Abstract
Many recent studies have used artificial neural network algorithms to model how the brain might process information. However, back-propagation learning, the method that is generally used to train these networks, is distinctly "unbiological." We describe here a more biologically plausible learning rule, using reinforcement learning, which we have applied to the problem of how area 7a in the posterior parietal cortex of monkeys might represent visual space in head-centered coordinates. The network behaves similarly to networks trained by using back-propagation and to neurons recorded in area 7a. These results show that a neural network does not require back propagation to acquire biologically interesting properties.
Full text
PDF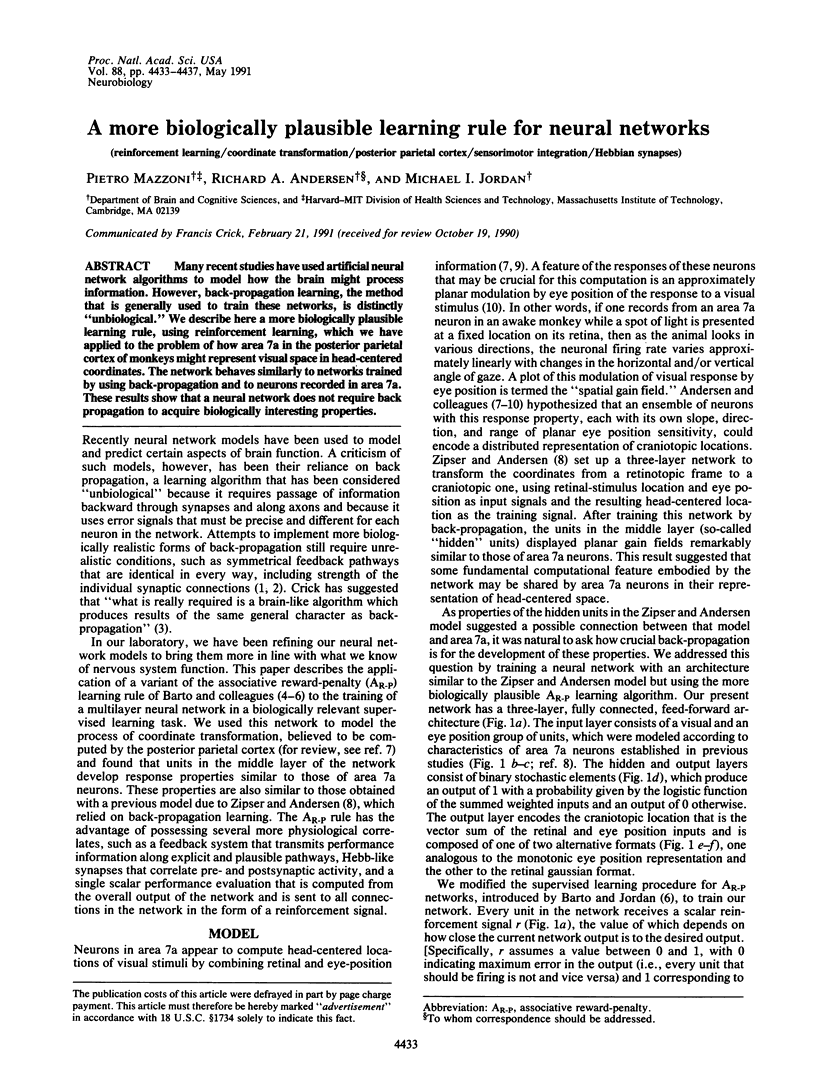
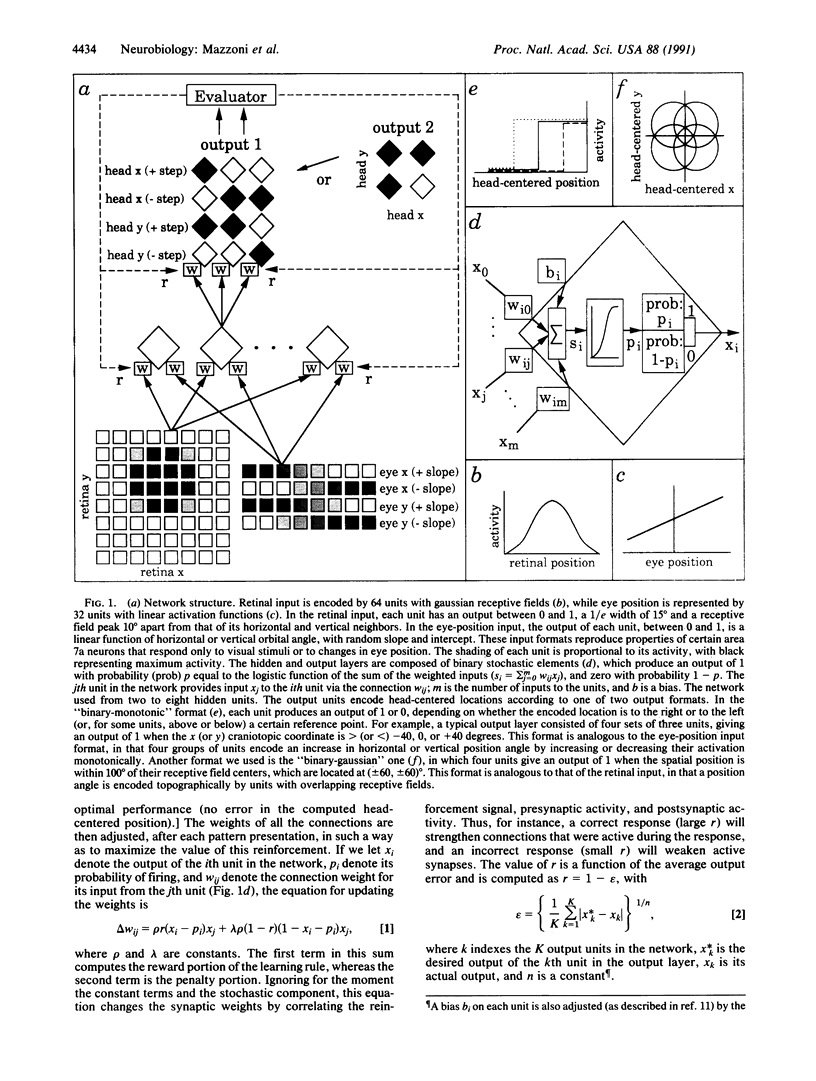
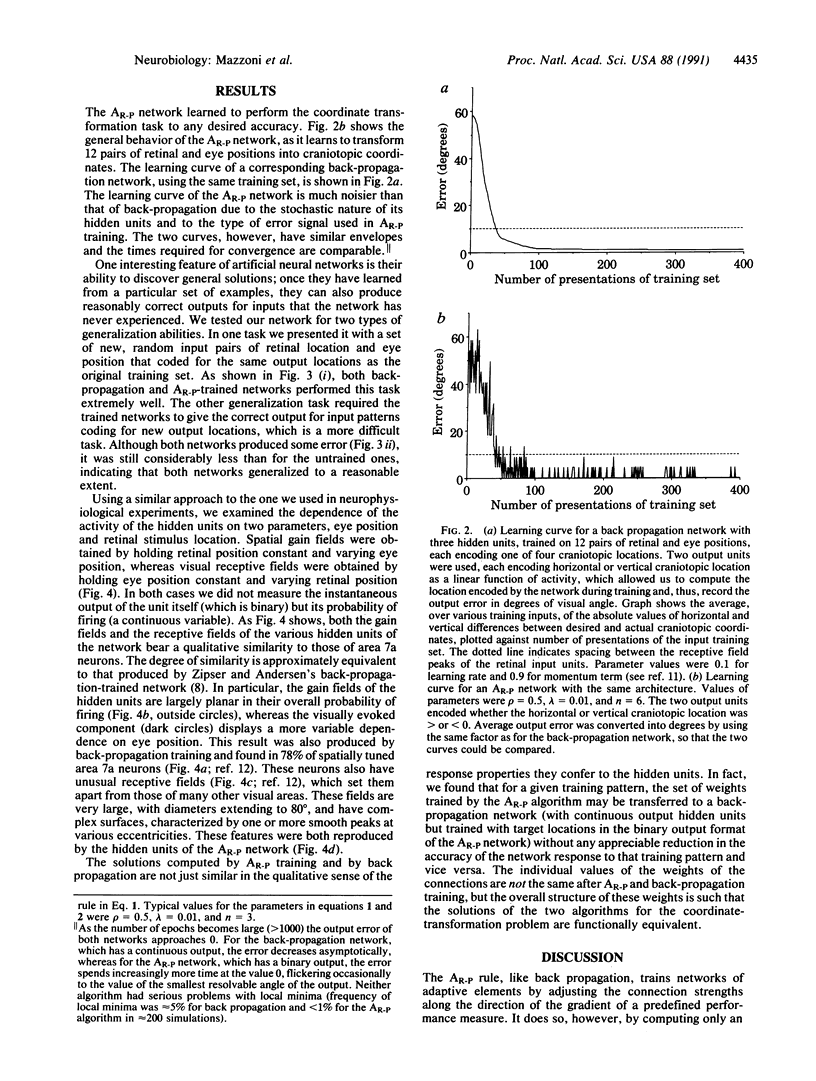
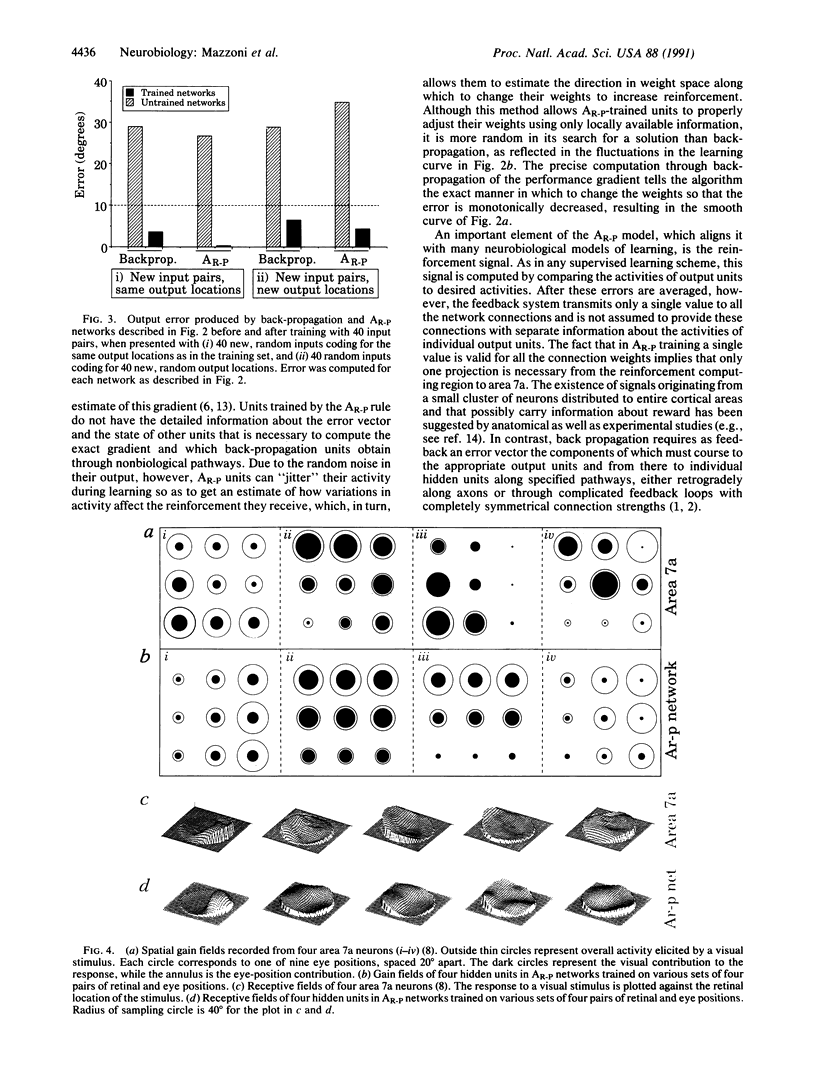
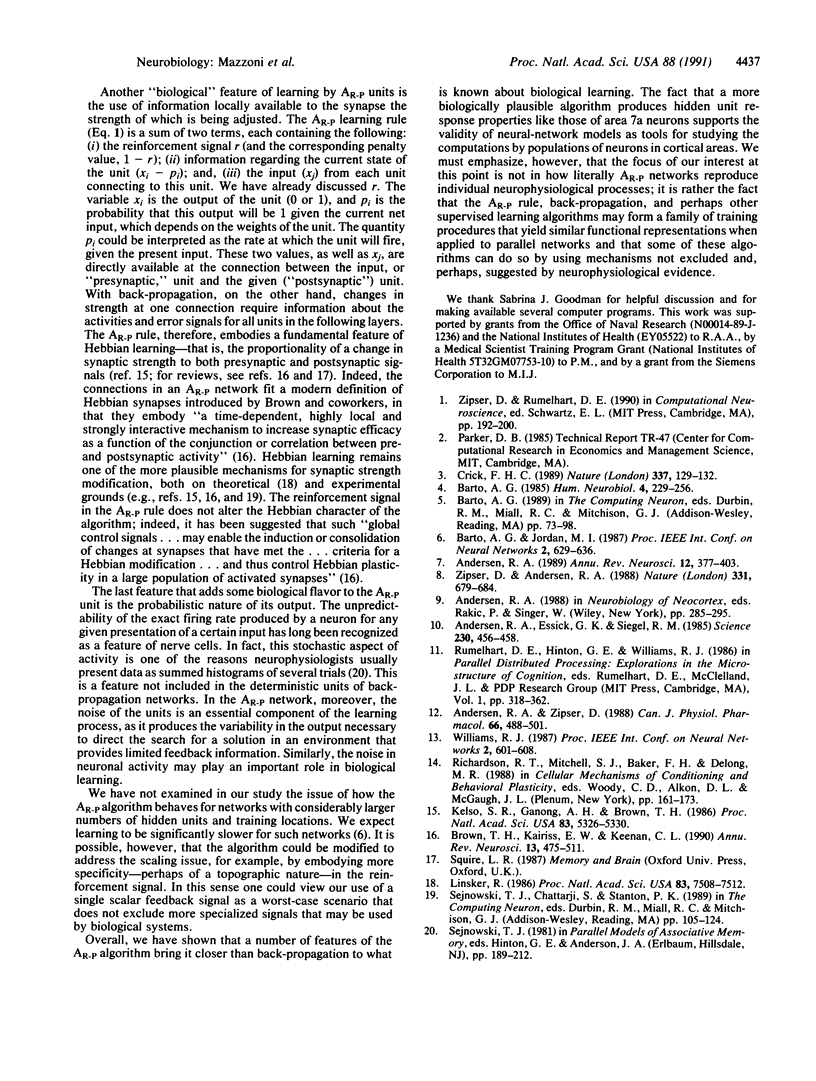
Images in this article
Selected References
These references are in PubMed. This may not be the complete list of references from this article.
- Andersen R. A., Essick G. K., Siegel R. M. Encoding of spatial location by posterior parietal neurons. Science. 1985 Oct 25;230(4724):456–458. doi: 10.1126/science.4048942. [DOI] [PubMed] [Google Scholar]
- Andersen R. A. Visual and eye movement functions of the posterior parietal cortex. Annu Rev Neurosci. 1989;12:377–403. doi: 10.1146/annurev.ne.12.030189.002113. [DOI] [PubMed] [Google Scholar]
- Andersen R. A., Zipser D. The role of the posterior parietal cortex in coordinate transformations for visual-motor integration. Can J Physiol Pharmacol. 1988 Apr;66(4):488–501. doi: 10.1139/y88-078. [DOI] [PubMed] [Google Scholar]
- Barto A. G. Learning by statistical cooperation of self-interested neuron-like computing elements. Hum Neurobiol. 1985;4(4):229–256. [PubMed] [Google Scholar]
- Brown T. H., Kairiss E. W., Keenan C. L. Hebbian synapses: biophysical mechanisms and algorithms. Annu Rev Neurosci. 1990;13:475–511. doi: 10.1146/annurev.ne.13.030190.002355. [DOI] [PubMed] [Google Scholar]
- Crick F. The recent excitement about neural networks. Nature. 1989 Jan 12;337(6203):129–132. doi: 10.1038/337129a0. [DOI] [PubMed] [Google Scholar]
- Kelso S. R., Ganong A. H., Brown T. H. Hebbian synapses in hippocampus. Proc Natl Acad Sci U S A. 1986 Jul;83(14):5326–5330. doi: 10.1073/pnas.83.14.5326. [DOI] [PMC free article] [PubMed] [Google Scholar]
- Linsker R. From basic network principles to neural architecture: emergence of spatial-opponent cells. Proc Natl Acad Sci U S A. 1986 Oct;83(19):7508–7512. doi: 10.1073/pnas.83.19.7508. [DOI] [PMC free article] [PubMed] [Google Scholar]
- Zipser D., Andersen R. A. A back-propagation programmed network that simulates response properties of a subset of posterior parietal neurons. Nature. 1988 Feb 25;331(6158):679–684. doi: 10.1038/331679a0. [DOI] [PubMed] [Google Scholar]