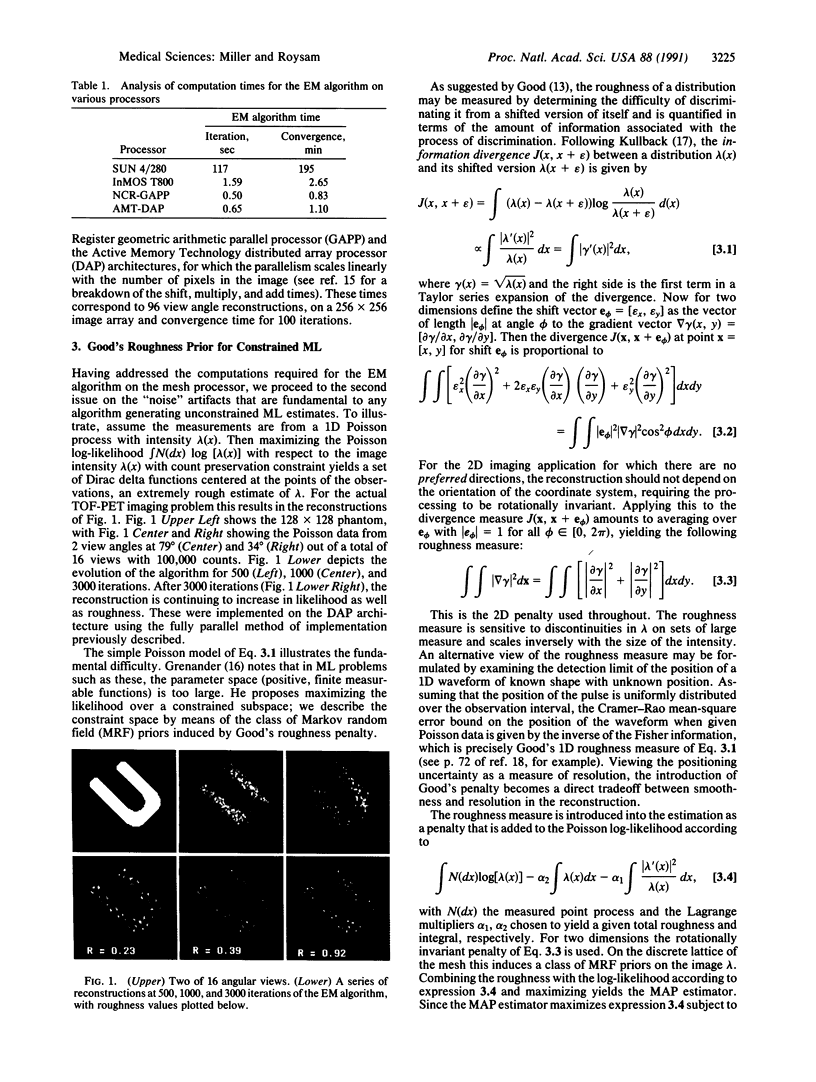
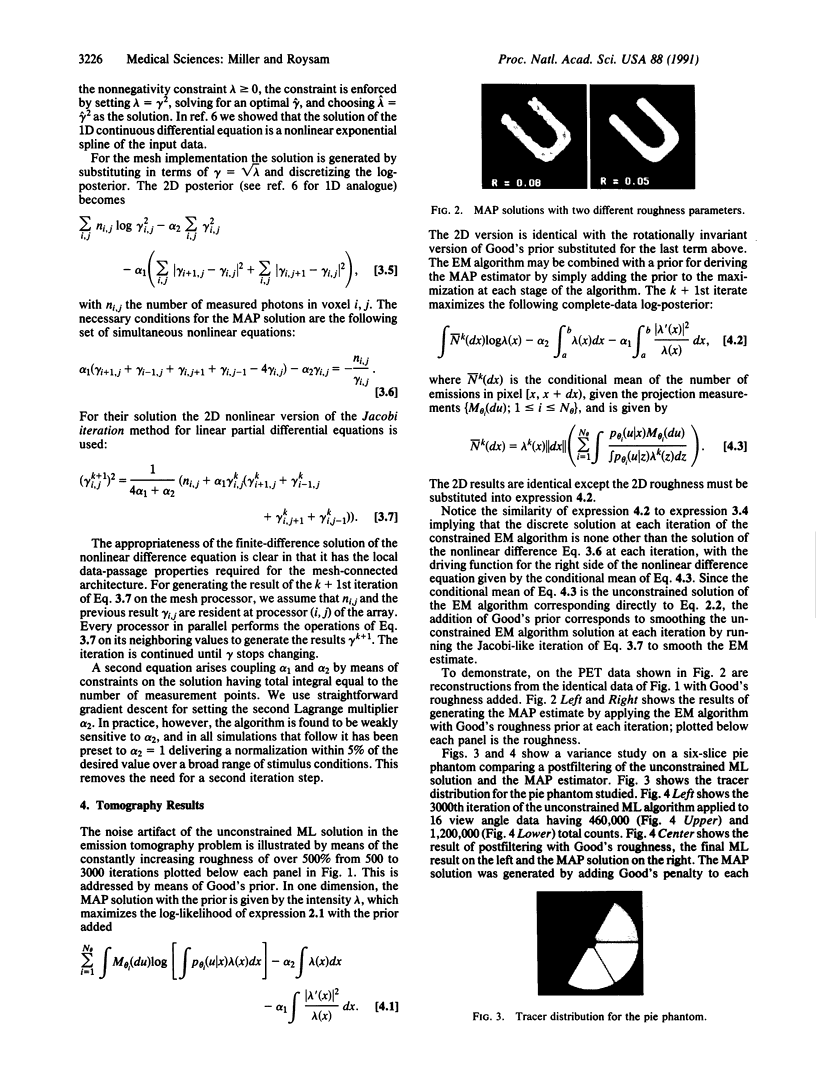
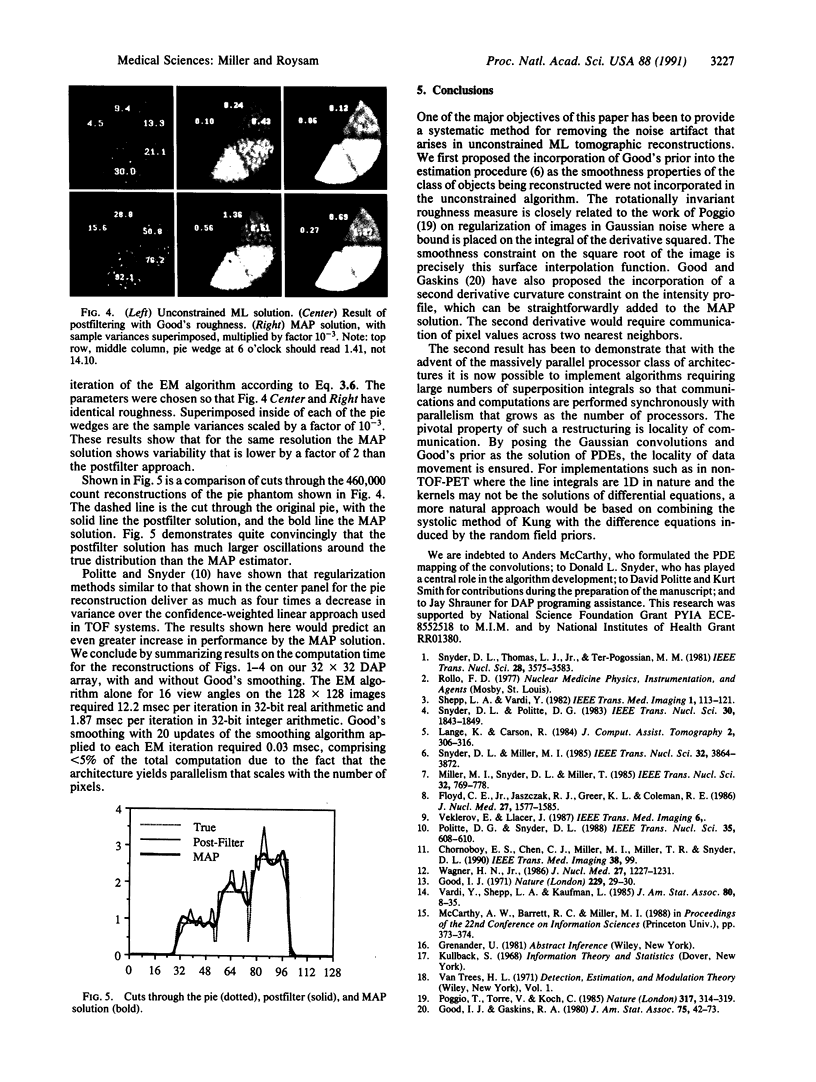
Images in this article
Selected References
These references are in PubMed. This may not be the complete list of references from this article.
- Floyd C. E., Jr, Jaszczak R. J., Greer K. L., Coleman R. E. Inverse Monte Carlo as a unified reconstruction algorithm for ECT. J Nucl Med. 1986 Oct;27(10):1577–1585. [PubMed] [Google Scholar]
- Lange K., Carson R. EM reconstruction algorithms for emission and transmission tomography. J Comput Assist Tomogr. 1984 Apr;8(2):306–316. [PubMed] [Google Scholar]
- Poggio T., Torre V., Koch C. Computational vision and regularization theory. 1985 Sep 26-Oct 2Nature. 317(6035):314–319. doi: 10.1038/317314a0. [DOI] [PubMed] [Google Scholar]