Abstract
Post-concussive symptoms occur frequently after mild traumatic brain injury (mTBI) and may be categorized as cognitive, somatic, or emotional. We aimed to: 1) assess whether patient demographics and clinical variables predict development of each of these three symptom categories, and 2) develop a prediction model for 6-month post-concussive symptoms. Patients with mTBI (Glasgow Coma Scale score 13–15) from the prospective multi-center Transforming Research and Clinical Knowledge in Traumatic Brain Injury (TRACK-TBI) Pilot study (2010–2012) who completed the Rivermead Post Concussion Symptoms Questionnaire (RPQ) at 6 months post-injury were included. Linear regression was utilized to determine the predictive value of candidate predictors for cognitive, somatic, and emotional subscales individually, as well as the overall RPQ. The final prediction model was developed using least absolute shrinkage and selection operator shrinkage and bootstrap validation. We included 277 mTBI patients (70% male; median age 42 years). No major differences in the predictive value of our set of predictors existed for the cognitive, somatic, and emotional subscales, and therefore one prediction model for the RPQ total scale was developed. Years of education, pre-injury psychiatric disorders, and prior TBI were the strongest predictors of 6-month post-concussive symptoms. The total set of predictors explained 21% of the variance, which decreased to 14% after bootstrap validation. Demographic and clinical variables at baseline are predictive of 6-month post-concussive symptoms following mTBI; however, these variables explain less than one-fifth of the total variance in outcome. Model refinement with larger datasets, more granular variables, and objective biomarkers are needed before implementation in clinical practice.
Keywords: : post-concussion symptoms, prediction model, traumatic brain injury
Introduction
Traumatic brain injury (TBI) is a common and often debilitating injury. In the United States alone, at least 2.5 million people suffer TBIs annually, accounting for 52,000 deaths, 275,000 inpatient hospitalizations, and 1,365,000 emergency department (ED) visits.1 Approximately 70–90% of all TBI is characterized as mild TBI (mTBI), defined by a Glasgow Coma Scale (GCS) score of 13 to 15 upon admission to the ED.2 Many patients recover completely from mTBI in the ensuing weeks to months.3,4 However, in 5–30% of subjects with mTBI, neurologic, cognitive, and/or neuropsychiatric symptoms persist up to 1 year post-injury or longer.5–8 Methodologies to predict those at greatest risk of incomplete recovery are limited but are the subject of active research incorporating neuroimaging, patient demographics, and genetic polymorphisms. Data from any of these sources may portend poor recovery.9–13
Post-concussive syndrome (PCS) is a clinical term used to describe a constellation of post-traumatic symptoms, which may be divided into the domains of cognitive (forgetfulness, poor concentration, or slowed processing speed), somatic (headaches, double or blurred vision, photophobia or phonophobia, dizziness, nausea, disrupted sleep habits, or fatigue), or emotional (irritability, depression, frustration, or restlessness).14–17 The International Classification of Diseases, Tenth Revision (ICD-10) states that a diagnosis of PCS should include a head injury usually sufficiently severe to result in loss of consciousness (LOC), as well as three or more subjective symptoms present for at least 4 weeks. Symptoms should cause significant clinical impairment.18
In civilian populations, estimates suggest that roughly 10–20% of patients experience PCS within 6 months following mTBI.14 However, the complaints are non-specific and are also observed in patients with extra-cranial injuries. Because systemic injuries often coexist with neurological injuries, accurate estimates of true prevalence of PCS are difficult to ascertain. The term is not without controversy—for instance, after being included in the Diagnostic and Statistical Manual of Mental Disorders, Fourth Edition (DSM-IV) as a research diagnosis, PCS has been removed as a standalone disorder from the DSM-5 in favor of “major or mild neurocognitive disorder due to TBI.”19 In addition, there is overlap between the diagnostic criteria for PCS and post-traumatic stress disorder (PTSD),20 further complicating the diagnosis of PCS. Therefore, it has been suggested that mTBI sequelae are more accurately understood as “post-concussive symptoms” rather than PCS.5,21 Nevertheless, prior efforts to identify and create prediction models of post-concussive symptoms have relied on surveying the entire constellation of PCS rather than analyzing individual symptoms and/or domains.22–24
The Rivermead Post Concussion Symptoms Questionnaire (RPQ) is one validated metric to survey post-concussive symptoms, relying on self-report as to the presence and severity of 16 symptoms.16,17,25 It has been widely utilized to characterize outcomes and formally endorse symptomatology across the acute and chronic phases following mTBI.26–29 The RPQ is composed of individual symptom domains: cognitive deficits, somatic complaints, and emotional complaints, as described above.16 Thus, the RPQ permits separate analysis of potential predictors of post-concussive symptoms in each domain. As different domains likely reflect different etiological pathways, one hypothesis is that each domain may be differentially susceptible to patient-specific and clinical factors. Alternatively, these complaints may reflect more global processes and therefore may not demonstrate differential susceptibility. The predictors that overlap across domains (cognitive, somatic, and emotional), and the predictors specific to each domain warrant further delineation. Utilizing the prospective multicenter Transforming Research and Clinical Knowledge in Traumatic Brain Injury Pilot (TRACK-TBI Pilot) dataset,30 we investigated whether cognitive, somatic, and emotional symptoms have different predictors, and whether multivariable prediction modeling using patient demographics and clinical variables can be successfully applied to identify those at greatest risk for suffering post-concussive symptoms following mTBI.
Methods
This study was conducted and reported according to the criteria of the Transparent Reporting of a Multivariable Prediction Model for Individual Prognosis or Diagnosis (TRIPOD) statement.31
Study design
The TRACK-TBI Pilot study was a multi-center, prospective observational study conducted at three Level I trauma centers in the U.S.: San Francisco General Hospital (SFGH), University of Pittsburgh Medical Center (UPMC), and University Medical Center Brackenridge in Austin, Texas, using the National Institute of Neurological Disorders and Stroke (NINDS) TBI Common Data Elements (CDEs) version 1 (https://commondataelements.ninds.nih.gov/TBI.aspx). Eligible subjects were enrolled upon presentation to the ED through convenience sampling at all three sites between April 2010 and June 2012. Institutional Review Board approval was obtained at all sites. Informed consent was obtained for all participants prior to enrollment in the study. For participants unable to provide consent due to their injury, consent was obtained from their legally authorized representative. Participants were re-consented, if cognitively able, at later inpatient and/or outpatient study follow-up assessments. The current analysis focuses on post-concussive symptoms as measured by the RPQ; other outcome measures obtained at 6 months post-injury included the Glasgow Outcome Scale-Extended (GOS-E), Brief Symptom Inventory-18 Item, Post-Traumatic Stress Disorder Checklist-Civilian Version, Trail Making Test, and California Verbal Learning Test, Second Edition, as previously described.30
Patient selection
Inclusion criteria for the TRACK-TBI Pilot study were adult patients (age ≥16 years) presenting to one of the participating Level I trauma centers suffering external force trauma to the head with sufficient indications to triage to clinically indicated head computed tomography (CT) scan within 24 h of injury. There were no requirements for visible pathology on CT scan.30 Exclusion criteria were pregnancy, comorbid life-threatening disease, incarceration, serious psychiatric and neurologic disorders that would interfere with outcome assessment, and non-English speakers due to limitations in participation with outcome assessments. For the present study, our analysis was restricted to the subset of patients with mTBI, defined by a GCS score ≥13.
Measurements
To assess the presence/absence and severity of post-concussive symptoms, subjects completed the RPQ at 6 months following injury, in person with trained study personnel, preceded by the Galveston Orientation and Amnesia Test to assess capacity. All study personnel were trained on outcome measure administration by a single neuropsychological outcomes coordinator from UPMC. As previously described, the RPQ is a sensitive and validated assessment tool for the presence of post-concussive symptoms16,17,25–29 and is a core level NINDS TBI CDE.32 It is comprised of questions directed toward the following 16 symptoms: headache, nausea or vomiting, dizziness, sensitivity to noise, disrupted sleep, irritability, frustration, fatigue, depression, impaired memory, poor concentration, slowed thinking, blurred vision, double vision, light sensitivity, and restlessness. Each symptom is rated on a 5-point scale to assess whether the symptom has been absent, no more of a problem, or a mild, moderate, or severe problem in the 24 h prior to completing the questionnaire, compared with pre-injury. As recommended by previous research,33 the scores 0 and 1 were collapsed into a single category, scored at 0 points. This resulted in a 4-point scale with the following categories: symptom is absent or no more of a problem (0), symptom is mild (1), symptom is moderate (2), or symptom is severe (3). The total score was determined by adding up all scores 0 to 3, which results in a minimum score of 0 and a maximum score of 48. Subject responses may then be clustered into distinct neuropsychiatric domains: i) cognitive deficits (impaired memory, poor concentration, slowed thinking); ii) somatic complaints (headaches, blurred or double vision, noise sensitivity, dizziness, nausea, sleep disturbances, fatigue); and iii) psychological complaints (irritability, depression, frustration, restlessness).16
Selection of candidate predictors
A systematic literature search was performed using subheadings and text words in EMBASE and Google Scholar to identify systematic reviews and prior published prediction modeling developing studies that assessed predictors of post-concussive symptoms (or related outcomes) following mTBI (see Appendix A for the EMBASE search strategy). To maximize the potential application of a prediction model to clinical practice, candidate predictors not readily available in the ED or during initial clinical evaluation were excluded. The following were chosen as candidate predictors: age, gender, years of education, pre-injury seizures, pre-injury migraine or headache, pre-injury psychiatric disorders, blood alcohol level (BAL >80 mg/dL [U.S. legal limit]; ≤ 80 mg/dL; not measured), GCS score, CT abnormalities (present; absent), post-traumatic amnesia (PTA; present; absent; not measured), LOC (present; absent; not measured), and extracranial injury. We further included whether subjects suffered a prior TBI per self-report as a potential candidate predictor. Prior TBI was assessed using the NINDS TBI CDEs version 1,34 and classified as yes (with or without hospitalization) or no. Although not found in systematic reviews and previous prediction modeling studies, we hypothesized that deficits from repeated TBIs may be cumulative and thus may result in greater post-concussive symptoms burden. Information on candidate predictors was gathered through abstraction of medical records and from patient interviews during the index hospital visit.
Statistical analysis
Baseline characteristics of the overall study population were reported as medians and interquartile ranges (IQR) and frequencies and percentages for continuous and categorical variables, respectively. To verify whether loss to follow-up resulted in possible bias, we compared baseline characteristics of included patients with those patients who had a missing 6-month RPQ (n = 199), using the Pearson chi-squared statistic for categorical variables and the Mann-Whitney U test for continuous variables. Missing data on candidate predictors were subsequently imputed with a single imputation technique, meaning that values for the missing data points were estimated in a regression model using all other predictor variables and outcomes as independent variables.
We described the RPQ total scale and subscales (mean, SD, range), and assessed the association between the RPQ total scale and subscales and functional outcome (as measured by the GOS-E), as well as intercorrelations between scales, using the non-parametric Spearman's Rho correlation coefficient. We subsequently calculated Cronbach's alpha for the RPQ total scales and subscales as a measurement of internal consistency.
To calculate the effect of candidate predictors on the RPQ cognitive, somatic, and emotional subscales, we used univariable linear regression models with the candidate predictor of interest as independent variable and the RPQ subscale as dependent variable. To assess the adjusted effect of candidate predictors, we used multivariable linear regression models with all candidate predictors as independent variables. Unstandardized ß's and p values were reported. The ß coefficient indicates the change in outcome (points on the RPQ scale or subscale) for one unit change in the predictor variable. To enhance comparability of effect estimates for the different subscales, we additionally calculated standardized ß coefficients. A standardized ß indicates the change in outcome in standard deviations (SDs) for one SD change in the predictor variable.
To assess whether the predictor effects differed across cognitive, somatic, and emotional subscales, we tested for interaction between the predictors (summarized in the predicted values of the RPQ total scale) and the subscales. We created three rows per patient in the database: one with the cognitive outcome, one with the somatic outcome, and one with the emotional outcome. We subsequently fitted a random effects model with a random intercept for patient number, the predicted value of the total RPQ scale based on the full multivariable model, “outcome type” and an interaction between “outcome type” and predicted value.
We developed the final model by using the least absolute shrinkage and selection operator (Lasso) method. This method shrinks the ß coefficients in order to obtain less extreme ßs to enhance the external validity of a prediction model.35 Variables with ßs that are unstable are shrunk to zero and omitted from the model. It should be noted that Lasso shrinkage focuses on the overall fit rather than statistical significance of individual predictors. As a consequence, predictors with a p value >0.05 could still be included in the final model. External validity of the final model was further enhanced by performing bootstrap validation with 100 samples.
The interaction test, Lasso shrinkage, and bootstrap validation were analyzed with R (version 3.2.2.) using the lme4,36 penalized,37 and foreign38 packages. All other analyses were performed using the Statistical Package for the Social Sciences (SPSS) version 21. A p value <0.05 was considered statistically significant in all analyses.
Sensitivity analyses
Although a prediction model with a linear outcome is statistically more appealing, models with a binary outcome variable are often preferred for clinical interpretation. We therefore performed multivariable logistic regression analysis with the variables obtained after Lasso shrinkage as independent variables and the dichotomized RPQ scale as dependent variable. For the dichotomization of the RPQ, we utilized the eight symptoms mentioned in the ICD-10 criteria. Subjects were subsequently diagnosed with PCS if they meet three or more of the following symptoms: 1) headache, 2) dizziness, 3) fatigue, 4) irritability, 5) insomnia, 6) memory problems, 7) concentration issues, and 8) frustration or depression (in ICD-10 explained as reduced tolerance to stress, emotional excitement, or alcohol). It should be recognized that the RPQ cannot be used to truly diagnose ICD-10 PCS since the RPQ is based on self-report rather than clinical examination and does not include information on symptom duration and clinical significant impairment. In addition, there is no consensus as to whether symptoms should be included if they are rated as “mild symptom or worse” or if they are rated as “moderate symptom or worse.”39 We therefore applied both classifications.
We further examined the influence of attrition on estimates of the predictors by simulating three scenarios:
1. The patients lost to follow-up have relatively favorable outcomes in comparison to those included in current study.
2. The patients lost to follow-up have similar outcomes to those included in current study.
3. The patients lost to follow-up have relatively unfavorable outcomes in comparison to those included in current study.
For the first scenario, we simulated the outcome of those lost to follow-up by generating random numbers with the range 0–48 (possible scores on RPQ), a mean of 0.00 (25th percentile of those included), and a SD of 10.0 (actual SD of those included). For the second scenario, we simulated outcome of those lost to follow-up with the range 0–48, a mean of 5.0 (median of those included), and a SD of 10.0 (actual SD of those included). For the third scenario, we simulated outcome with the range 0–48, a mean of 15 (75th percentile of those included), and a SD of 10.0 (actual SD of those included). For simplicity, we did not predetermine the associations between predictors and attrition, while acknowledging that this may play a role in the influence of attrition on effect estimates.
Results
Patient characteristics
The TRACK-TBI Pilot study consisted of 580 TBI subjects, of whom 476 had mTBI (GCS 13–15); 277 subjects (58%) completed 6-month RPQ assessment and were included in the current analysis (Fig. 1). Included subjects had more years of education (median, 14) than those lost to follow-up (median, 13; p < 0.01). No other statistically significant differences existed between those included in the current analysis versus lost to follow-up (Table 1). Median age for subjects in the current analysis was 42 years (interquartile range, 26–57 years) and most (70%) were male. Half of the subjects (n = 141) sustained a traffic accident. Fifty-four percent (n = 147) reported a prior TBI, for which 88 patients were hospitalized. By ED triage, 38% were discharged home, 35% were admitted to the ICU or other monitored inpatient bed, 23% were admitted to the ward, and 4% went directly to the operating room.
FIG. 1.
Flow-chart of included patients in the current study. Figure shows patients from the Transforming Research and Clinical Knowledge in Traumatic Brain Injury (TRACK TBI) pilot study that were included in current study.
Table 1.
Baseline Characteristics of 277 Subjects Included in the Study, Compared with 199 Subjects Lost to Follow-Up
Included subjects (n = 277) | Subjects lost to follow-up (n = 199) | ||||
---|---|---|---|---|---|
Variable | Missing | N (%)‡ | Missing | N (%)‡ | p value |
Age (median, IQR range) | - | 42 (26–57) | - | 43 (27–57) | 0.66 |
Gender (Female) | - | 84 (30%) | - | 51 (26%) | 0.26 |
Years of education (median, IQR range) | 11 | 14 (12–16) | 7 | 13 (12–15) | < 0.01 |
Pre-injury seizures* | - | 30 (11%) | - | 18 (9%) | 0.52 |
Pre-injury migraine and headache | - | 36 (13%) | - | 15 (8%) | 0.06 |
Pre-injury psychiatric disorders** | - | 89 (32%) | - | 49 (25%) | 0.08 |
Prior TBI | 7 | 147 (54%) | 14 | 84 (45%) | 0.06 |
Mechanism of injury | 1 | - | 0.11 | ||
- Traffic accident | 141 (51%) | 83 (42%) | |||
- Fall | 84 (30%) | 70 (35%) | |||
- Assault | 39 (14%) | 40 (21%) | |||
- Other | 12 (5%) | 6 (2%) | |||
BAL | - | - | 0.41 | ||
- ≤ 80 mg/dL (low BAL) | 80 (29%) | 53 (27%) | |||
- > 80 mg/dL (high BAL) | 39 (14%) | 37 (19%) | |||
- Not measured | 158 (57%) | 109 (54%) | |||
GCS <15 | - | 63 (23%) | - | 56 (28%) | 0.18 |
CT abnormalities*** | - | 95 (34%) | - | 74 (37%) | .52 |
PTA | 1 | 2 | 0.38 | ||
- Yes or suspected | 173 (63%) | 112 (56%) | |||
- No | 90 (32%) | 72 (37%) | |||
- Unknown | 13 (5%) | 13 (7%) | |||
LOC | 2 | 1 | 0.58 | ||
- Yes | 190 (69%) | 132 (67%) | |||
- No | 66 (24%) | 55 (28%) | |||
- Unknown | 19 (7%) | 11 (5%) | |||
Extracranial AIS ≥3 in at least one body region | - | 36 (13%) | - | 32 (16%) | 0.34 |
ED disposition | - | - | 0.33 | ||
- Home | 105 (38%) | 62 (31%) | |||
- Hospital ward | 63 (23%) | 42 (21%) | |||
- Step-down bed or ICU | 97 (35%) | 88 (44%) | |||
- Operating room | 12 (4%) | 7 (4%) |
Values are presented as N (%) unless otherwise specified.
The p value presents results of Chi-Square test (categorical variables) or Mann-Whitney U test (continuous variables) for the differences between the included subjects and subjects that were lost to follow-up.
Includes seizures and epilepsy
Includes anxiety, depression, sleeping disorders and bipolar disorder
Includes epidural hematoma, subdural hematoma, subarachnoid hemorrhage, contusion, intracerebral hemorrhage, intraventricular hemorrhage, diffuse axonal injury, brain swelling, midline shift, cistern compression, fourth ventricle shift, and third ventricle shift
IQR, interquartile range; TBI, traumatic brain injury; BAL, blood alcohol level; GCS, Glasgow Coma Scale; CT, computed tomography; PTA, posttraumatic amnesia; LOC, loss of consciousness; AIS, Abbreviated Injury Scale; ED, emergency department; ICU, intensive care unit.
At 6 months post-injury, the mean RPQ score was 8.8 (SD = 10.0). Fifty-three percent (n = 147) reported at least three or more of the eight symptoms defined for PCS by ICD-10 as “mild or worse,” while 27% (n = 74) reported at least three of eight symptoms as “moderate or worse.”
RPQ scales
The RPQ cognitive, somatic, and emotional subscales, and the RPQ total scale all demonstrated a skewed distribution with the majority of patients having relatively lower scores (Table 2). Cronbach's alpha was >0.80 for the subscales and the total scale, indicating adequate internal consistency.40 The RPQ total scale and subscales demonstrated moderate correlation with the GOS-E at 6 months post-injury (r = −0.61 to −0.71; p < 0.01), indicating that higher RPQ scores were associated with worse functional outcome. Intercorrelations between subscales were moderate (r = 0.63 to 0.76; p < 0.01).
Table 2.
RPQ Outcome Scales 6 Months after Mild Traumatic Brain Injury
Psychometric characteristics | Correlations | |||||||||
---|---|---|---|---|---|---|---|---|---|---|
No. Items | Mean | SD | Range | Possible range | Cronbach's alpha | GOSE | RPQ Cognitive Scale | RPQ Somatic Scale | RPQ Emotional Scale | |
RPQ Cognitive Scale | 3 | 2.25 | 2.74 | 0–9 | 0–9 | 0.92 | −0.61* | - | ||
RPQ Somatic Scale | 9 | 4.32 | 5.34 | 0–27 | 0–27 | 0.85 | −0.65* | 0.63* | - | |
RPQ Emotional Scale | 4 | 2.19 | 3.07 | 0–12 | 0–12 | 0.89 | −0.64* | 0.69* | 0.76* | - |
RPQ Total Scale | 16 | 8.76 | 10.03 | 0–44 | 0–48 | 0.93 | −0.71* | 0.82* | 0.94* | 0.90* |
p < 0.01
Results are presented after collapsing the RPQ scores 0 (no problem) and 1 (no more of a problem) together.
Correlation coefficients represent non-parametric Spearman's Rho correlation coefficients.
Cognitive scale is based on the items forgetfulness, poor concentration, and taking longer to think.
Somatic scale is based on the items headache, dizziness, nausea, noise sensitivity, sleep disturbance, fatigue, blurred vision, light sensitivity, and double vision.
Emotional scale is based on the items irritability, depressed, frustrated, and restlessness.
RPQ, Rivermead Post Concussion Questionnaire; SD, standard deviation; GOSE, Glasgow Outcome Scale Extended.
Predictors of cognitive, somatic, and emotional post-concussive symptoms
The cognitive, somatic, and emotional subscales were significantly associated with years of education (p < 0.01), pre-injury psychiatric disorders (p < 0.01), and prior TBI (p < 0.01) in both univariable and multivariable linear regression analyses. Strengths of the effect sizes, illustrated with standardized ßs, were similar across the three different scales (Appendix B). In addition, age, pre-injury seizures, pre-injury migraine and headache, and CT abnormalities were significant predictors for one or more subscales (Table 3). The interaction test between the cognitive, somatic, and emotional outcome subscales and the predicted value of the RPQ total scale was not statistically significant (t = 0.54; standard error = 0.02). This indicates that although some differences exist on an individual predictor level, overall predictor effects are similar for the three subscales. Hence, one prediction model using the RPQ total scale as the outcome measure of choice could be developed from the current dataset, which comprised the next phase of our analysis.
Table 3.
Univariable and Multivariable Predictors of Cognitive, Somatic, and Emotional Post-Concussive Symptoms after 6 Months in 277 Patients with Mild Traumatic Brain Injury
RPQ – cognitive (three items) | RPQ – somatic (nine items) | RPQ – emotional (four items) | ||||
---|---|---|---|---|---|---|
Predictors | Univariable (ß, p value) | Multivariable (ß, p value) | Univariable (ß, p value) | Multivariable (ß, p value) | Univariable (ß, p value) | Multivariable (ß, p value) |
Age (/10y) | 0.15 (p = 0.09) | 0.11 (p = 0.23) | 0.30 (p = 0.10) | 0.38 (p = 0.03) | 0.02 (p = 0.83) | 0.09 (p = 0.40) |
Gender (female vs. male) | 0.39 (p = 0.27) | 0.58 (p = 0.10) | 0.74 (p = 0.29) | 0.88 (p = 0.19) | 0.05 (p = 0.90) | 0.22 (p = 0.58) |
Years of education (/y) | −0.24 (p < 0.01) | −0.23 (p < 0.01) | −0.46 (p < 0.01) | −0.39 (p < 0.01) | −0.24 (p < 0.01) | −0.21 (p < 0.01) |
Pre-injury seizures* (yes vs. no) | 1.14 (p = 0.03) | 0.47 (p = 0.36) | 1.85 (p = 0.07) | −0.001 (p = 0.99) | 1.32 (p = 0.03) | 0.44 (p = 0.46) |
Pre-injury migraine and headache (yes vs. no) | 0.86 (p = 0.08) | 0.02 (p = 0.96) | 4.58 (p < 0.01) | 2.82 (p < 0.01) | 1.50 (p = 0.01) | 0.45 (p = 0.41) |
Pre-injury psychiatric disorders** (yes vs. no) | 1.57 (p < 0.01) | 0.99 (p = 0.01) | 3.14 (p < 0.01) | 2.04 (p < 0.01) | 1.67 (p < 0.01) | 1.12 (p < 0.01) |
Prior TBI (yes vs. no) | 1.08 (p < 0.01) | 1.19 (p < 0.01) | 2.54 (p < 0.01) | 2.04 (p < 0.01) | 1.49 (p < 0.01) | 1.10 (p < 0.01) |
BAL | ||||||
-High BAL vs. low/unmeasured | −0.83 (p = 0.12) | −0.94 (p = 0.08) | 0.50 (p = 0.64) | 0.59 (p = 0.56) | 0.15 (p = 0.81) | −0.14 (p = 0.81) |
-Unmeasured BAL vs. high/low | −0.67 (p = 0.07) | −0.59 (p = 0.11) | −0.09 (p = 0.91) | −0.17 (p = 0.81) | 0.06 (p = 0.89) | −0.44 (p = 0.92) |
GCS 13 or 14 vs. GCS 15 | 0.17 (p = 0.67) | 0.25 (p = 0.53) | 0.24 (p = 0.75) | −0.14 (p = 0.85) | 0.66 (p = 0.14) | 0.52 (p = 0.25) |
CT abnormalities*** (yes vs. no) | 0.05 (p = 0.88) | 0.46 (p = 0.22) | −1.32 (p = 0.05) | −0.35 (p = 0.62) | −0.90 (p = 0.02) | −0.42 (p = 0.33) |
PTA | ||||||
- Yes vs. no/unknown | −1.20 (p = 0.13) | −1.09 (p = 0.16) | 0.74 (p = 0.63) | 1.24 (p = 0.40) | −0.02 (p = 0.99) | −0.09 (p = 0.92) |
- No vs. yes/unknown | −0.94 (p = 0.25) | −1.20 (p = 0.15) | 0.51 (p = 0.75) | 0.25 (p = 0.87) | −0.10 (p = 0.92) | −0.42 (p = 0.65) |
LOC | ||||||
- Yes vs. no/unknown | 0.36 (p = 0.59) | 0.37 (p = 0.56) | −1.18 (p = 0.36) | −1.33 (p = 0.28) | −0.12 (p = 0.87) | −0.06 (p = 0.93) |
- No vs. yes/unknown | 0.02 (p = 0.98) | 0.10 (p = 0.89) | −1.85 (p = 0.18) | −1.55 (p = 0.26) | −0.82 (p = 0.31) | −0.56 (p = 0.50) |
Extracranial AIS ≥3 in at least one Body region (yes vs. no) | −0.41 (p = 0.40) | −0.45 (p = 0.34) | −0.37 (p = 0.70) | −0.19 (p = 0.83) | −0.41 (p = 0.45) | −0.46 (p = 0.39) |
R2 | 0.20 | 0.23 | 0.17 |
Unstandardized ß's and p values are shown for all analyses. The multivariable model is based on all candidate predictors in the table.
Cognitive scale is based on the items forgetfulness, poor concentration, and taking longer to think.
Somatic scale is based on the items headache, dizziness, nausea, noise sensitivity, sleep disturbance, fatigue, blurred vision, light sensitivity, and double vision.
Emotional scale is based on the items irritability, depressed, frustrated, and restlessness.
Includes seizures and epilepsy.
Includes anxiety, depression, sleeping disorders, and bipolar disorder.
Includes epidural hematoma, subdural hematoma, subarachnoid hemorrhage, contusion, intracerebral hemorrhage, intraventricular hemorrhage, diffuse axonal injury, , brain swelling, midline shift, cistern compression, fourth ventricle shift, and third ventricle shift
RPQ, Rivermead Post Concussion Symptoms Questionnaire; TBI, traumatic brain injury; BAL, blood alcohol level; GCS, Glasgow Coma Scale; CT, computed tomography; PTA, posttraumatic amnesia; LOC, loss of consciousness; AIS, Abbreviated Injury Scale.
Prediction model of 6-month post-concussive symptoms
The RPQ total scale was significantly associated with years of education (p < 0.01), pre-injury seizures (p = 0.03), pre-injury migraine and headache (p < 0.01), pre-injury psychiatric disorders (p < 0.01), and prior TBI (p < 0.01) in univariable analyses. In a multivariable model, the variables years of education (p < 0.01), pre-injury psychiatric disorders (p < 0.01), and prior TBI (p < 0.01) were statistically significant. We applied Lasso shrinkage to obtain the final set of independent predictors and their shrunken ßs. After shrinkage, the occurrence and severity of persistent post-concussion symptoms (higher scores on the RPQ) were associated with older age, female gender, less years of education, confirmed or unknown PTA, confirmed or unknown LOC and the presence of pre-injury migraine and headache, pre-injury psychiatric disorders, and prior TBI (Table 4). Comparison of the expected values of the scales with the actual scores resulted in an R2 of 0.21, which decreased to 0.14 after bootstrap validation. The expected score on the subscales and total scale could be calculated for individual patients by using the regression formula (Table 4, footnote). An example of the calculation for two individual patients is displayed in Box 1.
Table 4.
Predictors of 6-Month Post-Concussive Syndrome in 277 Patients with Mild Traumatic Brain Injury
Predictors | Univariable (ß, p value) | Multivariable (ß, p value) | LASSO shrinkage (ß) |
---|---|---|---|
Age (/10y) | 0.50 (p = 0.16) | 0.58 (p = 0.08) | 0.53 |
Gender (female vs. male) | 1.18 (p = 0.37) | 1.68 (p = 0.19) | 0.74 |
Years of education (/y) | −0.94 (p < 0.01) | −0.84 (p < 0.01) | −0.79 |
Pre-injury seizures* (yes vs. no) | 4.30 (p = 0.03) | 0.91 (p = 0.63) | - |
Pre-injury migraine and headache (yes vs. no) | 6.95 (p < 0.01) | 3.30 (p = 0.06) | 2.07 |
Pre-injury psychiatric disorders** (yes vs. no) | 6.28 (p < 0.01) | 4.15 (p < 0.01) | 3.73 |
Prior TBI (yes vs. no) | 5.11 (p < 0.01) | 4.34 (p < 0.01) | 3.71 |
BAL | |||
-High BAL vs. low/unmeasured | −0.19 (p = 0.92) | −0.49 (p = 0.80) | - |
-Unmeasured BAL vs. high/low | −0.70 (p = 0.61) | −0.80 (p = 0.54) | - |
GCS 13 or 14 vs. GCS 15 | 1.07 (p = 0.46) | 0.62 (p = 0.66) | - |
CT abnormalities*** (yes vs. no) | −2.17 (p = 0.09) | −0.31 (p = 0.82) | - |
PTA | |||
- yes vs. no/unknown | −0.47 (p = 0.87) | 0.06 (p = 0.98) | - |
- no vs. yes/unknown | −0.53 (p = 0.86) | −1.36 (p = 0.64) | −0.47 |
LOC | |||
- yes vs. no/unknown | −0.94 (p = 0.70) | −1.02 (p = 0.66) | - |
- no vs. yes/unknown | −2.65 (p = 0.31) | −2.01 (p = 0.43) | −0.38 |
Extracranial AIS ≥3 in at least one body region (yes vs. no) | −1.20 (p = 0.51) | −1.09 (p = 0.52) | - |
R2 | 0.23 | 0.21![]() |
Unstandardized ß's and p values are shown for all analyses. The multivariable model is based on all candidate predictors in the table.
The expected 6-month Rivermead Post Concussion Symptoms Questionnaire (RPQ) score can be estimated with the following formula: 6-month RPQ = 14.45 + (0.05*Age) + (−0.79*Years of education) + (0.74*female gender) + (2.07*pre-injury migraine or headache) + (3.73*pre-injury psychiatric disorder) + (3.71*prior TBI) + (−0.47* no PTA) + (−0.38*no LOC).
R2 decreased to 0.14 after bootstrap validation with 100 samples.
Includes seizures and epilepsy.
Includes anxiety, depression, sleeping disorders, and bipolar disorder.
Includes epidural hematoma, subdural hematoma, subarachnoid hemorrhage, contusion, intracerebral hemorrhage, intraventricular hemorrhage, diffuse axonal injury, brain swelling, midline shift, cistern compression, fourth ventricle shift, and third ventricle shift.
LASSO, least absolute shrinkage and selection operator; TBI, traumatic brain injury; BAL, blood alcohol level; GCS, Glasgow Coma Scale; CT, computed tomography; PTA, posttraumatic amnesia; LOC, loss of consciousness; AIS, Abbreviated Injury Scale.
Box 1.
Two Cases and Their Predicted Score on the RPQ Scale According to Our Prediction Model
Case 1: Male patient, 65 years, 23 years of education with pre-injury headache or migraine, a pre-injury psychiatric disorder, a prior TBI, LOC, and PTA. |
Predicted value total RPQ scale after 6 months = 14.45 (intercept) + (0.74*0) + (0.05*65) + (−0.79*23) + (2.07*1) + (3.73*1) + (3.71*1) + (−0.47*0) + (−0.38*0) = 9.04 (95% CI: 4.57 – 13.50) |
Case 2: Female patient, 30 years, 10 years of education with pre-injury headache or migraine, a pre-injury psychiatric disorder and no prior TBI, LOC and PTA |
Predicted value total RPQ scale after 6 months = 14.45 (intercept) + (0.74*1) + (0.05*30) + (−0.79*10) + (2.07*1) + (3.73*1) + (3.71*0) + (−0.47*1) + (−0.38*1) = 17.45 (95% CI: 13.00 – 21.90) |
Expected scores can be calculated with the regression formula in the footnote of Table 4. The 95% Confidence interval can only be calculated with advanced statistical software.
Sensitivity analyses
Multiple logistic regression analyses with the variables obtained after Lasso shrinkage resulted in the same set of predictors being statistically significant (PCS classified as ≥3 “mild or worse” symptoms: years of education, odds ratio (OR) = 0.84, 95% CI: 0.76–0.93; pre-injury psychiatric disorders, OR = 2.05, 95% CI: 1.14–3.68; prior TBI, OR = 2.94, 95% CI: 1.71–5.08; PCS classified as ≥3 “moderate or worse” symptoms: years of education, OR = 0.87, 95% CI: 0.77–0.97; pre-injury psychiatric disorders, OR = 3.24, 95% CI: 1.77–5.91; prior TBI, OR = 2.08, 95% CI: 1.10–3.93). Female gender was a statistically significant predictor of PCS classified as ≥3 “mild or worse” symptoms (OR 2.02, 95% CI: 1.11–3.68). The areas under the curve (AUCs) ranged from 0.74 to 0.76, indicating reasonable discriminative ability (Appendix C). We did not apply further model development (e.g., shrinkage, bootstrap validation) since our sample size was too small to develop a valid model with a binary outcome.
When analyzing different scenarios of attrition, we found that the scenarios in which patients lost to follow-up had similar or more favorable outcomes did not result in major changes in effect estimates. However, in the scenario where patients lost to follow-up had relatively unfavorable outcomes, prior TBI was no longer a statistically significant predictor of 6-month post-concussive symptoms, while age, GCS, and PTA became significant predictors (Appendix D).
Discussion
We developed a prediction model to predict 6-month post-concussive symptoms following mTBI in a multi-center study with 277 subjects. Post-concussive symptoms were associated with older age, female gender, less education, pre-injury migraine or headache, pre-injury psychiatric problems, prior TBI, PTA, and LOC, of which years of education, presence of pre-injury psychiatric disorders, and prior TBI were the most robust predictors. This set of predictors accounted for less than one-fifth of the variance in post-concussive symptoms.
Previous investigations often have reported that PCS is a multi-dimensional concept.5,16, 17,33,41,42 Therefore, it has been hypothesized that the cognitive, somatic, and emotional RPQ subscales are differentially susceptible to predictor variables. We did not find a difference in the predicted probabilities of the total set of candidate predictors for the three subscales, and therefore we developed one overall prediction model for 6-month post-concussive symptoms using the RPQ total scale. This might indicate that post-concussive symptoms from different domains share etiological factors. However, we did find differences in the predictive ability for some predictors (age, pre-injury seizures, pre-injury migraine and headache, CT abnormalities) and the intercorrelations between the three subscales were modest. Therefore, confirmation of our findings in larger patient samples is necessary to confirm the adequacy of the total RPQ scale as an outcome variable in prognostic research. Our final prediction model has an R2 of 0.21, which decreased to 0.14 after bootstrap validation. This indicates that less than one-fifth of the variation in post-concussive symptoms could be explained by the predictors in the model. Despite being low for a prediction model, this is consistent with previous studies examining predictors of post-concussive symptoms using the linear RPQ as an outcome measurement. For example, in a systematic review conducted by Silverberg and colleagues,43 R2s ranged from 0.06 to 0.89 in six studies that used the RPQ as a continuous outcome measurement and was only above 0.40 in two studies deemed at high risk of statistical overfitting.43
In prior systematic reviews, the most robust predictors of mTBI sequelae were gender, pre-injury mental health, early post-injury neurological functioning, and post-injury anxiety.43,44 Consistent with this, pre-injury mental health was also a significant predictor in our study. Patients with pre-injury psychiatric disorders are known to be vulnerable to recurrence of the psychiatric disorder45 or the development of other psychopathology,46 which might be triggered by a stressful or traumatic event such as mTBI. Other significant predictors in our study were years of education and prior TBI. Both of these also were candidate predictors in the prediction model developed by Stulemeijer and colleagues24 but were not found to be statistically significant in their final model, which was confirmed by the systematic review of Silverberg and colleagues.43 Nevertheless, higher education is associated with return to work in several studies,24,47,48 and highly educated people generally have improved coping skills, cognitive and financial reserves, and a wider social network to deal with possible consequences of mTBI. The influence of prior TBI on persistent post-concussion symptoms is less often studied. However, emerging basic science and clinical research on repetitive brain injury suggests that the deleterious effects of brain injury are cumulative.49 Therefore, inclusion of a history of prior TBI is an important consideration for future work on post-concussive symptoms and other neuropsychiatric sequelae of TBI. The predictors age, gender, pre-injury migraine and headache, PTA, and LOC also appeared in our final prediction model because they contributed to the overall model fit. It however should be noted that they were not statistically significantly associated with persistent post-concussion symptoms and their potential as predictors should therefore be examined in future studies.
In creating our prediction model, we attempted to methodologically overcome several of the shortcomings of prior work. Our set of candidate predictors was based on existing literature and was appropriately limited to not exceed the rule of thumb of a maximum of one candidate predictor for every 10 cases,50,51 which limits the risk of statistical overfitting.50,52 Additionally, we used Lasso shrinkage and bootstrap validation to correct for model optimism, improving generalizability of the model.50,52 Third, we examined the influence of predictors on the three RPQ subscales and tested whether the total RPQ scale as an outcome variable was adequate. The use of the RPQ as a linear scale also might be regarded as a strength of our study. Since there is no clear cut-off point determining whether a patient should be diagnosed with PCS, dichotomization might result in an arbitrary difference between favorable and unfavorable outcome, limiting its potential for clinical practice. For example, in our study we found that two different classifications of PCS (i.e., PCS ≥3 “mild or worse” symptoms vs. PCS ≥ “moderate or worse” symptoms) resulted in a prevalence difference of 26%. Further, dichotomization results in a loss of information and potentially overly optimistic results.50 Large sample sizes are needed to prevent statistical overfitting in prognostic studies with a dichotomous outcome, especially when the prevalence of patients with the outcome of interest is relatively low. In our study, we would have needed a total of 599 patients to develop a prediction model with a binary outcome variable (PCS defined as ≥3 moderate symptoms or worse). On the other hand, models with a dichotomized outcome are clinically appealing since these models can directly estimate the risk of post-concussive symptoms. In addition, it might be more relevant for clinicians to predict a clinical significant problem (e.g., PCS) rather than predicting an increase on the RPQ scale. The latter also may necessitate clinically relevant cut-off points that are currently unavailable. To improve clinical interpretation, we created a model with a dichotomous outcome for clinical interpretation.
We note several limitations. First, there was a significant proportion of subjects lost to follow-up (42%). Although this percentage is similar to other prospective studies in mTBI research24,53,54 and patients lost to follow-up did not differ from those who remained, we cannot exclude selection bias. Patients included in our sample may, for instance, differ from those not included on factors that were not measured, or on the severity of their post-concussive symptoms. To estimate the possible effect of attrition on our estimation of predictors, we performed sensitivity analyses in which we simulated scenarios where patients lost to follow-up had a more favorable, similar, or more unfavorable outcome, compared with those included in our study. We did not find major differences in the predictive probability of our set of predictors in the scenarios where patients lost to follow-up had similar or more favorable outcomes than the included patients. This corroborated similar studies analyzing the influence of attrition on predictor estimates.55,56 However, in the scenario were patients lost to follow-up had less favorable outcomes, additional predictors were associated with post-concussive symptoms, while prior TBI, which is a strong predictor in this study, was no longer statistically significant. The effect of attrition on outcome should therefore be taken into account when interpreting the results of the current study. A second limitation is that our sample size is relatively small for the development of a prediction model.57 Consequently, our study might not have been sufficiently powered to detect the significance of some of the candidate predictors and current regression coefficients might be relatively unstable.52 Third, in the present study, the included mild TBI patients were relatively severely injured. For example, 34% of the patients had CT abnormalities, and the majority of patients had PTA and LOC. In addition, 35% of the patients were admitted to step-down beds or the ICU. The relative severity of our study population may have implications for the generalizability to other populations of mTBI patients. Given these limitations, the results of the current study should be considered preliminary; validation in an independent population is needed.
We chose to develop a model with baseline and clinical predictors that can be gathered during the ED visit to maximize the potential application of the model in clinical practice. The inclusion of post-injury characteristics may be less useful as mTBI patients may not receive routine follow-up after leaving the ED.58 However, since our model explained less than one-fifth of the variation in 6-month post-concussive symptoms, additional variables are likely necessary to obtain more reliable predictions. Since early post-injury symptoms have been shown to associate highly with chronic symptoms,43 the addition of these symptoms could substantially improve our prediction model. Ideally, two models could be developed, validated, and implemented in future ED practices. First, a model based on baseline and clinical characteristics collected at ED presentation with a high sensitivity should be developed. This model could select high-risk patients that should be seen at a follow-up appointment soon after their ED visit. Such a model could be based on current findings and could further be refined with larger datasets, more granular variables and objective biomarkers. At the follow-up appointment, early post-injury symptoms could be further investigated and added to the model. This second model could subsequently identify patients at risk for long-term sequelae, who should be prioritized for preventative or rehabilitative interventions.
Conclusion
Demographic and clinical variables at baseline predict post-concussive symptoms after mild traumatic brain injury; however, these variables explain less than one-fifth of the total variance in outcome. Model refinement with larger datasets, more granular variables, and objective biomarkers are needed before implementation in clinical practice.
Appendix A: EMBASE Search Strategy
(‘brain injury’/exp OR ‘brain injury assessment’/exp OR ‘head injury’/exp OR concussion/exp OR (((brain OR head OR crani* OR intracrani* OR skull* OR cerebr* OR capitis OR hemisphere*) NEAR/3 (injur* OR trauma* OR posttrauma* OR damag* OR lesion* OR fracture*)) OR concus* OR contus* OR neurotraum* OR tbi OR mtbi):ab,ti) AND (injury/exp OR accident/exp OR emergency/exp OR ‘emergency care’/exp OR ‘emergency ward’/exp OR violence/exp OR (trauma* OR posttrauma* OR injur* OR tbi OR mtbi OR accident* OR emergen* OR violen*):ab,ti) AND ((mild* OR minor):ti,ab OR (mtbi OR mhi):ti,ab OR (concuss* NEAR/4 (symptoms OR syndrome*)):ti,ab OR (postconcuss* OR post-concuss*):ti,ab OR ((posttraum* OR post-traum*) NEAR/2 (symptom* OR complaint*)):ti,ab) AND (‘prediction’/exp OR ‘prognosis’/exp) OR (‘prediction model’ OR ‘prognostic model’ OR ‘predictive model’):ti,ab NOT ([Conference Abstract]/lim OR [Letter]/lim OR [Note]/lim OR [Conference Paper]/lim OR [Editorial]/lim) AND [english]/lim NOT ([animals]/lim NOT [humans]/lim)
Appendix B:
Standardized Betas for Univariable and Multivariable Regression Analyses Using the Rivermead Post Concussion Questionnaire Total Scale and Subscales as Outcome Measurement
PCS – cognitive (3 items) | RPQ – somatic (9 items) | RPQ – emotional (4 items) | RPQ total scale (16 items) | |||||
---|---|---|---|---|---|---|---|---|
Predictors | Univariable (standardized ß) | Multivariable (standardized ß) | Univariable (standardized ß) | Multivariable (standardized ß) | Univariable (standardized ß) | Multivariable (standardized ß) | Univariable (standardized ß) | Multivariable (standardized ß) |
Age (/y) | 0.10 | 0.07 | 0.10 | 0.13 | 0.01 | 0.05 | 0.09 | 0.10 |
Gender (female vs. male) | 0.07 | 0.10 | 0.06 | 0.08 | 0.01 | 0.03 | 0.05 | 0.08 |
Years of education (/y) | −0.25 | −0.24 | −0.25 | −0.21 | −0.22 | −0.20 | −0.20 | −0.24 |
Pre‐injury seizures* (yes vs. no) | 0.13 | 0.05 | 0.11 | <0.01 | 0.13 | 0.04 | 0.13 | 0.03 |
Pre‐injury migraine and headache (yes vs. no) | 0.11 | <0.01 | 0.29 | 0.18 | 0.17 | 0.05 | 0.23 | 0.11 |
Pre‐injury psychiatric disorders** (yes vs. no) | 0.27 | 0.17 | 0.28 | 0.18 | 0.26 | 0.17 | 0.30 | 0.19 |
Prior TBI (yes vs. no) | 0.20 | 0.22 | 0.24 | 0.19 | 0.24 | 0.18 | 0.25 | 0.22 |
BAL | ||||||||
‐High BAL vs. low/unmeasured | −0.11 | −0.12 | 0.03 | 0.04 | 0.02 | −0.16 | −0.01 | −0.02 |
‐Unmeasured BAL vs. high/low | −0.12 | −0.11 | −0.01 | −0.02 | 0.01 | −0.08 | −0.04 | −0.04 |
GCS 13 or 14 vs. GCS 15 | 0.03 | 0.04 | 0.02 | −0.01 | 0.09 | 0.07 | 0.05 | 0.03 |
CT abnormalities*** (yes vs. no) | 0.01 | 0.08 | −0.12 | −0.03 | −0.14 | −0.07 | −0.10 | −0.02 |
PTA | ||||||||
‐ yes vs. no/unknown | −0.21 | −0.19 | 0.07 | 0.11 | <0.01 | −0.01 | −0.02 | <0.01 |
‐ no vs. yes/unknown | −0.16 | −0.21 | 0.05 | 0.02 | −0.02 | −0.06 | −0.03 | −0.04 |
LOC | ||||||||
‐ Yes vs. no/unknown | 0.06 | 0.06 | −0.10 | −0.12 | −0.02 | −0.01 | −0.04 | −0.05 |
‐ No vs. yes/unknown | <0.01 | 0.02 | −0.15 | −0.13 | −0.11 | −0.08 | −0.11 | −0.09 |
Extracranial AIS ≥3 in at least one body region (yes vs. no) | −0.05 | −0.06 | −0.02 | −0.01 | −0.05 | −0.05 | −0.04 | −0.04 |
Standardized betas are shown for all variables. The multivariable model is based on all candidate predictors in the table.
Includes seizures and epilepsy.
Includes anxiety, depression, sleeping disorders, and bipolar disorder.
Includes epidural hematoma, subdural hematoma, subarachnoid hemorrhage, contusion, intracerebral hemorrhage, intraventricular hemorrhage, diffuse axonal injury, brain swelling, midline shift, cistern compression, fourth ventricle shift, and third ventricle shift.
PCS, post‐concussive syndrome; TBI, traumatic brain injury; BAL, blood alcohol level; GCS, Glasgow coma scale; CT, computed tomography; PTA, posttraumatic amnesia; LOC, loss of consciousness; AIS, abbreviated injury score.
Appendix C:
Logistic Regression Analyses With Predictors Selected in Lasso Shrinkage as Independent Variables and the Rivermead Post Concussion Questionnaire Dichotomized Using Two Different Definitions as Dependent Variable
Variable | PCS defined as ≥3 out of 8 symptoms with score ≥2 (mild problem and worse)‡OR (95% CI) |
PCS defined as ≥3 out of 8 symptoms with score ≥3 (moderate problem and worse)![]() |
---|---|---|
Age (/10y) | 1.04 (0.89–1.22) | 1.16 (0.97–1.39) |
Gender (Female vs Male) | 2.02 (1.11–3.68) | 1.79 (0.93–3.43) |
Years of education (/y) | 0.84 (0.76–0.93) | 0.87 (0.77–0.97) |
Pre‐injury migraine & headache (yes vs. no) | 1.68 (0.70–4.07) | 1.32 (0.57–3.02) |
Pre‐injury psychiatric disorders* (yes vs. no) | 2.05 (1.14–3.68) | 3.24 (1.77–5.91) |
Prior TBI (yes vs. no) | 2.94 (1.71–5.08) | 2.08 (1.10 − 3.93) |
PTA | ||
‐ Yes vs. no/unknown | 0.60 (0.16–2.23) | 1.15 (0.28–4.69) |
‐ No vs. yes/unknown | 0.49 (0.12–1.98) | 0.77 (0.17–3.21) |
LOC | ||
‐ Yes vs. no/unknown | 1.28 (0.46–3. 59) | 0.75 (0.25–2.25) |
‐ No vs. yes/unknown | 0.92 (0.29–2.92) | 0.48 (0.13–1.78) |
AUC | 0.74 | 0.76 |
147 (53%) patients are diagnosed with PCS according to this definition.
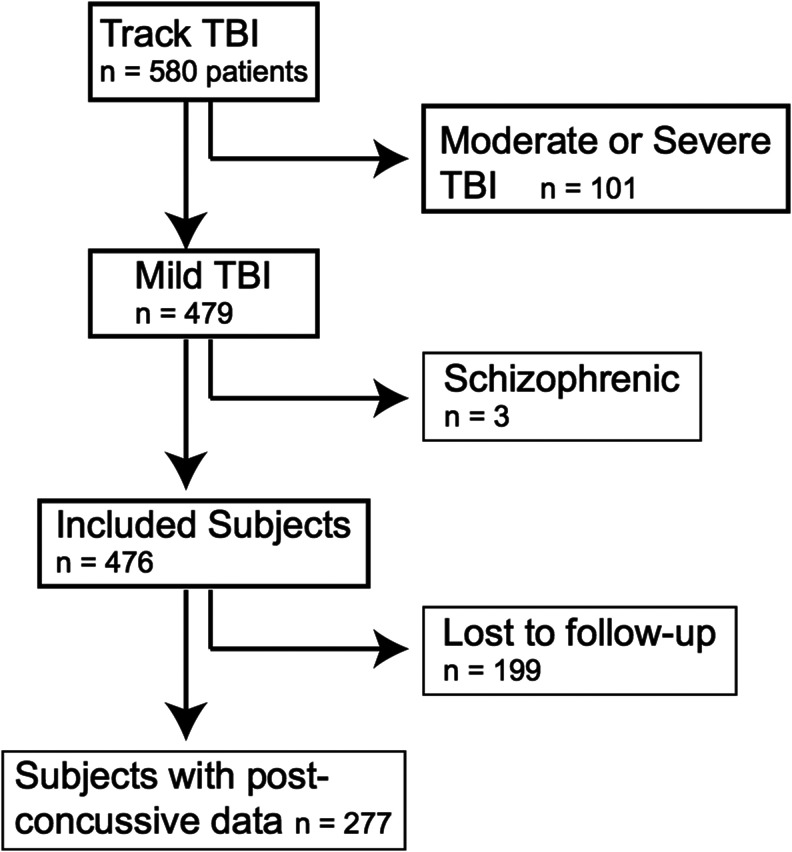
74 (27%) patients are diagnosed with PCS according to this definition.
Includes anxiety, depression, sleeping disorders, and bipolar disorder.
PCS, post‐concussive syndrome; TBI, traumatic brain injury; PTA, posttraumatic amnesia; LOC, loss of consciousness; AUC, area under the curve.
Appendix D:
The Influence of Attrition on the Estimation of Predictors
D1. Descriptions of Three Scenarios of Attrition
Scenario | N | Mean score on Rivermead Post Concussion Questionnaire (SD) |
---|---|---|
Patients lost to follow-up not included in the analyses (analyses in this paper) | 277 | 8.8 (10.0) |
Scenario 1: Patients lost to follow-up have a relatively favorable outcome‡ | 476 | 6.6 (8.8) |
Scenario 2: Patients lost to follow-up have an average outcome![]() |
476 | 7.7 (9.0) |
Scenario 3: Patients lost to follow-up have a relatively unfavorable outcome![]() |
476 | 11.8 (10.4) |
199 patients lost to follow-up received a random score on the Rivermead Post Concussion Symptoms Questionnaire with a mean of 0.0 (25th percentile patients included at 6-month follow-up) and a standard deviation of 10.0 (standard deviation patients included at 6-month follow-up).
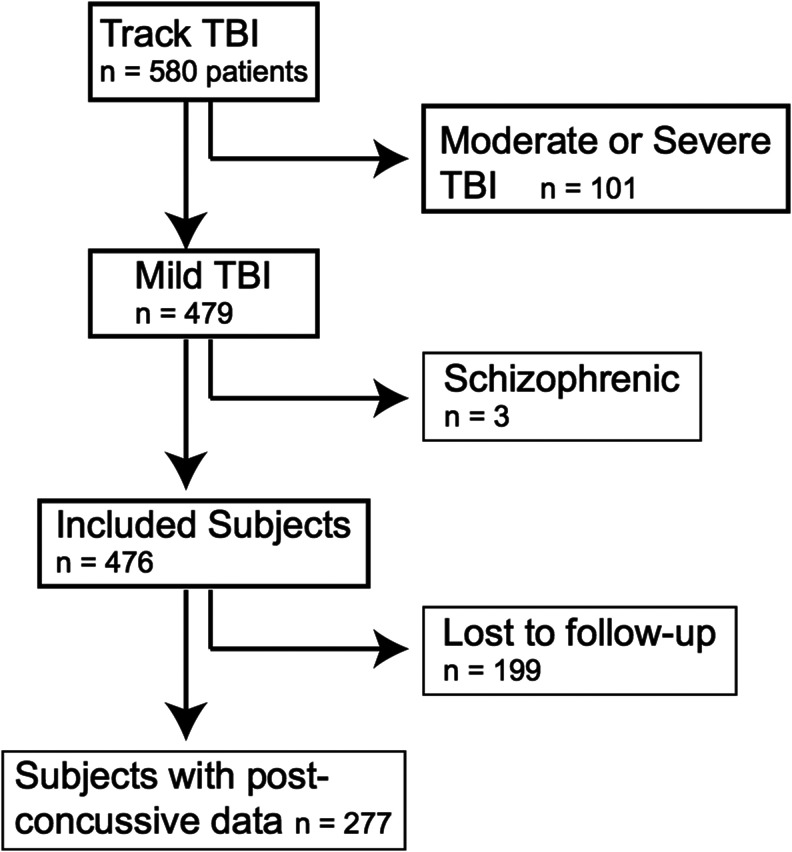
199 patients lost to follow-up received a random score on the Rivermead Post Concussion Symptoms Questionnaire with a mean of 5.0 (Median (50th percentile) at 6-month follow-up) and a standard deviation of 10.0 (standard deviation patients included at 6-month follow-up).
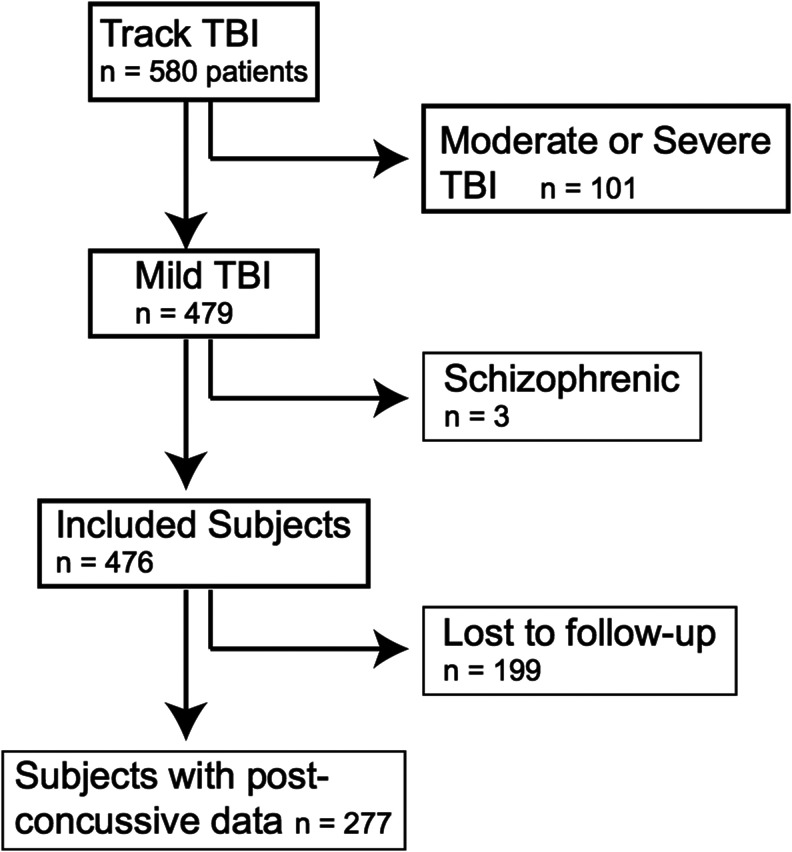
199 patients lost to follow-up received a random score on the Rivermead Post Concussion Symptoms Questionnaire with a mean of 15 (75th percentile patients included at 6-month follow-up) and a standard deviation of 10.0 (standard deviation patients included at 6-month follow-up).
D2.
Multiple Regression Analyses
Predictors | Scenario 1: Favorable outcome | Scenario 2: Average outcome | Scenario 3: Unfavorable outcome |
---|---|---|---|
Age (/y) | 0.36 (p = 0.11) | 0.43 (p = 0.06) | 0.63 (p = 0.02) |
Gender (female vs. male) | 1.10 (p = 0.22) | 1.24 (p = 0.18) | 1.21 (p = 0.27) |
Years of education (/y) | −0.27 (p = 0.05) | −0.36 (p = 0.01) | −0.76 (p < 0.01) |
Pre-injury seizures* (yes vs. no) | 1.36 (p = 0.31) | 1.47 (p = 0.29) | −0.18 (p = 0.91) |
Pre-injury migraine and headache (yes vs. no) | 3.40 (p = 0.01) | 3.28 (p = 0.02) | 1.11 (p = 0.48) |
Pre-injury psychiatric disorders** (yes vs. no) | 2.99 (p < 0.01) | 2.65 (p < 0.01) | 2.56 (p = 0.02) |
Prior TBI (yes vs. no) | 2.73 (p < 0.01) | 2.77 (p < 0.01) | 1.04 (p = 0.30) |
BAL | |||
-High BAL vs. low/unmeasured | −1.26 (p = 0.32) | −1.31 (p = 0.31) | −1.00 (p = 0.51) |
-Unmeasured BAL vs. high/low | −1.37 (p = 0.14) | −1.51 (p = 0.11) | −1.14 (p = 0.30) |
GCS 13 or 14 vs GCS 15 | −0.33 (p = 0.72) | −0.25 (p = 0.79) | 2.45 (p = 0.03) |
CT abnormalities*** (yes vs. no) | −0.85 (p = 0.35) | −1.02 (p = 0.27) | −0.75 (p = 0.49) |
PTA | |||
- Yes vs. no/unknown | 1.90 (p = 0.27) | 2.16 (p = 0.22) | 4.66 (p = 0.03) |
- No vs. yes/unknown | 1.07 (p = 0.56) | 1.35 (p = 0.48) | 3.18 (p = 0.15) |
LOC | |||
- Yes vs. no/unknown | −1.59 (p = 0.33) | −1.85 (p = 0.27) | −1.43 (p = 0.47) |
- No vs. yes/unknown | −2.28 (p = 0.21) | −2.44 (p = 0.19) | −1.95 (p = 0.37) |
Extracranial AIS ≥3 in at least one body region (yes vs. no) | −0.18 (p = 0.87) | 0.14 (p = 0.91) | 2.12 (p = 0.12) |
Includes seizures and epilepsy.
Includes anxiety, depression, sleeping disorders and bipolar disorder.
Includes epidural hematoma, subdural hematoma, subarachnoid hemorrhage, contusion, intracerebral hemorrhage, intraventricular hemorrhage, diffuse axonal injury, brain swelling, midline shift, cistern compression, fourth ventricle shift, and third ventricle shift.
PCS, post-concussive syndrome; TBI, traumatic brain injury; BAL, blood alcohol level; GCS, Glasgow coma scale; CT, computed tomography; PTA, posttraumatic amnesia; LOC, loss of consciousness; AIS, abbreviated injury score.
Contributor Information
Collaborators: the TRACK-TBI Investigators, Kristen Dams-O'Connor, Wayne A. Gordon, Allison J. Hricik, Andrew I. R. Maas, David K. Menon, Pratik Mukherjee, Ava M. Puccio, David M. Schnyer, Mary J. Vassar, and Esther L. Yuh
Acknowledgments
*TRACK-TBI Investigators: Kristen Dams-O'Connor, PhD (Department of Rehabilitation Medicine, Icahn School of Medicine at Mount Sinai, New York, NY); Wayne A. Gordon, PhD (Department of Rehabilitation Medicine, Icahn School of Medicine at Mount Sinai, New York, NY); Allison J. Hricik, MS (Department of Neurological Surgery, University of Pittsburgh Medical Center, Pittsburgh, PA); Andrew I. R. Maas, MD, PhD (Department of Neurological Surgery, University Hospital Antwerp, Antwerp, Belgium); David K. Menon, MD, PhD (Departments of Anaesthesia and Neurocritical Care, University of Cambridge, Cambridge, U.K.); Pratik Mukherjee, MD, PhD (Department of Radiology, University of California, San Francisco, San Francisco, CA); Ava M. Puccio, RN, PhD (Department of Neurological Surgery, University of Pittsburgh Medical Center, Pittsburgh, PA); David M. Schnyer, PhD (Department of Psychology, University of Texas at Austin, Austin, TX); Mary J. Vassar, RN, MS (Department of Neurological Surgery, University of California, San Francisco, San Francisco, CA); Esther L. Yuh, MD, PhD (Department of Radiology, University of California, San Francisco, San Francisco, CA)
The authors would like to thank the following contributors to the development of the TRACK-TBI database and repositories by organization and alphabetical order by last name – One Mind for Research: General Peter Chiarelli, U.S. Army (Ret.), and Garen Staglin, MBA QuesGen Systems, Inc.: Vibeke Brinck, MS, and Michael Jarrett, MBA. Thomson Reuters: Sirimon O'Charoen, PhD.
Amy J. Markowitz, JD provided editorial support. Daan Nieboer performed the bootstrap validation analyses.
This work was supported by the following grants: NINDS 1RC2NS069409-01, 3RC2NS069409-02S1, 5RC2NS069409-02, 1U01NS086090-01, 3U01NS086090-02S1, 3U01NS086090-02S2, 3U01NS086090-03S1, 5U01NS086090-02, 5U01NS086090-03; U.S. DOD W81XWH-13-1-0441, U.S. DOD W81XWH-14-2-0176 (to G.T. Manley). The authors M.C. Cnossen, H.F. Lingsma and E.W. Steyerberg were further supported by the European Union FP 7th Framework program (grant 602150).
Author Disclosure Statement
No competing financial interests exist.
References
- 1.Faul M., Xu L., Wald M.M., and Coronado V.G. (2010). Traumatic Brain Injury in the United States: Emergency Department Visits, Hospitalizations and Deaths, 2002–2006. Centers for Disease Control and Prevention, National Center for Injury: Atlanta, GA [Google Scholar]
- 2.Cassidy J.D., Carroll L.J., Peloso P.M., Borg J., von Holst H., Holm L., Kraus J., and Coronado V.G.; W. H. O. Collaborating Centre Task Force on Mild Traumatic Brain Injury (2004). Incidence, risk factors and prevention of mild traumatic brain injury: results of the WHO Collaborating Centre Task Force on Mild Traumatic Brain Injury. J. Rehabil. Med, 28–60 [DOI] [PubMed] [Google Scholar]
- 3.Carroll L.J., Cassidy J.D., Peloso P.M., Borg J., von Holst H., Holm L., Paniak C., and Pepin M. (2004). Prognosis for mild traumatic brain injury: results of the WHO Collaborating Centre Task Force on Mild Traumatic Brain Injury. J. Rehabil. Med. 84–105 [DOI] [PubMed] [Google Scholar]
- 4.McCrea M., Iverson G.L., McAllister T.W., Hammeke T.A., Powell M.R., Barr W.B., and Kelly J.P. (2009). An integrated review of recovery after mild traumatic brain injury (MTBI): implications for clinical management. Clin. Neuropsychol. 23, 1368–1390 [DOI] [PubMed] [Google Scholar]
- 5.Arciniegas D.B., Anderson C.A., Topkoff J., and McAllister T.W. (2005). Mild traumatic brain injury: a neuropsychiatric approach to diagnosis, evaluation, and treatment. Neuropsychiatric Dis. Treat. 1, 311–327 [PMC free article] [PubMed] [Google Scholar]
- 6.Boake C., McCauley S.R., Levin H.S., Pedroza C., Contant C.F., Song J.X., Brown S.A., Goodman H., Brundage S.I., and Diaz-Marchan P.J. (2005). Diagnostic criteria for postconcussional syndrome after mild to moderate traumatic brain injury. J. Neuropsychiatry Clin. Neurosci. 17, 350–356 [DOI] [PubMed] [Google Scholar]
- 7.Roe C., Sveen U., Alvsaker K., and Bautz-Holter E. (2009). Post-concussion symptoms after mild traumatic brain injury: influence of demographic factors and injury severity in a 1-year cohort study. Disabil. Rehabil. 31, 1235–1243 [DOI] [PubMed] [Google Scholar]
- 8.Dikmen S., Machamer J., and Temkin N. (2016). Mild traumatic brain injury: longitudinal study of cognition, functional status, and post-traumatic symptoms. J. Neurotrauma 34, 1524–1530 [DOI] [PMC free article] [PubMed] [Google Scholar]
- 9.Lingsma H.F., Yue J.K., Maas A.I., Steyerberg E.W., and Manley G.T. (2015). Outcome prediction after mild and complicated mild traumatic brain injury: external validation of existing models and identification of new predictors using the TRACK-TBI pilot study. J. Neurotrauma 32, 83–94 [DOI] [PMC free article] [PubMed] [Google Scholar]
- 10.Winkler E.A., Yue J.K., McAllister T.W., Temkin N.R., Oh S.S., Burchard E.G., Hu D., Ferguson A.R., Lingsma H.F., Burke J.F., Sorani M.D., Rosand J., Yuh E.L., Barber J., Tarapore P.E., Gardner R.C., Sharma S., Satris G.G., Eng C., Puccio A.M., Wang K.K., Mukherjee P., Valadka A.B., Okonkwo D.O., Diaz-Arrastia R., and Manley G.T.; TRACK-TBI Investigators. (2015). COMT Val Met polymorphism is associated with nonverbal cognition following mild traumatic brain injury. Neurogenetics. 17, 31–41 [DOI] [PMC free article] [PubMed] [Google Scholar]
- 11.Yue J.K., Pronger A.M., Ferguson A.R., Temkin N.R., Sharma S., Rosand J., Sorani M.D., McAllister T.W., Barber J., Winkler E.A., Burchard E.G., Hu D., Lingsma H.F., Cooper S.R., Puccio A.M., Okonkwo D.O., Diaz-Arrastia R., and Manley G.T. (2015). Association of a common genetic variant within ANKK1 with six-month cognitive performance after traumatic brain injury. Neurogenetics 16, 169–180 [DOI] [PMC free article] [PubMed] [Google Scholar]
- 12.Yuh E.L., Cooper S.R., Mukherjee P., Yue J.K., Lingsma H.F., Gordon W.A., Valadka A.B., Okonkwo D.O., Schnyer D.M., Vassar M.J., Maas A.I., and Manley G.T. (2014). Diffusion tensor imaging for outcome prediction in mild traumatic brain injury: a TRACK-TBI study. J. Neurotrauma 31, 1457–1477 [DOI] [PMC free article] [PubMed] [Google Scholar]
- 13.Yuh E.L., Mukherjee P., Lingsma H.F., Yue J.K., Ferguson A.R., Gordon W.A., Valadka A.B., Schnyer D.M., Okonkwo D.O., Maas A.I., and Manley G.T. (2013). Magnetic resonance imaging improves 3-month outcome prediction in mild traumatic brain injury. Ann. Neurol. 73, 224–235 [DOI] [PMC free article] [PubMed] [Google Scholar]
- 14.Broshek D.K., De Marco A.P., and Freeman J.R. (2015). A review of post-concussion syndrome and psychological factors associated with concussion. Brain Inj. 29, 228–237 [DOI] [PubMed] [Google Scholar]
- 15.Mittenberg W., DiGiulio D.V., Perrin S., and Bass A.E. (1992). Symptoms following mild head injury: expectation as aetiology. J. Neurol. Neurosurg. Psychiatry 55, 200–204 [DOI] [PMC free article] [PubMed] [Google Scholar]
- 16.Smith-Seemiller L., Fow N.R., Kant R., and Franzen M.D. (2003). Presence of post-concussion syndrome symptoms in patients with chronic pain vs mild traumatic brain injury. Brain Inj. 17, 199–206 [DOI] [PubMed] [Google Scholar]
- 17.Potter S., Leigh E., Wade D., and Fleminger S. (2006). The Rivermead Post Concussion Symptoms Questionnaire: a confirmatory factor analysis. J. Neurol. 253, 1603–1614 [DOI] [PubMed] [Google Scholar]
- 18.World Health Organization (1993). The ICD-10 Classification of Mental and Behavioural Disorders: Diagnostic Criteria for Research. World Health Organization: Geneva [Google Scholar]
- 19.American Psychiatric Association. (2013). Diagnostic and Statistical Manual of Mental Disorders, Fifth Ed. (DSM-5). Washington, D.C. [Google Scholar]
- 20.Lagarde E., Salmi L.R., Holm L.W., Contrand B., Masson F., Ribereau-Gayon R., Laborey M., and Cassidy J.D. (2014). Association of symptoms following mild traumatic brain injury with posttraumatic stress disorder vs. postconcussion syndrome. JAMA Psychiatry 71, 1032–1040 [DOI] [PubMed] [Google Scholar]
- 21.Zasler ND, Katz D., and Zafonte RD, (ed)s. (2006). Post-concussive disorder, in: Brain Injury Medicine: Principles and Practice. Demos Medical Publishing: New York, pps. 374–385 [Google Scholar]
- 22.Lange R.T., Brickell T., French L.M., Ivins B., Bhagwat A., Pancholi S., and Iverson G.L. (2013). Risk factors for postconcussion symptom reporting after traumatic brain injury in U.S. military service members. J. Neurotrauma 30, 237–246 [DOI] [PubMed] [Google Scholar]
- 23.Rabinowitz A.R., Li X., McCauley S.R., Wilde E.A., Barnes A., Hanten G., Mendez D., McCarthy J.J., and Levin H.S. (2015). Prevalence and Predictors of Poor Recovery from Mild Traumatic Brain Injury. J. Neurotrauma 32, 1488–1496 [DOI] [PMC free article] [PubMed] [Google Scholar]
- 24.Stulemeijer M., van der Werf S., Borm G.F., and Vos P.E. (2008). Early prediction of favourable recovery 6 months after mild traumatic brain injury. J. Neurol. Neurosurg. Psychiatry 79, 936–942 [DOI] [PubMed] [Google Scholar]
- 25.King N.S., Crawford S., Wenden F.J., Moss N.E., and Wade D.T. (1995). The Rivermead Post Concussion Symptoms Questionnaire: a measure of symptoms commonly experienced after head injury and its reliability. J. Neurol. 242, 587–592 [DOI] [PubMed] [Google Scholar]
- 26.Ahman S., Saveman B.I., Styrke J., Bjornstig U., and Stalnacke B.M. (2013). Long-term follow-up of patients with mild traumatic brain injury: a mixed-method study. J. Rehabil. Med. 45, 758–764 [DOI] [PubMed] [Google Scholar]
- 27.McCauley S.R., Wilde E.A., Barnes A., Hanten G., Hunter J.V., Levin H.S., and Smith D.H. (2014). Patterns of early emotional and neuropsychological sequelae after mild traumatic brain injury. J. Neurotrauma 31, 914–925 [DOI] [PMC free article] [PubMed] [Google Scholar]
- 28.Stalnacke B.M., Bjornstig U., Karlsson K., and Sojka P. (2005). One-year follow-up of mild traumatic brain injury: post-concussion symptoms, disabilities and life satisfaction in relation to serum levels of S-100B and neurone-specific enolase in acute phase. J. Rehabil. Med. 37, 300–305 [DOI] [PubMed] [Google Scholar]
- 29.Stulemeijer M., Vos P.E., Bleijenberg G., and van der Werf S.P. (2007). Cognitive complaints after mild traumatic brain injury: things are not always what they seem. J. Psychosom. Res. 63, 637–645 [DOI] [PubMed] [Google Scholar]
- 30.Yue J.K., Vassar M.J., Lingsma H.F., Cooper S.R., Okonkwo D.O., Valadka A.B., Gordon W.A., Maas A.I., Mukherjee P., Yuh E.L., Puccio A.M., Schnyer D.M., and Manley G.T.; TRACK-TBI Investigators. (2013). Transforming research and clinical knowledge in traumatic brain injury pilot: multicenter implementation of the common data elements for traumatic brain injury. J. Neurotrauma 30, 1831–1844 [DOI] [PMC free article] [PubMed] [Google Scholar]
- 31.Collins G.S., Reitsma J.B., Altman D.G., and Moons K.G.M. (2015). Transparent reporting of a multivariable prediction model for individual prognosis or diagnosis (TRIPOD): the TRIPOD statement. BMJ 350, g7594. [DOI] [PubMed] [Google Scholar]
- 32.Maas A.I., Harrison-Felix C.L., Menon D., Adelson P.D., Balkin T., Bullock R., Engel D.C., Gordon W., Orman J.L., Lew H.L., Robertson C., Temkin N., Valadka A., Verfaellie M., Wainwright M., Wright D.W., and Schwab K. (2010). Common data elements for traumatic brain injury: recommendations from the interagency working group on demographics and clinical assessment. Arch. Phys. Med. Rehabil. 91, 1641–1649 [DOI] [PubMed] [Google Scholar]
- 33.Eyres S., Carey A., Gilworth G., Neumann V., and Tennant A. (2005). Construct validity and reliability of the Rivermead Post-Concussion Symptoms Questionnaire. Clin. Rehabil. 19, 878–887 [DOI] [PubMed] [Google Scholar]
- 34.The National Institute of Neurlogical Disorders and Stroke (2015). NINDS Common Data Elements—Traumatic Brain Injury. Available at: www.commondataelements.ninds.nih.gov/tbi.aspx#tab=Data_Standards Accessed July14, 2016
- 35.Steyerberg E.W. (2009). Clinical Prediction Models. Springer Sciences and Business Media: New York [Google Scholar]
- 36.Bates D., Maechler M., Bolker B., and Walker S. (2015). Fitting linear mixed-effects models using Ime4 J. Stat. Soft. 67, 1–48 [Google Scholar]
- 37.Goeman J.J. (2010). L 1 penalized estimation in the Cox proportional hazards model. Biometric. J. 52, 80–84 [DOI] [PubMed] [Google Scholar]
- 38.R core team, B.R., Cary VJ, DebRoy S, Eglen S, Guha R, Lewin-Koh N, Myatt M, Pfaff B, Quistoff B, Warmerdam F, Weigand S. (2016). Package “foreign.” https://CRAN.R-project.org.package-foreign (last accessed May24, 2017).
- 39.Waljas M., Iverson G.L., Lange R.T., Hakulinen U., Dastidar P., Huhtala H., Liimatainen S., Hartikainen K., and Ohman J. (2015). A prospective biopsychosocial study of the persistent post-concussion symptoms following mild traumatic brain injury. J. Neurotrauma 32, 534–547 [DOI] [PubMed] [Google Scholar]
- 40.Nunnally J.C. (1978). Psychometric Theory, 2nd ed. McGraw-Hill: New York [Google Scholar]
- 41.Herrmann N., Rapoport M.J., Rajaram R.D., Chan F., Kiss A., Ma A.K., Feinstein A., McCullagh S., and Lanctôt K.L. (2009). Factor analysis of the rivermead post-concussion symptoms questionnaire in mild-to-moderate traumatic brain injury patients. J. Neuropsychiatry Clin. Neurosci. 21, 181–188 [DOI] [PubMed] [Google Scholar]
- 42.Lannsjö M., Borg J., Björklund G., Af Geijerstam J.L., and Lundgren-Nilsson A. (2011). Internal construct validity of the Rivermead Post-Concussion Symptoms Questionnaire. J. Rehabil. Med. 43, 997–1002 [DOI] [PubMed] [Google Scholar]
- 43.Silverberg N.D., Gardner A.J., Brubacher J.R., Panenka W.J., Li J.J., and Iverson G.L. (2015). Systematic review of multivariable prognostic models for mild traumatic brain injury. J. Neurotrauma 32, 517–526 [DOI] [PubMed] [Google Scholar]
- 44.Cassidy J.D., Cancelliere C., Carroll L.J., Cote P., Hincapie C.A., Holm L.W., Hartvigsen J., Donovan J., Nygren-de Boussard C., Kristman V.L., and Borg J. (2014). Systematic review of self-reported prognosis in adults after mild traumatic brain injury: results of the International Collaboration on Mild Traumatic Brain Injury Prognosis. Arch. Phys. Med. Rehabil. 95, S132–151 [DOI] [PubMed] [Google Scholar]
- 45.Mueller T.I., Leon A.C., Keller M.B., Solomon D.A., Endicott J., Coryell W., Warshaw M., and Maser J.D. (1999). Recurrence after recovery from major depressive disorder during 15 years of observational follow-up. Am. J. Psychiatry 156, 1000–1006 [DOI] [PubMed] [Google Scholar]
- 46.Zimmerli M., Tisljar K., Balestra G.M., Langewitz W., Marsch S., and Hunziker S. (2014). Prevalence and risk factors for post-traumatic stress disorder in relatives of out-of-hospital cardiac arrest patients. Resuscitation 85, 801–808 [DOI] [PubMed] [Google Scholar]
- 47.Clay F.J., Newstead S.V., Watson W.L., and McClure R.J. (2010). Determinants of return to work following non life threatening acute orthopaedic trauma: a prospective cohort study. J. Rehabil. Med. 42, 162–169 [DOI] [PubMed] [Google Scholar]
- 48.Hess D.W., Ripley D.L., McKinley W.O., and Tewksbury M. (2000). Predictors for return to work after spinal cord injury: A 3-year multicenter analysis. Arch. Phys. Med. Rehabil. 81, 359–363 [DOI] [PubMed] [Google Scholar]
- 49.Luo J., Nguyen A., Villeda S., Zhang H., Ding Z., Lindsey D., Bieri G., Castellano J.M., Beaupre G.S., and Wyss-Coray T. (2014). Long-term cognitive impairments and pathological alterations in a mouse model of repetitive mild traumatic brain injury. Front. Neurol. 5, 12. [DOI] [PMC free article] [PubMed] [Google Scholar]
- 50.Babyak M.A. (2004). What you see may not be what you get: A brief, nontechnical introduction to overfitting in regression-type models. Psychosom. Med. 66, 411–421 [DOI] [PubMed] [Google Scholar]
- 51.Bouwmeester W., Zuithoff N.P., Mallett S., Geerlings M.I., Vergouwe Y., Steyerberg E.W., Altman D.G., and Moons K.G. (2012). Reporting and methods in clinical prediction research: a systematic review. PLoS Med. 9, 1–12 [DOI] [PMC free article] [PubMed] [Google Scholar]
- 52.Hukkelhoven C.W., Rampen A.J., Maas A.I., Farace E., Habbema J.D., Marmarou A., Marshall L.F., Murray G.D., and Steyerberg E.W. (2006). Some prognostic models for traumatic brain injury were not valid. J. Clin. Epidemiol. 59, 132–143 [DOI] [PubMed] [Google Scholar]
- 53.Hou R., Moss-Morris R., Peveler R., Mogg K., Bradley B.P., and Belli A. (2012). When a minor head injury results in enduring symptoms: a prospective investigation of risk factors for postconcussional syndrome after mild traumatic brain injury. J. Neurol. Neurosurg. Psychiatry 83, 217–223 [DOI] [PubMed] [Google Scholar]
- 54.Dischinger P.C., Ryb G.E., Kufera J.A., and Auman K.M. (2009). Early predictors of postconcussive syndrome in a population of trauma patients with mild traumatic brain injury. J. Trauma 66, 289–296 [DOI] [PubMed] [Google Scholar]
- 55.Wolke D., Waylen A., Samara M., Steer C., Goodman R., Ford T., and Lamberts K. (2009). Selective drop-out in longitudinal studies and non-biased prediction of behaviour disorders. Br. J. Psychiatry 195, 249–256 [DOI] [PMC free article] [PubMed] [Google Scholar]
- 56.Gustavson K., von Soest T., Karevold E., and Roysamb E. (2012). Attrition and generalizability in longitudinal studies: findings from a 15-year population-based study and a Monte Carlo simulation study. BMC Public Health 12, 918. [DOI] [PMC free article] [PubMed] [Google Scholar]
- 57.Mushkudiani N.A., Hukkelhoven C.W., Hernandez A.V., Murray G.D., Choi S.C., Maas A.I., and Steyerberg E.W. (2008). A systematic review finds methodological improvements necessary for prognostic models in determining traumatic brain injury outcomes. J. Clin. Epidemiol. 61, 331–343 [DOI] [PubMed] [Google Scholar]
- 58.Crandall M., Rink R.A., Shaheen A.W., Butler B., Unger E., and Zollman F.S. (2014). Patterns and predictors of follow-up in patients with mild traumatic brain injury. Brain Inj. 28, 1359–1364 [DOI] [PubMed] [Google Scholar]