Abstract
Most studies of networks have only looked at small subsets of the true network. Here, we discuss the sampling properties of a network's degree distribution under the most parsimonious sampling scheme. Only if the degree distributions of the network and randomly sampled subnets belong to the same family of probability distributions is it possible to extrapolate from subnet data to properties of the global network. We show that this condition is indeed satisfied for some important classes of networks, notably classical random graphs and exponential random graphs. For scale-free degree distributions, however, this is not the case. Thus, inferences about the scale-free nature of a network may have to be treated with some caution. The work presented here has important implications for the analysis of molecular networks as well as for graph theory and the theory of networks in general.
Keywords: complex networks, protein interaction networks, random graphs, sampling theory
Over the last few years, it has been suggested that many technological, social, and biological networks may be characterized as scale-free (1–3): that is, the majority of nodes in such networks have only a few connections to other nodes, whereas some nodes are connected to many other nodes in the network and the degree distribution decays much slower than exponentially. In many cases, it has been found to be well described by a power-law, and for the case of an infinite network, we can write for the probability of a node having k connections
![]() |
[1] |
where ζ(γ) is Riemann's zeta function, which normalizes the distribution such that . Such models are called scale-free because the ratio P(α × k)/P(k) depends only on α but not on k.
One of the particular attractions of such scale-free networks is that they can be generated by simple and plausible models (1). Networks that grow by new nodes preferentially forming connections with nodes that are already highly connected, for example, do give rise to scale-free networks. For instance, suppose new connections are formed at some constant rate, attached to new nodes (with probability p) or to existing nodes (with overall probability 1 – p and with relative probability k of attaching to a node having k links). This assumption asymptotically gives the distribution of Eq. 1, with the exponent γ = (2 – p)/(1 – p). This model could offer an explanation of how some network structures have evolved. But even if such a mechanistic model is incorrect, a corresponding statistical ensemble based on such models can still offer meaningful insights into network properties (4).
It is important to note, however, that in practice, many surveyed networks to date have been subnets of much larger networks. This finding is true for protein interaction (5, 6), gene regulation (7), and metabolic networks (8), where only a subset of the molecular entities in a cell have been sampled, as well as some social networks (9), which often include only subsets of interacting individuals. Sexual partner networks, however, are generally ascertained by following individual's histories and mapping the network locally. Some technological networks, e.g., the graphs of the Internet and World Wide Web (10), and some food webs (11, 12), may in principle be fairly accurate and complete images of the real underlying networks. For some model organisms, however, protein interaction data covers <20% of the proteins known to exist in that organism (ignoring multiple isoforms due to alternative splicing, etc.). This observation poses the interesting and important question of just how representative a random subnet is for the global network (see Fig. 1). Although this question is obviously an important one, it has thus far not been addressed explicitly [with the exception of a few simulation studies, which seem to have dismissed the problem fairly quickly (13)].
Fig. 1.
Sampling process on networks: each node is picked randomly with probability p to be included in the subnet. Only the links/interactions between nodes that are both in the subnet (red) can be studied in the subnet. Only for very special cases will the sampled subnet (red) be of the same type as the overall network.
Here, we show that random subnets sampled from scale-free networks are not themselves scale-free. This finding is in marked contrast to other important network models, notably Erdös–Rényi (14) and exponential random graphs. Below, we will first outline the notion of random sampling of networks and then outline the sampling properties of random, exponential, and scale-free networks.
Random Sampling of Networks
We start with a complete and self-contained network 𝒩 of size N (where we will consider the limit N → ∞) with a given degree distribution P(k). We emphasize that the degree distribution alone does not suffice to characterize a network: very different networks, e.g., some with many cross-connections (loops) and others with “tree-like” form (no loops at all), can have the same degree distribution. The degree distribution, P(k), is, however, the most commonly studied property of a network (1, 2) (followed by the clustering coefficient and network diameter), and we therefore focus on it. Moreover, claims of scale-free-like behavior are generally based solely on assessing the degree distribution (15).
The sampling process we consider is the most parsimonious process possible: each node in 𝒩 is included in the subnet 𝒮 with probability p and left out of the subnet with probability (1 – p) (in the case of protein interaction networks, this process would, for example, correspond to testing for interactions between a subset of proteins in an organism). For finite networks, the expected size of the subnet is thus E[M] = Np with variance Var[M] = Np(1 – p). From Fig. 1, it is apparent that a network generated by such a random sampling approach can be substantially different from the overall network 𝒩.
More precisely, let the degree distribution of the net 𝒩be P(k) and of the subnet 𝒮 be P*(k). A compact and conventional presentation is obtained by defining P(k) in terms of its probability-generating function (PGF), G(s), as follows:
![]() |
[2] |
P(k) is then derived from G(s) as k!P(k) = (dkG(s)/dsk)s=0. Note that in scale-free networks, there are no unconnected or “orphan” nodes; P(0) = 0.
If the nodes in the subnet are selected at random, then the probability that a node of degree i in the full net will be connected to k other nodes (k ≤ i) in the subnet is given by the usual binomial formula, . Hence, we have
![]() |
[3] |
It follows that the PGF for the subnet, G*(s), has the simple form
![]() |
[4] |
For networks where orphaned nodes are not allowed, e.g., scale-free networks explicitly forbid the existence of k = 0, we have to renormalize the distribution of the subnet after discarding orphaned nodes that were created by the sampling process, and we obtain∥
![]() |
[5] |
(see also Supporting Text, which is published as supporting information on the PNAS web site).
It is apparent that the original PGF, Eq. 2 and that of the subnet, Eq. 4 or 5, will not in general describe similar degree distributions for the degree distribution of the sampled subnet to belong to the same family of distributions, it is required that
![]() |
[6] |
where Ω and Ω′ are parameters describing the distributions. For Eq. 6 to be the case, a necessary and sufficient condition follows from Eq. 4 (or Eq. 5) with Eq. 6, i.e.
![]() |
[7] |
The proof for this equation is given in Supporting Text.
However, P(k) and P*(k) do have the same degree distribution (although, of course, with average connectivity reduced by the sampling probability p) for positive and negative binomial degree distributions. These distributions represent a wide class of distributions, which importantly include the Erdös–Rényi (14) (alternatively called classical random or Poisson) and exponential networks.††
For scale-free distributions, Fig. 2 makes it plain that subnets do not have the same degree distribution as the full network. This finding can be seen more explicitly from Eq. 4 with P(k) having the power-law form of Eq. 1.
Fig. 2.
The power-law degree distribution for an infinitely large scale-free network (black circles) and the degree distributions (excluding k = 0) in the subnets created by choosing each node with P = 0.8 (blue triangles) and P = 0.2 (red crosses), respectively, for γ = 3(Top), 2(Middle), and 1.5 (Bottom).
Specifically, for γ = 2, we can obtain exact analytic expressions for the degree distribution P*(k), whence it can be seen that for small values of p most of the subnet nodes are orphans [P*(0) ≃ 1 – pln(e/p)]. Discarding these, we have many nodes with a single link {P*(1) ≃ ln(1/p)/[1 + ln(e/p)]}, whereas for k > 1 the degree distribution is P*(k > 1) ≃ [constant]/[k(k – 1)], which is initially less steep than, but asymptotically identical with, the original network's k–2 distribution.‡‡ For γ = 3, analytic results show a proportion of orphans that is even larger than for γ = 2 when p « 1, and, once orphans are removed, a greater proportion of nodes with one or two links, falling off for k > 2 as const./[k(k – 1)(k – 2)], which eventually asymptotes to the original k–3 power law.§§
In short, and as indicated in Fig. 2, subnets randomly sampled from a scale-free network will not themselves be strictly scale free, in contrast with random and exponential nets, where sampled subnets have the same degree distribution as their parents, although with suitably rescaled parameters. The deviation from scale-free behavior is more pronounced as the power law exponent, γ, increases. The general rule is for the subnets to have more (sometimes many more) nodes with relatively few connections, but to asymptote to the full network's power law behavior at large connectivity, k » 1. It is perhaps worth noting that these properties are observed in many real networks that have been presented as scale-free. Interestingly, the curvature of the subnets degree distribution is concave rather than the convex shape frequently observed for real networks; this observation could suggest that true networks may deviate quite substantially from the ideal set by simple scale-free models.
Discussion
In practice, most networks analyzed today offer only partial insights into the true networks. For example for protein interaction data, depending on the organism, 10–80% of proteins in the proteome have been surveyed (see http://dip.doe-mbi.ucla.edu). The process by which nodes are chosen to be analyzed may of course not conform to our assumption of independent random sampling (without replacement). If this assumption is not met, then things can be even worse; for nonrandom sampling strategies, it can be shown that even for classical random graphs the degree distribution will no longer be conserved (data not shown). Moreover, the nonconservation of the power-law degree distribution of scale-free networks under sampling also can be shown from the master equation that described the evolution of scale-free networks. The degree distribution is a function of time (and network size, which is often a proxy for time, in particular in the Barabási–Albert construction). Unless sampling reverses the sequence of events by which networks were generated, the subnet will not have a scale-free distribution.
We have seen that the deviation from a power-law-like behavior is only slight for p sufficiently close to 1 (e.g., 1 ≥ p ≳ 0.8 for γ ≳ 3). Conversely, for small p the sampled network can deviate significantly from a power law with nearly all of the nodes having low connectivity in extreme cases (p « 1). Interestingly (and rather worryingly) this pattern is seen (and often dismissed) in some putative examples of power laws. In short, for some systems the properties observed in subnets could be sufficiently similar to the same properties in the overall network. If p is known (e.g., from the ratio of network sizes in the subnet and full network), then it is possible to estimate the power-law exponent γ from the data. The size of the class of orphaned nodes, of course, also contains information about properties of the global network, 𝒩.
The theory underlying much of the literature on scale-free networks is both powerful and intuitive (1, 2). However there seems to have been a recent trend to apply the name “scale-free” to any kind of network with a fat-tailed degree distribution, without a detailed statistical assessment (16). To understand the role of networks in biology or elsewhere, it is, however, important to focus on the entire network and not just the tail; as we have seen, it is the nodes with low to medium connectivities that are most severely affected by sampling.
Supplementary Material
Author contributions: M.P.H.S., C.W., and R.M.M. designed research, performed research, and wrote the paper.
Abbreviation: PGF, probability-generating function.
Footnotes
![]() |
![]() |
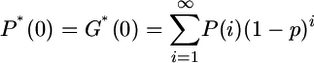
![]() |
The negative binomial distribution has the PGF G(s) = [1 + (m/k)(1 – s)]–k, where m represents the distribution`s mean value and k characterizes the distribution's “clumpiness” (the variance is given by σ2/m2 = 1/m + 1/k). This widely studied distribution includes the Poisson distribution (the degree distribution of classical random graphs) as the special case k → ∞ and the exponential or geometric as k = 1. The subnet PGF is obtained, via Eq. 4, by substituting 1 – p(1 – s) for s in G(s), to get G*(s) = [1 + (mp/k)(1 – s)]–k. Thus, the subnet has an identical PGF to the full distribution, excepting only that the mean is reduced to mp (the clumping parameter k is unaltered). The proof for the binomial distribution is even more trivial.
For Eq. 1 with γ = 2, the PGF of the subnet is , with C given by
. Defining u = 1 – p + ps, whence dG*(s)/ds = pdG*/du, we have the degree distribution given by k!P*(k) = pk(dkG*(u)/duk)u = 1 – p. For small p « 1, consider first
. This exact result gives P*(1) = –[Cpln(p)]/(1 – p). Further differentiation gives exact, but increasingly complicated, expressions for P*(k > 1). Thus, P*(2) = [Cp/(1 – p)][1 + pln(p)/(1 – p)]. For larger k, P*(k > 2) = [Cp/k(k – 1)][1 + O(p)]. Finally, we can calculate
.
![]() |
![]() |
![]() |
![]() |
References
- 1.Barabasi, A. L. & Albert, R. (1999) Science 286, 509–512. [DOI] [PubMed] [Google Scholar]
- 2.Albert, R. & Barabasi, A. L. (2002) Rev. Mod. Phys. 74, 47–97. [Google Scholar]
- 3.Newman, M. J. E. (2003) Soc. Industrial Appl. Math. Rev. 45, 167–256. [Google Scholar]
- 4.Burda, Z., Diaz-Correia, J. & Krzywicki, A. (September 24, 2001) Phys. Rev. E, 10.1103/PhysRevE.64.046118. [DOI]
- 5.Qin, H., Lu, H. S., Wu, W. B. & Li, W. H. (2003) Proc. Natl. Acad. Sci. USA 100, 12820–12824. [DOI] [PMC free article] [PubMed] [Google Scholar]
- 6.Maslov, S. & Sneppen, H. (2002) Science 296, 910–913. [DOI] [PubMed] [Google Scholar]
- 7.van Noort, V., Snel, B. & Huynen, M. (2004) EMBO Rep. 5, 280–284. [DOI] [PMC free article] [PubMed] [Google Scholar]
- 8.Jeong, H., Tombor, B. Albert, R. Oltval, Z. N. & Barabasi, A. L. (2000) Nature 407, 651–654. [DOI] [PubMed] [Google Scholar]
- 9.Newman, M. J. E & Park, J. (September 22, 2003) Phys. Rev. E, 10.1103/PhysRevE.68.036122. [DOI]
- 10.Albert, R., Jeong, H. & Barabasi, A. (1999) Nature 401, 130–131. [Google Scholar]
- 11.Girvan, M. & Newman, M. J. E. (2002) Proc. Natl. Acad. Sci. USA 99, 7821–7826. [DOI] [PMC free article] [PubMed] [Google Scholar]
- 12.Williams, R., Berlow, E., Dunne, J., Barabasi, A. & Martinez, A. (2002) Proc. Natl. Acad. Sci. USA 99, 12913–12916. [DOI] [PMC free article] [PubMed] [Google Scholar]
- 13.Yook, S. H., Oltvai, Z. N. & Barabasi, A. L. (2004) Proteomics 4, 928–942. [DOI] [PubMed] [Google Scholar]
- 14.Bollobás, B. (1998) Random Graphs (Cambridge Univ. Press, Cambridge, U.K.).
- 15.Jensen, H. J. (1998) Self-Organized Criticality (Cambridge Univ. Press, Cambridge, U.K.).
- 16.Kim, H. Kim, I., Lee, Y. & Kahng, B. (2002) J. Korean Phys. Soc. 40, 1105–1108. [Google Scholar]
Associated Data
This section collects any data citations, data availability statements, or supplementary materials included in this article.