Abstract
We propose a reversible reaction mechanism with a single stationary state in which certain concentrations assume either high or low values dependent on the concentration of a catalyst. The properties of this mechanism are those of a McCulloch-Pitts neuron. We suggest a mechanism of interneuronal connections in which the stationary state of a chemical neuron is determined by the state of other neurons in a homogeneous chemical system and is thus a "hardware" chemical implementation of neural networks. Specific connections are determined for the construction of logic gates: AND, NOR, etc. Neural networks may be constructed in which the flow of time is continuous and computations are achieved by the attainment of a stationary state of the entire chemical reaction system, or in which the flow of time is discretized by an oscillatory reaction. In another article, we will give a chemical implementation of finite state machines and stack memories, with which in principle the construction of a universal Turing machine is possible.
Full text
PDF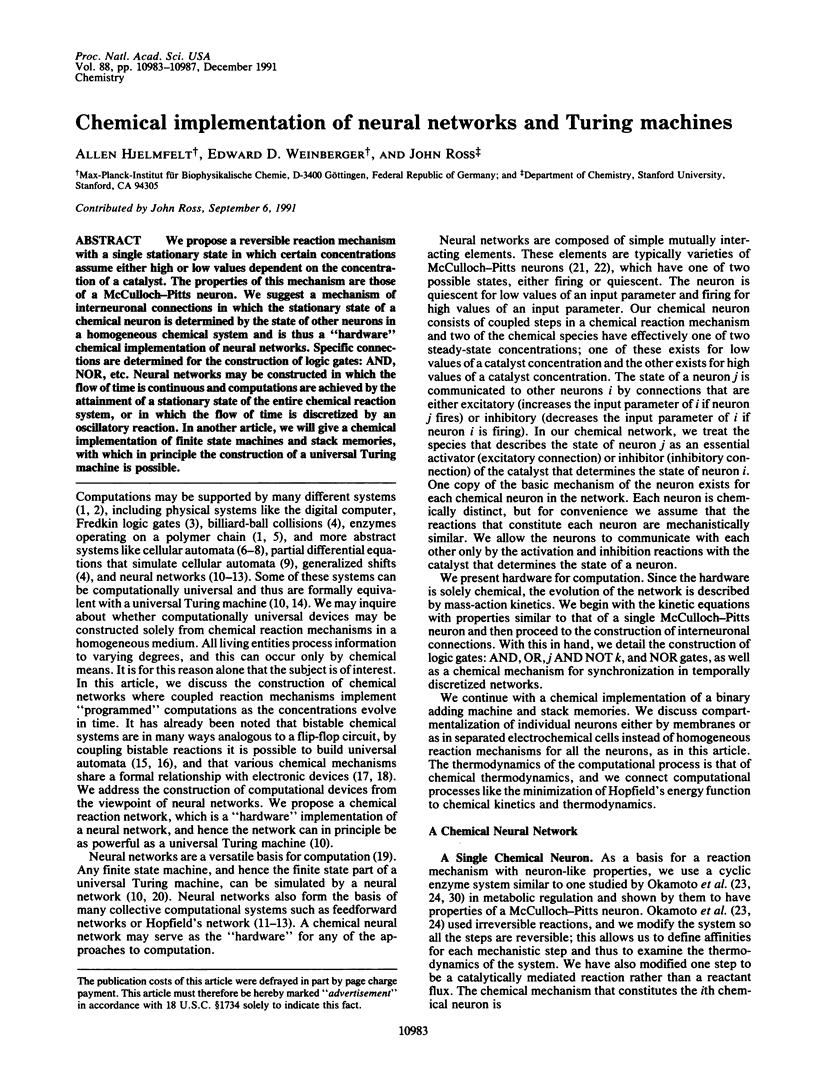
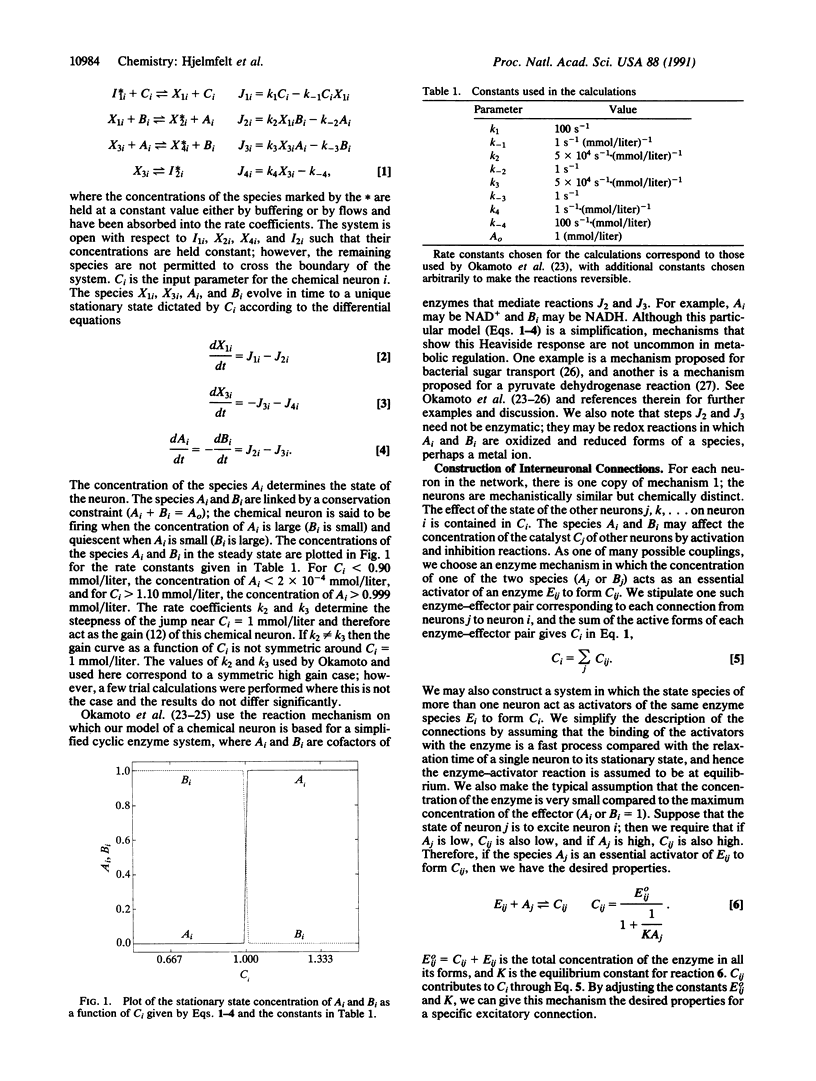
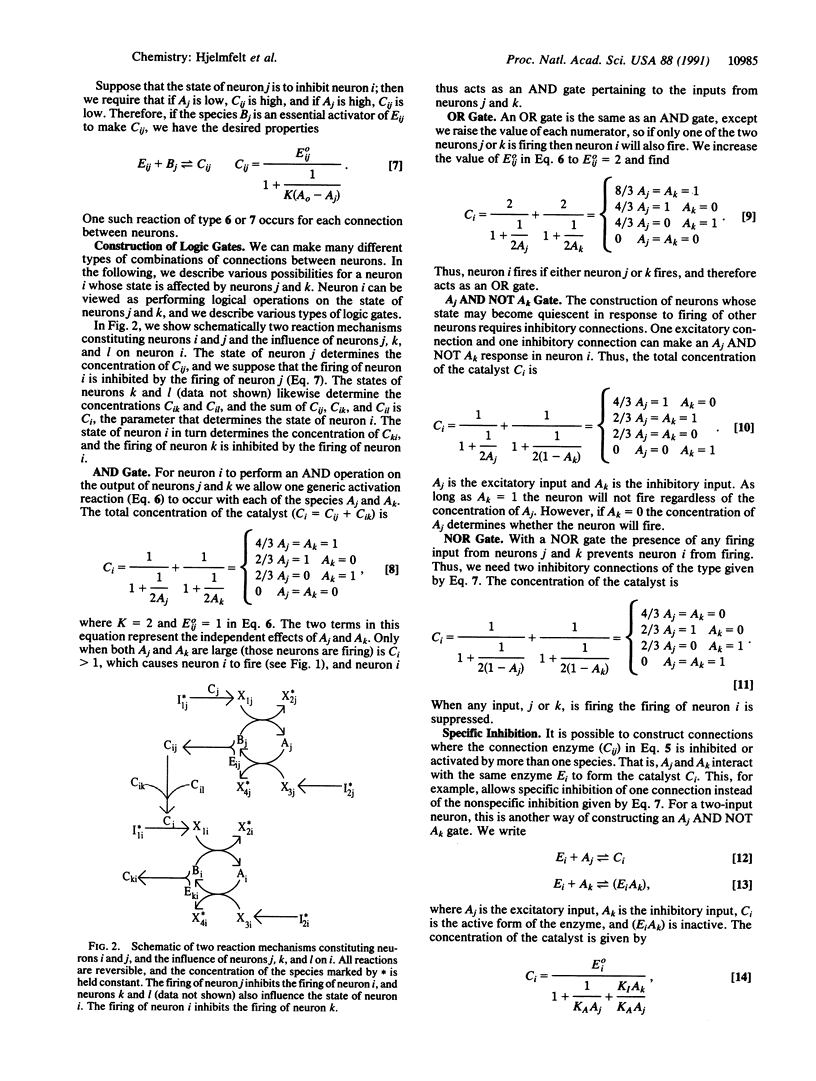
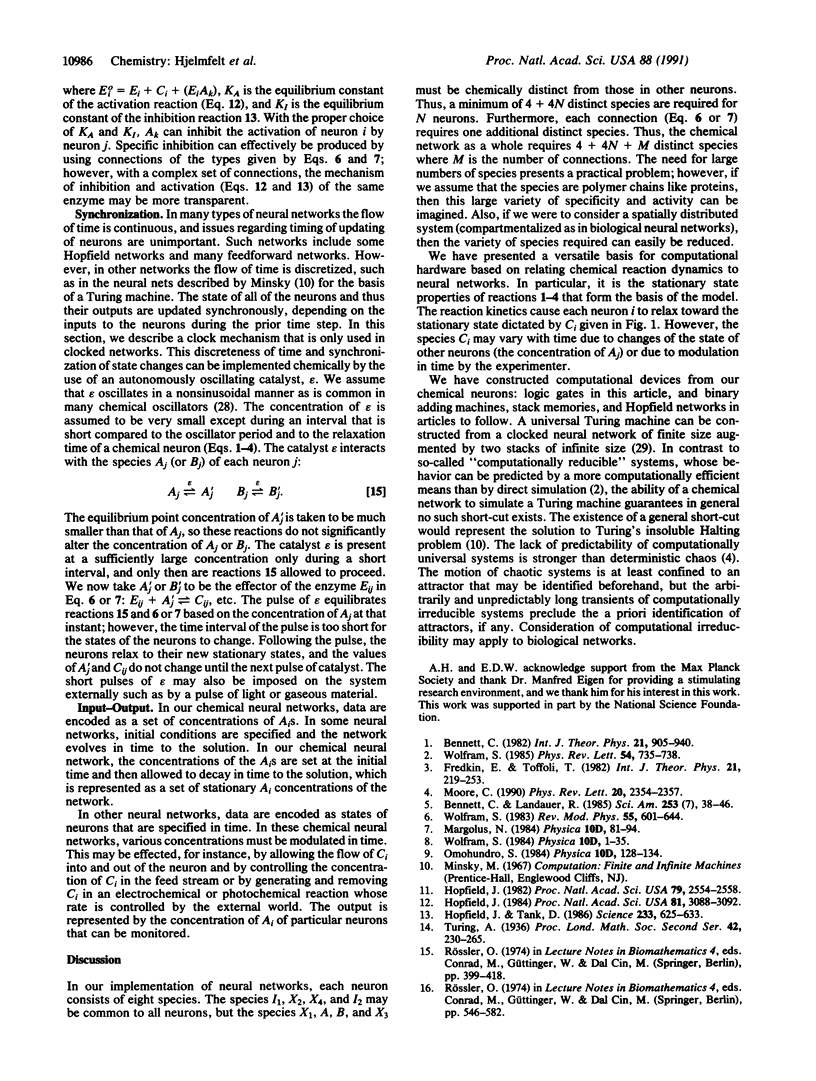
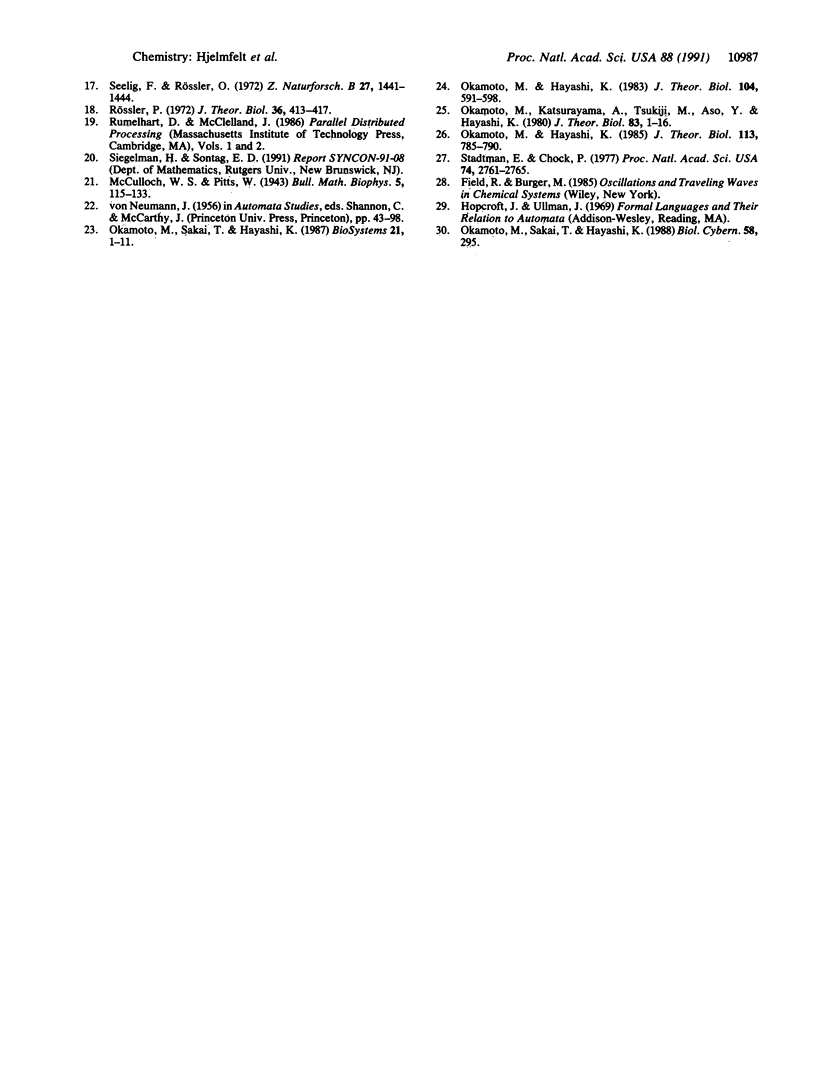
Selected References
These references are in PubMed. This may not be the complete list of references from this article.
- Hopfield J. J. Neural networks and physical systems with emergent collective computational abilities. Proc Natl Acad Sci U S A. 1982 Apr;79(8):2554–2558. doi: 10.1073/pnas.79.8.2554. [DOI] [PMC free article] [PubMed] [Google Scholar]
- Hopfield J. J. Neurons with graded response have collective computational properties like those of two-state neurons. Proc Natl Acad Sci U S A. 1984 May;81(10):3088–3092. doi: 10.1073/pnas.81.10.3088. [DOI] [PMC free article] [PubMed] [Google Scholar]
- Hopfield J. J., Tank D. W. Computing with neural circuits: a model. Science. 1986 Aug 8;233(4764):625–633. doi: 10.1126/science.3755256. [DOI] [PubMed] [Google Scholar]
- Moore C. Unpredictability and undecidability in dynamical systems. Phys Rev Lett. 1990 May 14;64(20):2354–2357. doi: 10.1103/PhysRevLett.64.2354. [DOI] [PubMed] [Google Scholar]
- Okamoto M., Hayashi K. Dynamic behavior of cyclic enzyme systems. J Theor Biol. 1983 Oct 21;104(4):591–598. doi: 10.1016/0022-5193(83)90247-3. [DOI] [PubMed] [Google Scholar]
- Okamoto M., Katsurayama A., Tsukiji M., Aso Y., Hayashi K. Dynamic behavior of enzymatic system realizing two-factor model. J Theor Biol. 1980 Mar 7;83(1):1–16. doi: 10.1016/0022-5193(80)90369-0. [DOI] [PubMed] [Google Scholar]
- Okamoto M., Sakai T., Hayashi K. Biochemical switching device realizing McCulloch-Pitts type equation. Biol Cybern. 1988;58(5):295–299. doi: 10.1007/BF00363938. [DOI] [PubMed] [Google Scholar]
- Okamoto M., Sakai T., Hayashi K. Switching mechanism of a cyclic enzyme system: role as a "chemical diode". Biosystems. 1987;21(1):1–11. doi: 10.1016/0303-2647(87)90002-5. [DOI] [PubMed] [Google Scholar]
- Stadtman E. R., Chock P. B. Superiority of interconvertible enzyme cascades in metabolic regulation: analysis of monocyclic systems. Proc Natl Acad Sci U S A. 1977 Jul;74(7):2761–2765. doi: 10.1073/pnas.74.7.2761. [DOI] [PMC free article] [PubMed] [Google Scholar]
- Wolfram S. Undecidability and intractability in theoretical physics. Phys Rev Lett. 1985 Feb 25;54(8):735–738. doi: 10.1103/PhysRevLett.54.735. [DOI] [PubMed] [Google Scholar]