Abstract
OBJECTIVE: To evaluate the predictive accuracy of the Diagnostic Cost Group (DCG) model using health survey information. DATA SOURCES/STUDY SETTING: Longitudinal data collected for a sample of members of a Dutch sickness fund. In the Netherlands the sickness funds provide compulsory health insurance coverage for the 60 percent of the population in the lowest income brackets. STUDY DESIGN: A demographic model and DCG capitation models are estimated by means of ordinary least squares, with an individual's annual healthcare expenditures in 1994 as the dependent variable. For subgroups based on health survey information, costs predicted by the models are compared with actual costs. Using stepwise regression procedures a subset of relevant survey variables that could improve the predictive accuracy of the three-year DCG model was identified. Capitation models were extended with these variables. DATA COLLECTION/EXTRACTION METHODS: For the empirical analysis, panel data of sickness fund members were used that contained demographic information, annual healthcare expenditures, and diagnostic information from hospitalizations for each member. In 1993, a mailed health survey was conducted among a random sample of 15,000 persons in the panel data set, with a 70 percent response rate. PRINCIPAL FINDINGS: The predictive accuracy of the demographic model improves when it is extended with diagnostic information from prior hospitalizations (DCGs). A subset of survey variables further improves the predictive accuracy of the DCG capitation models. The predictable profits and losses based on survey information for the DCG models are smaller than for the demographic model. Most persons with predictable losses based on health survey information were not hospitalized in the preceding year. CONCLUSIONS: The use of diagnostic information from prior hospitalizations is a promising option for improving the demographic capitation payment formula. This study suggests that diagnostic information from outpatient utilization is complementary to DCGs in predicting future costs.
Full text
PDF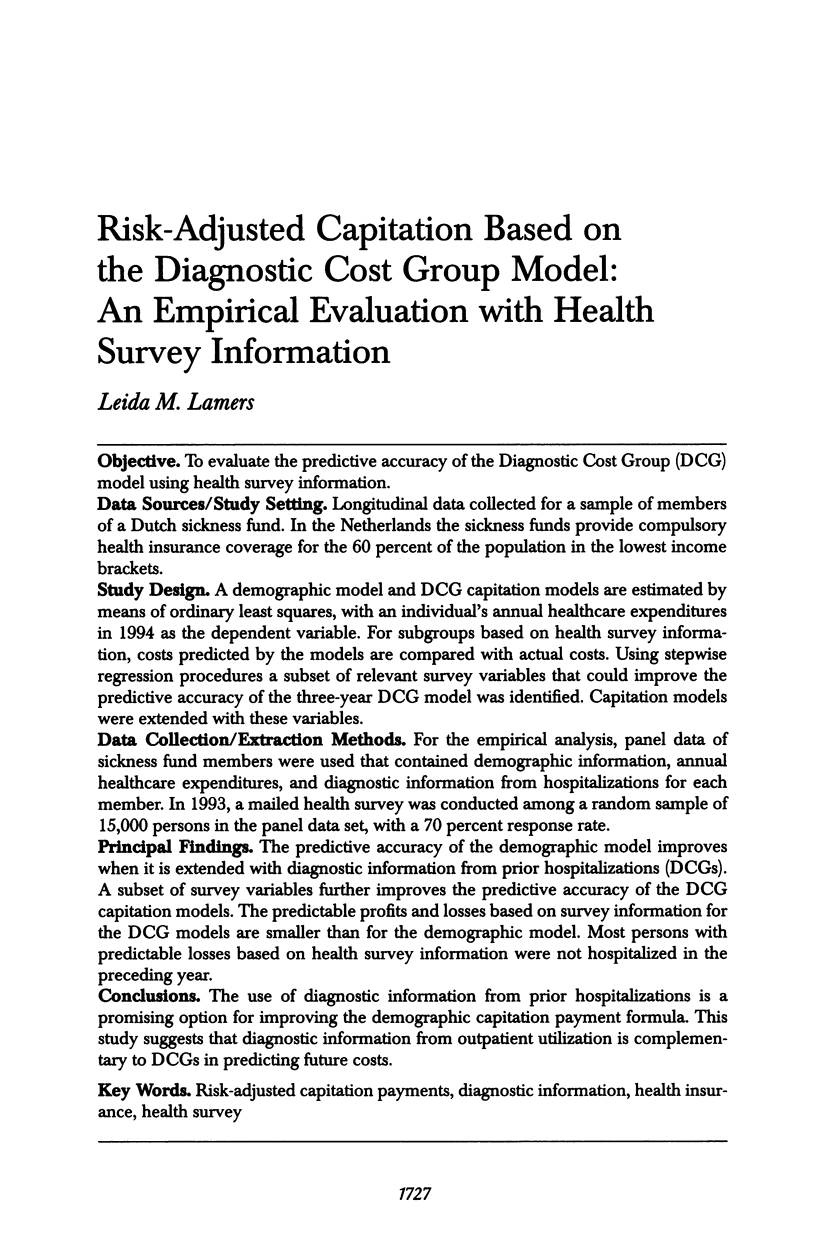
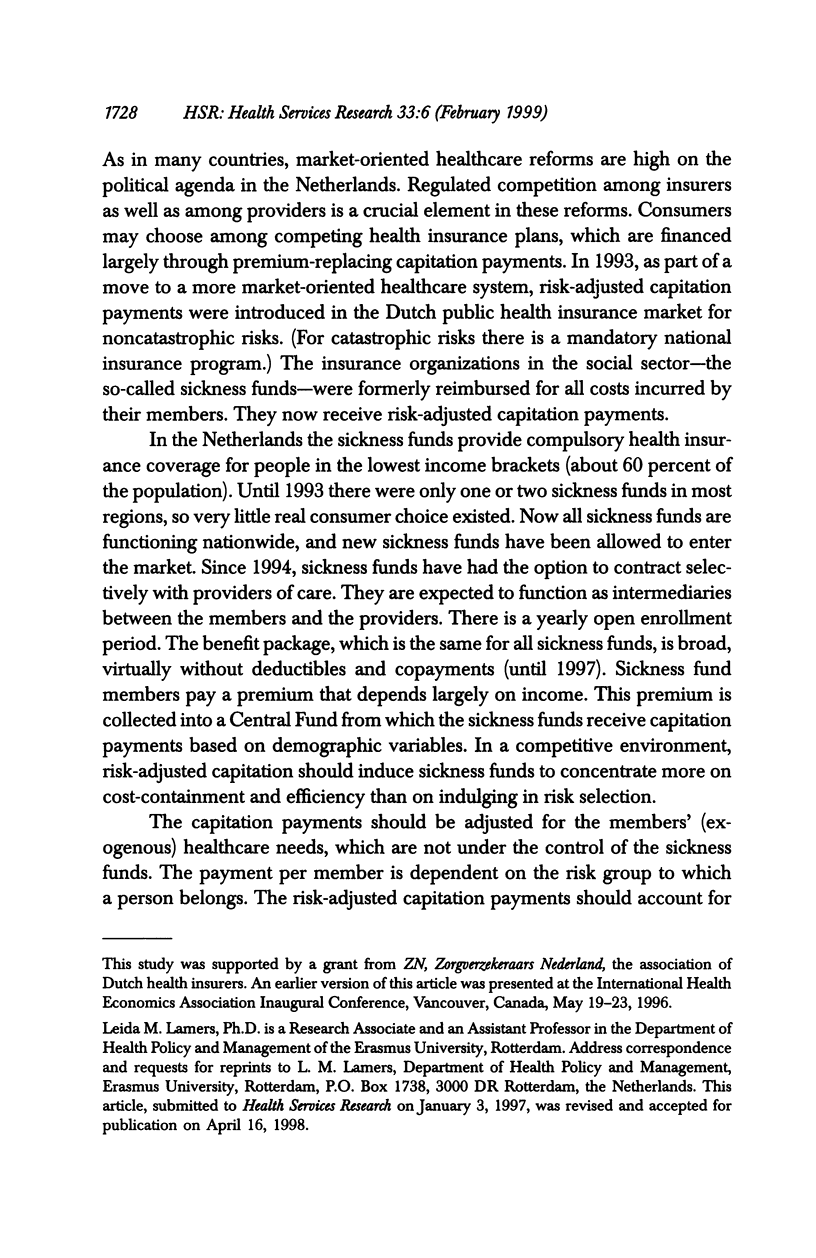
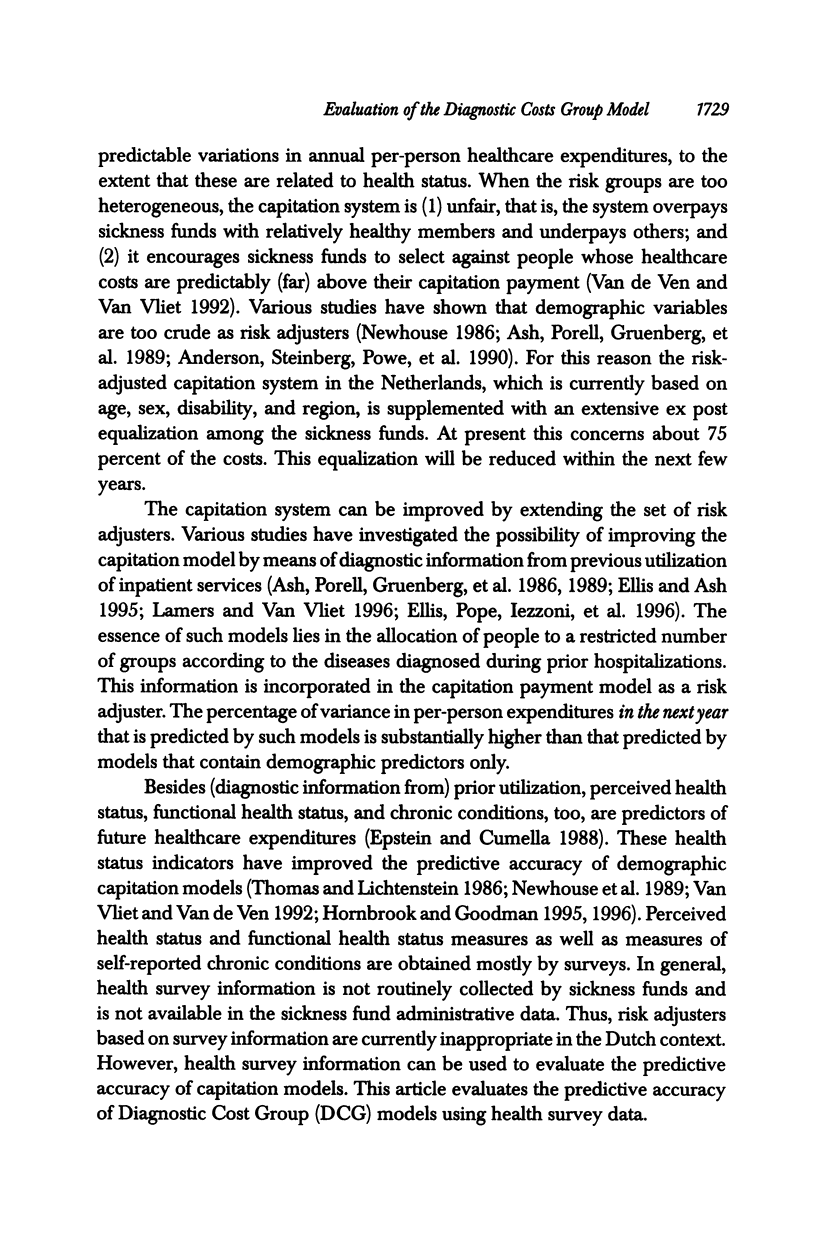
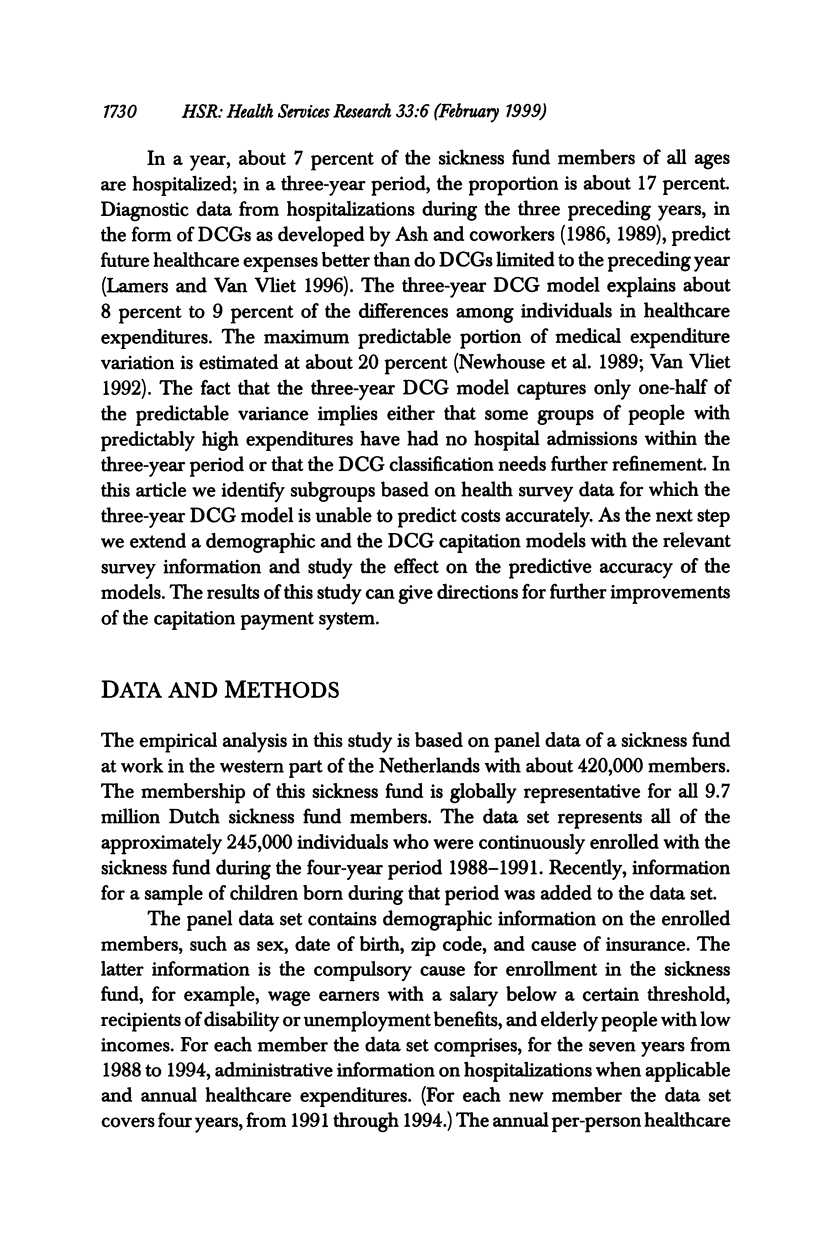
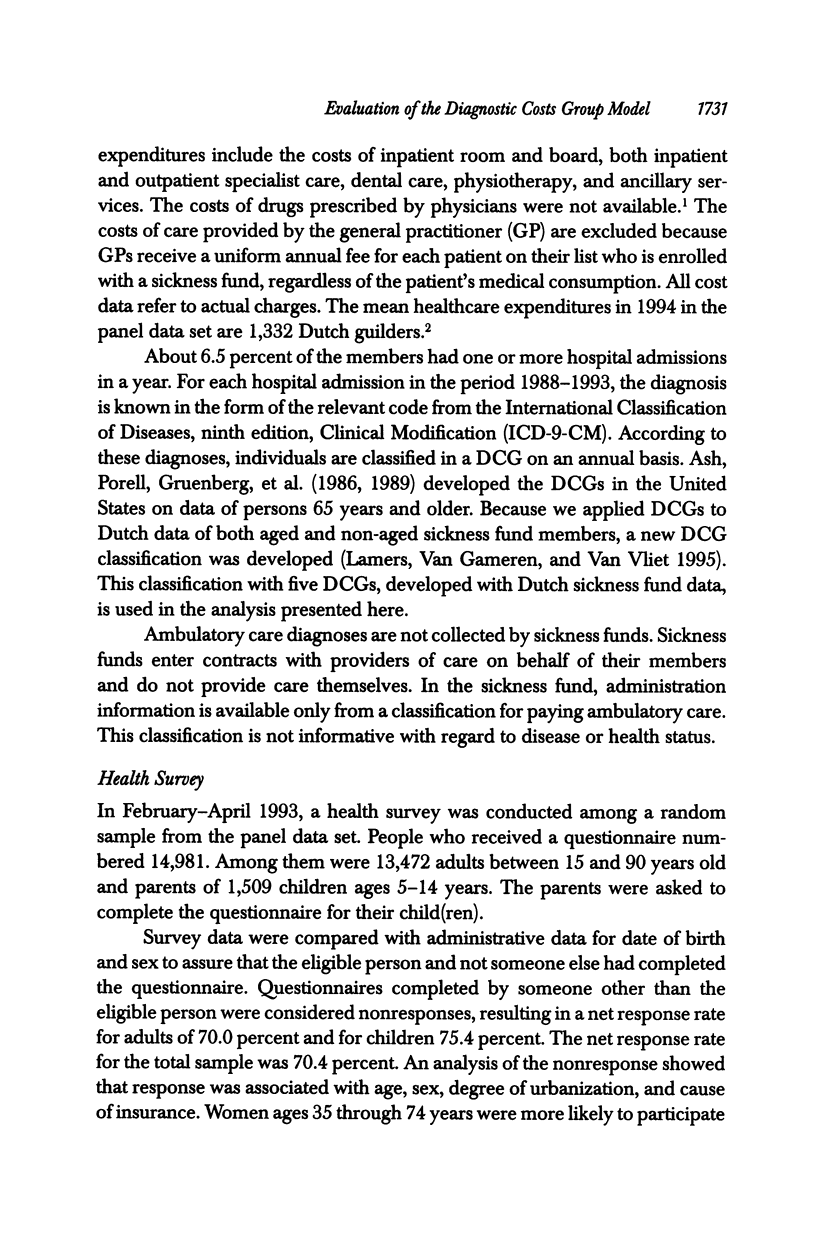
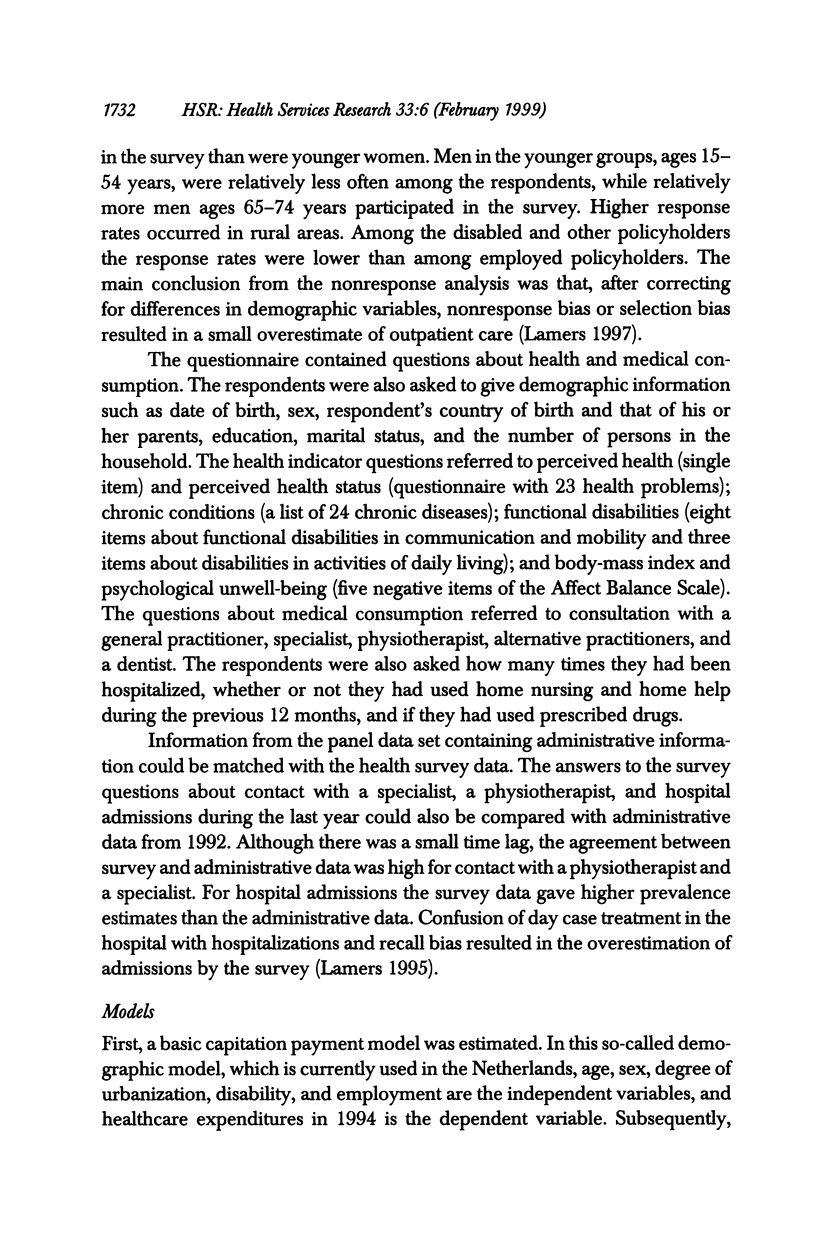
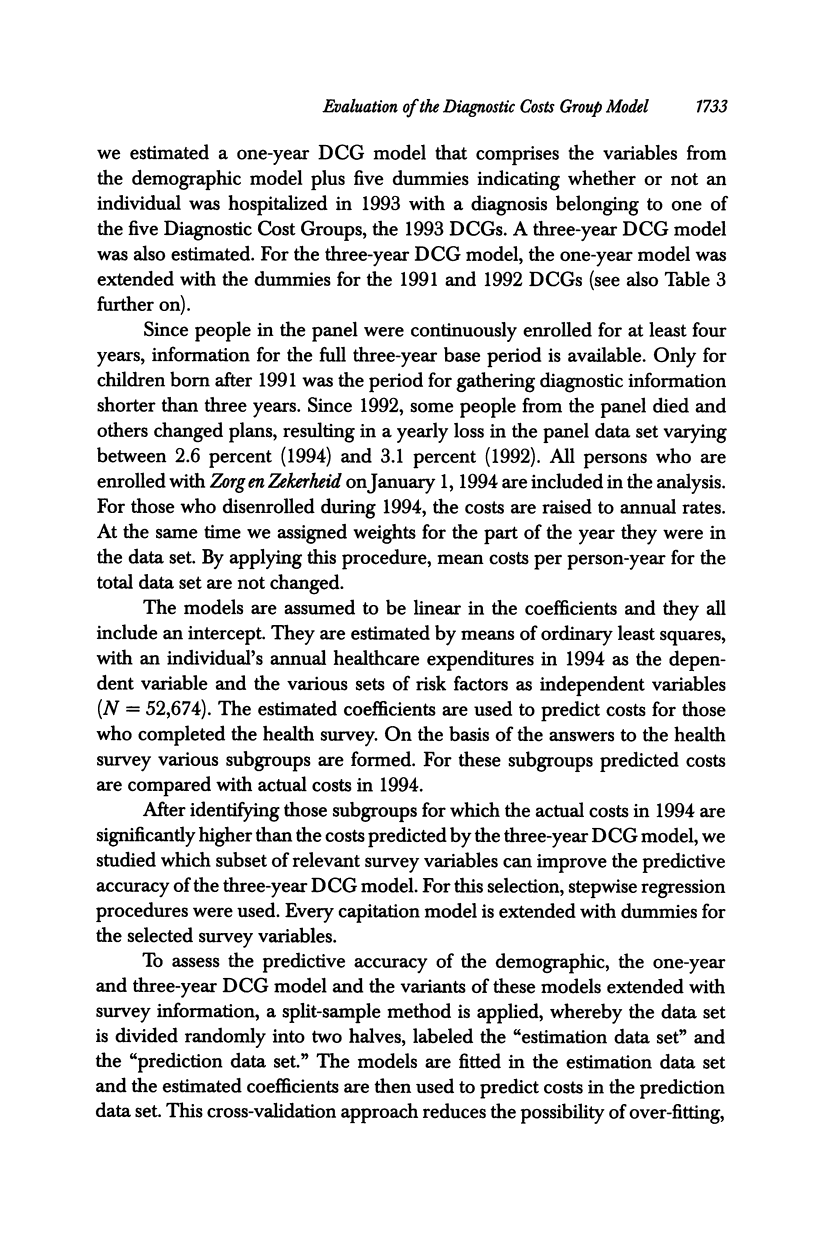
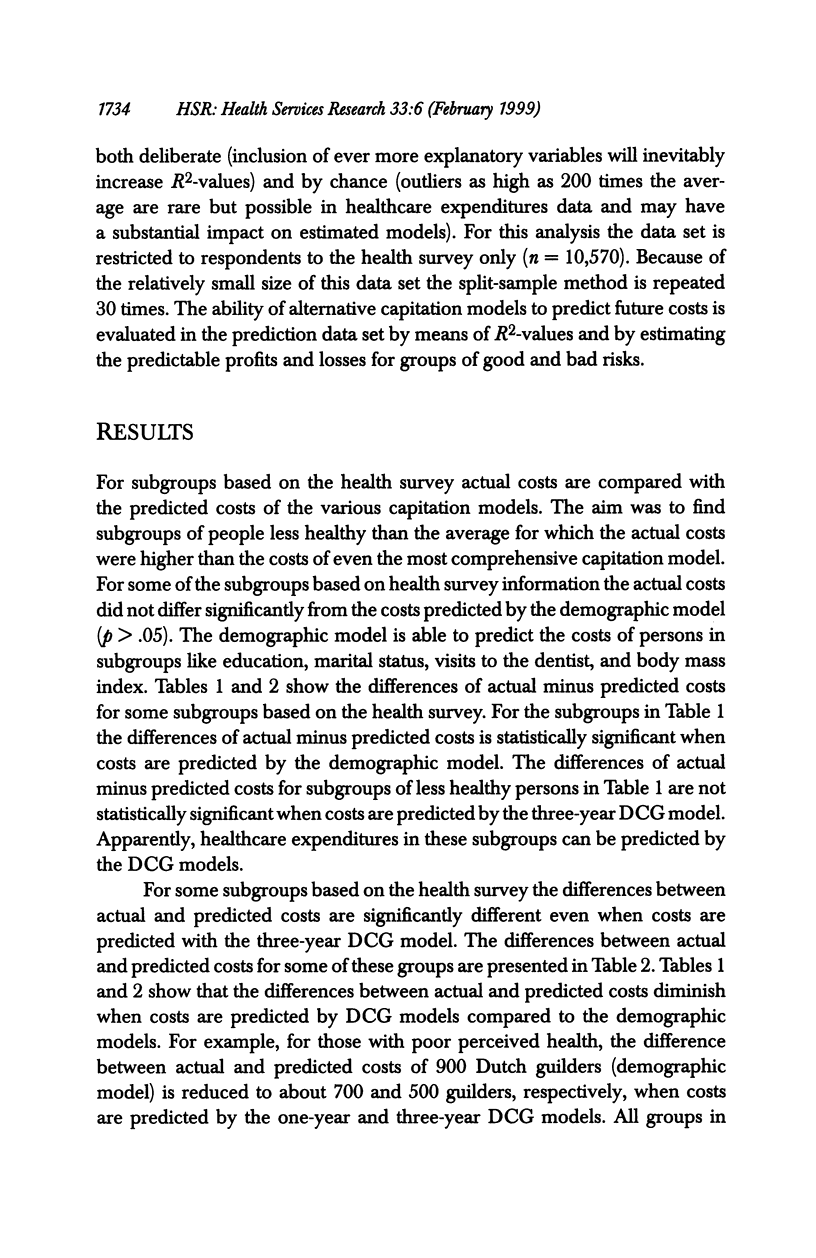
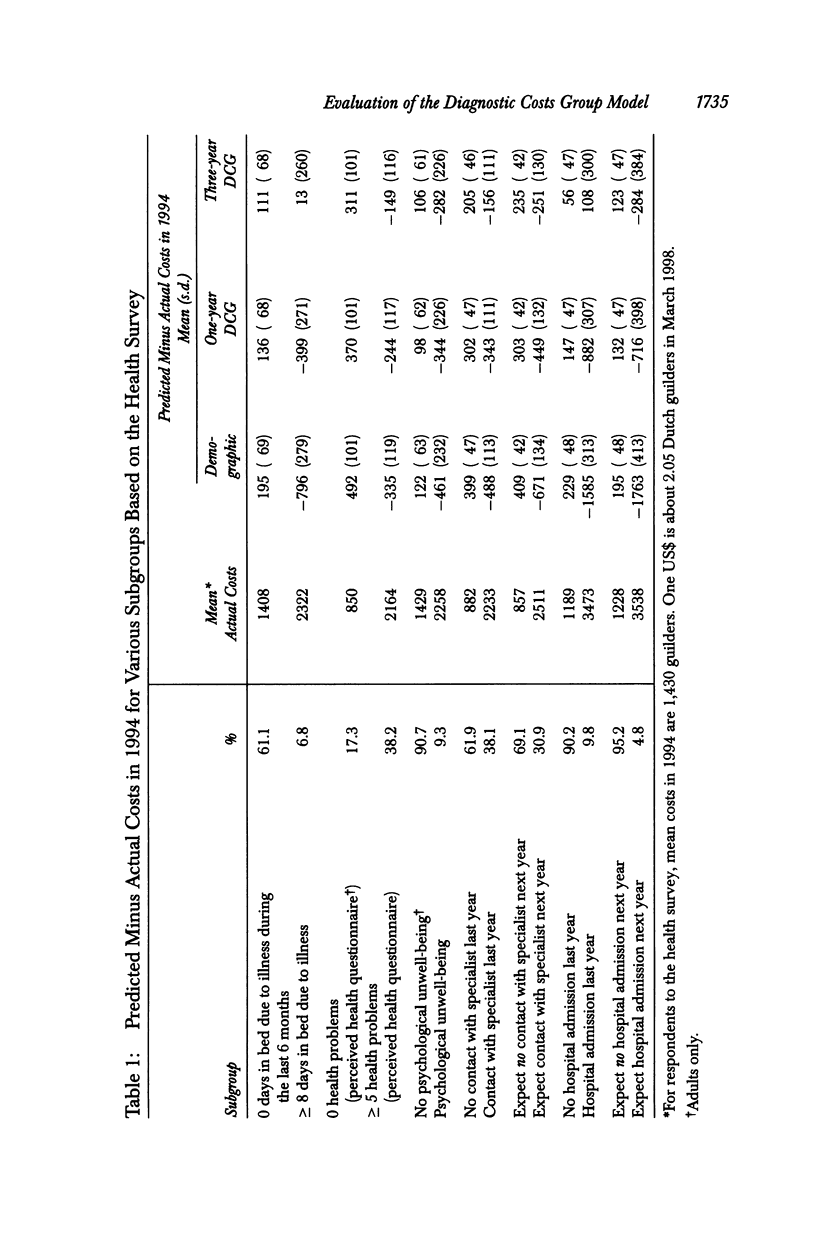
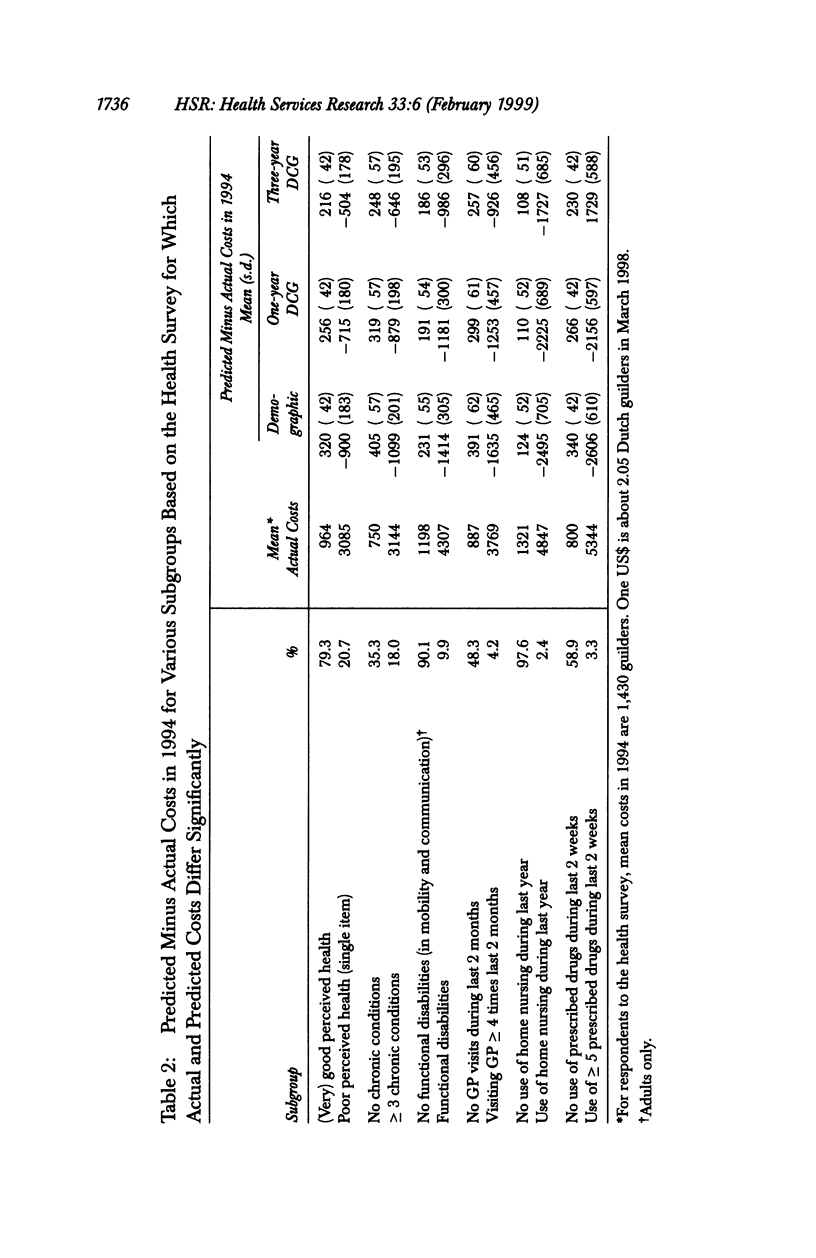
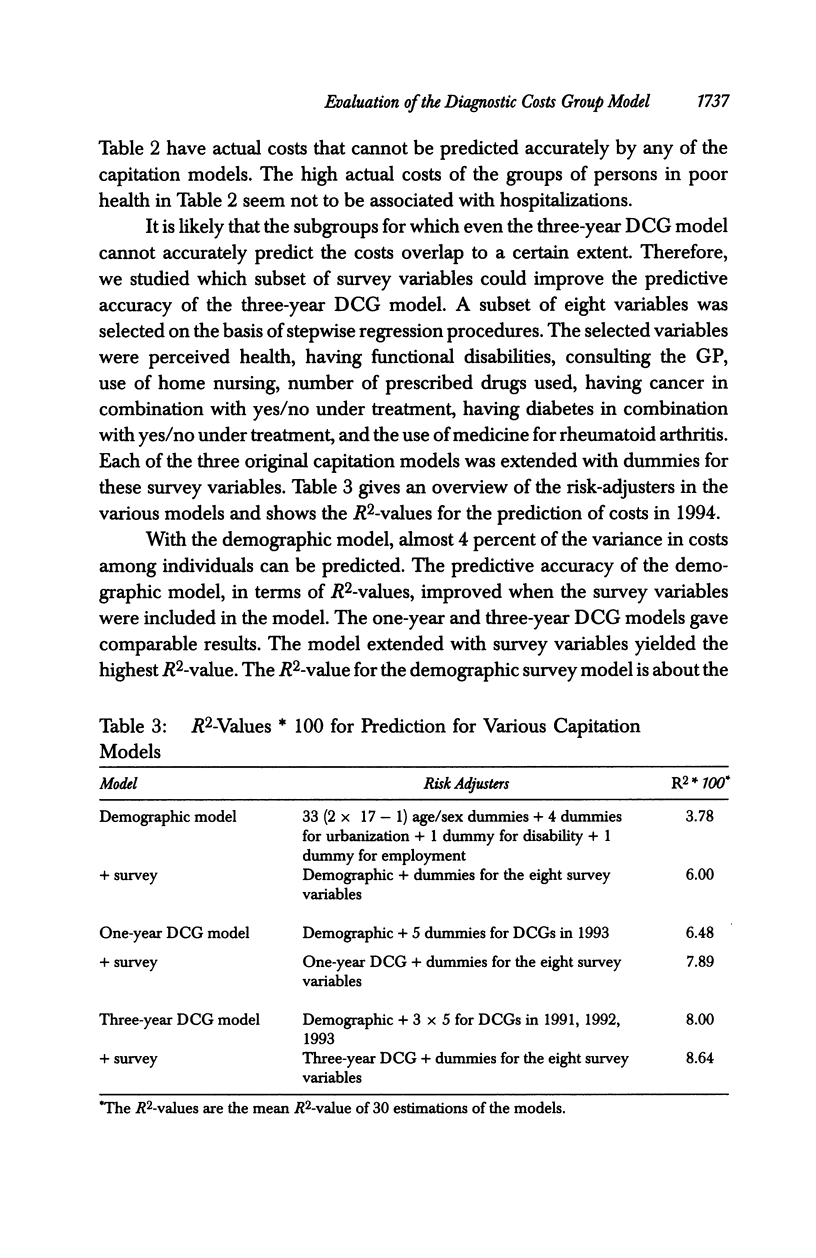
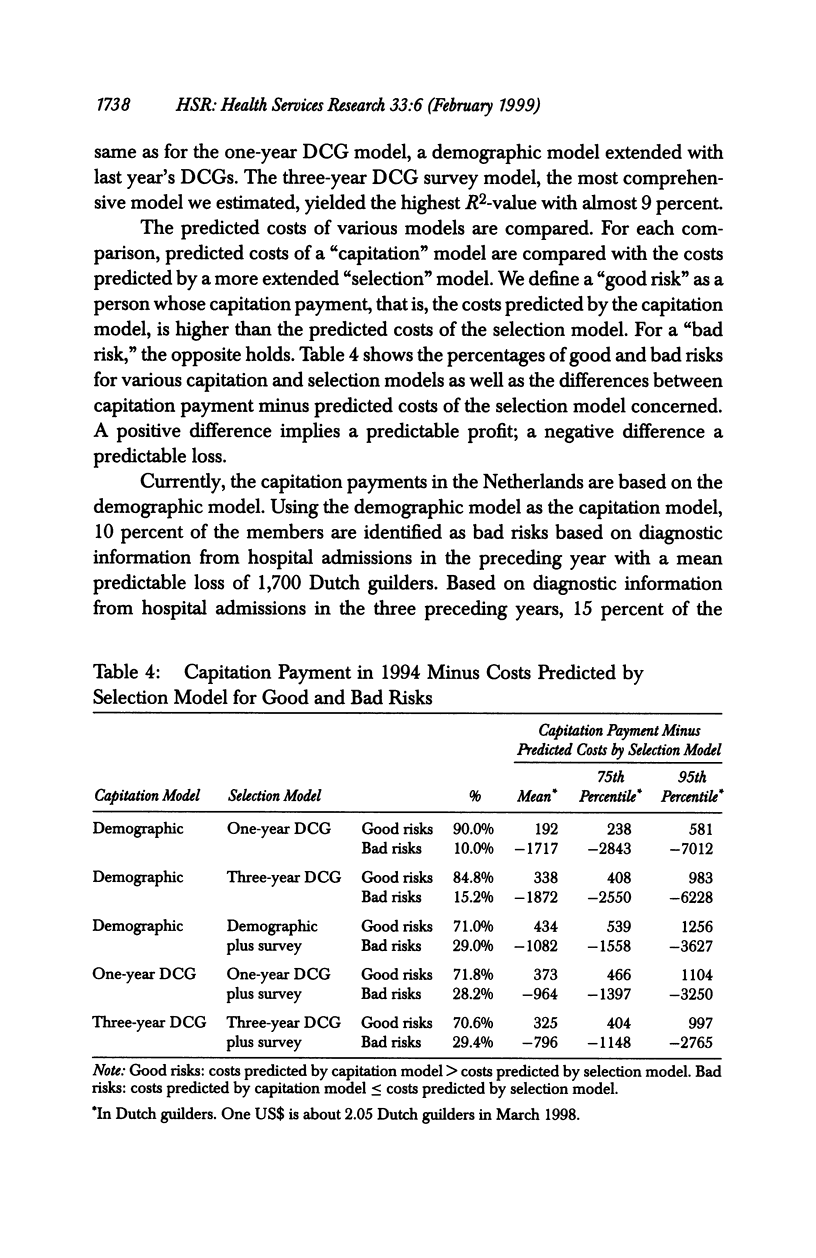
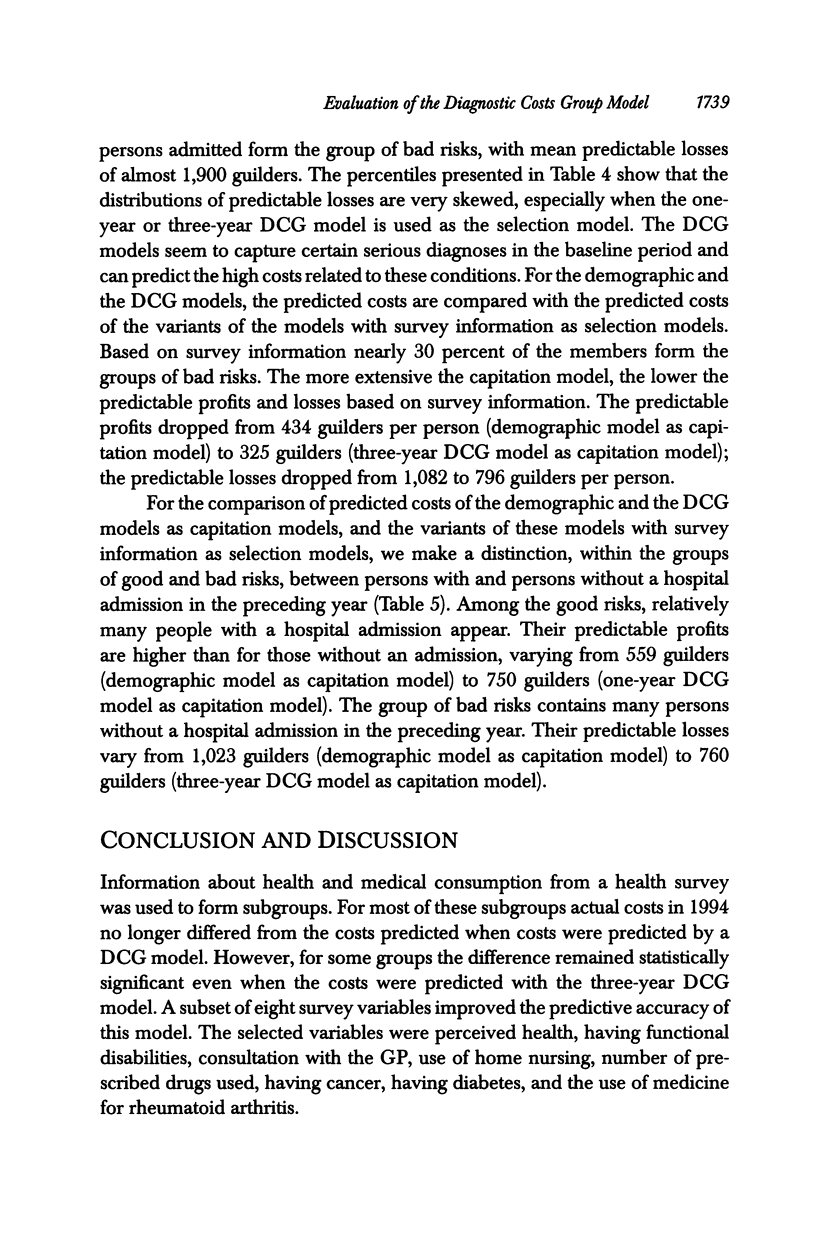
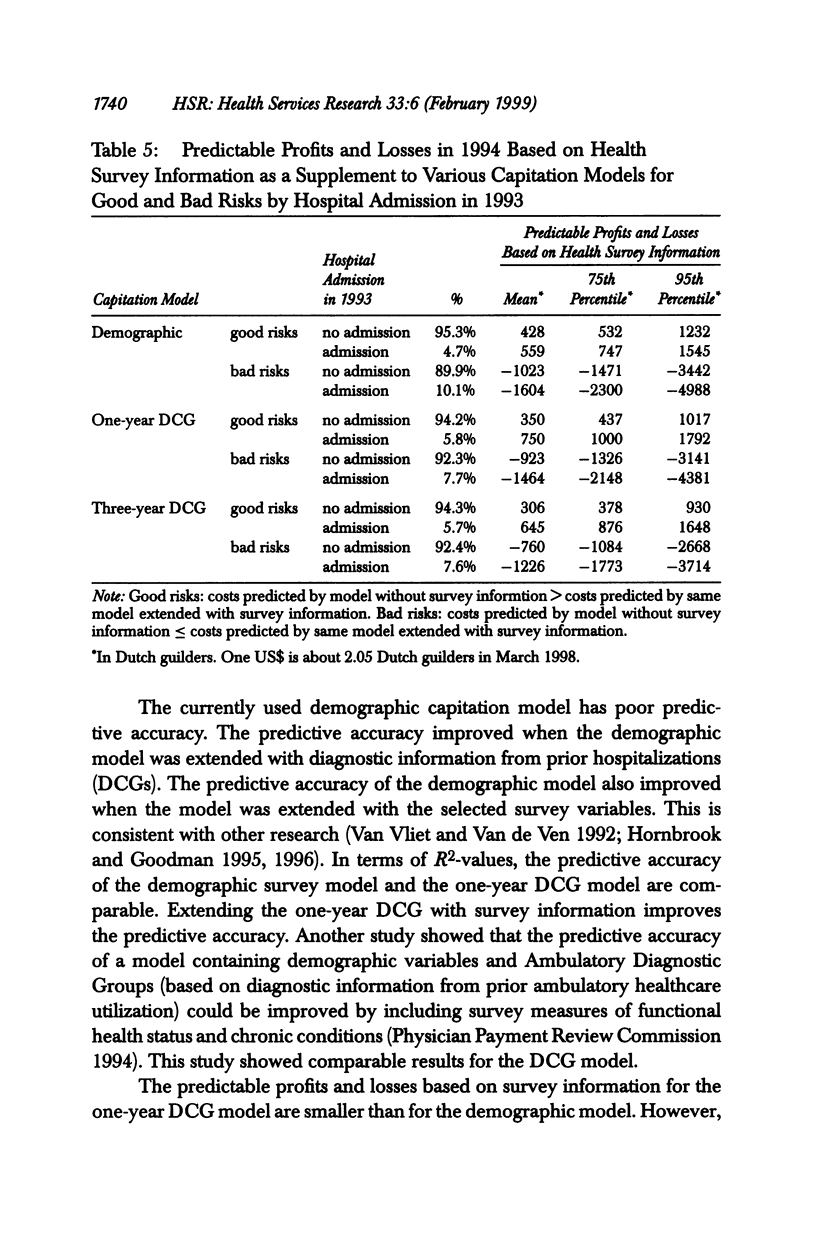
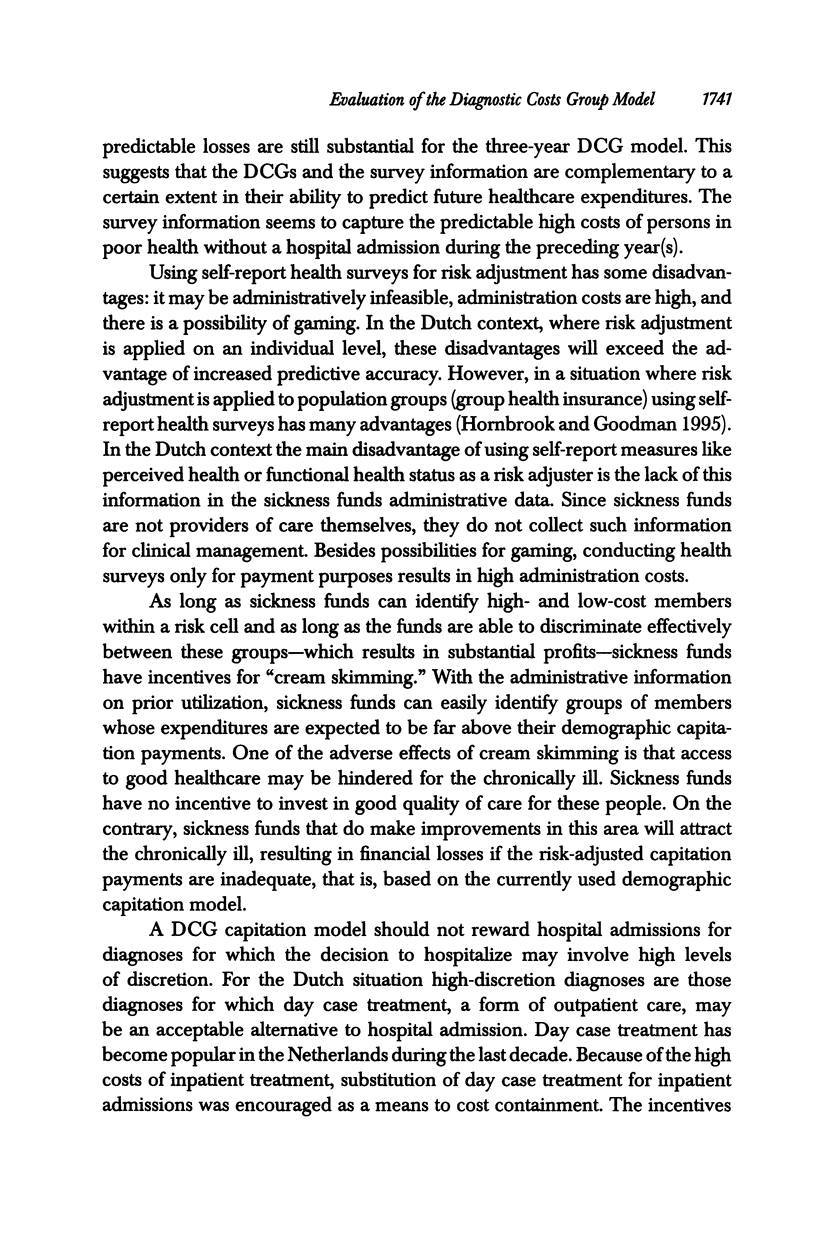
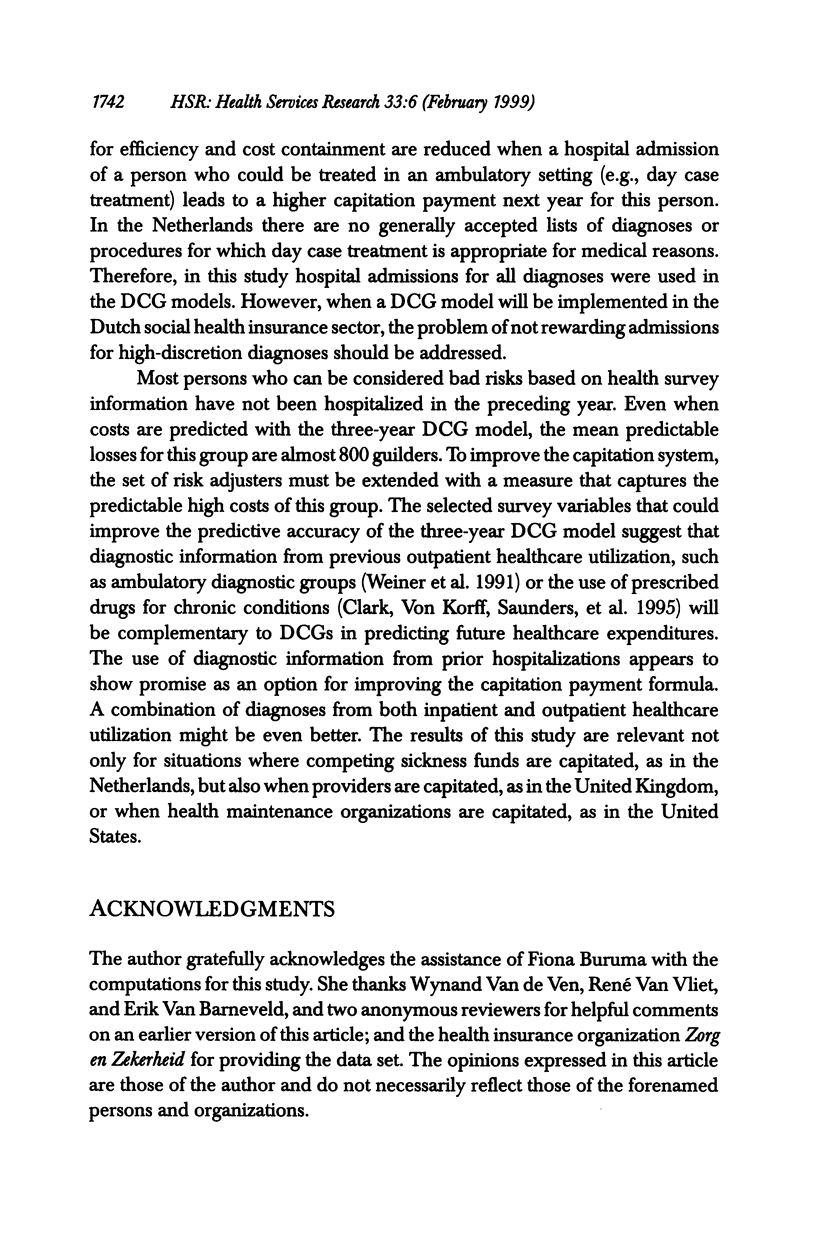
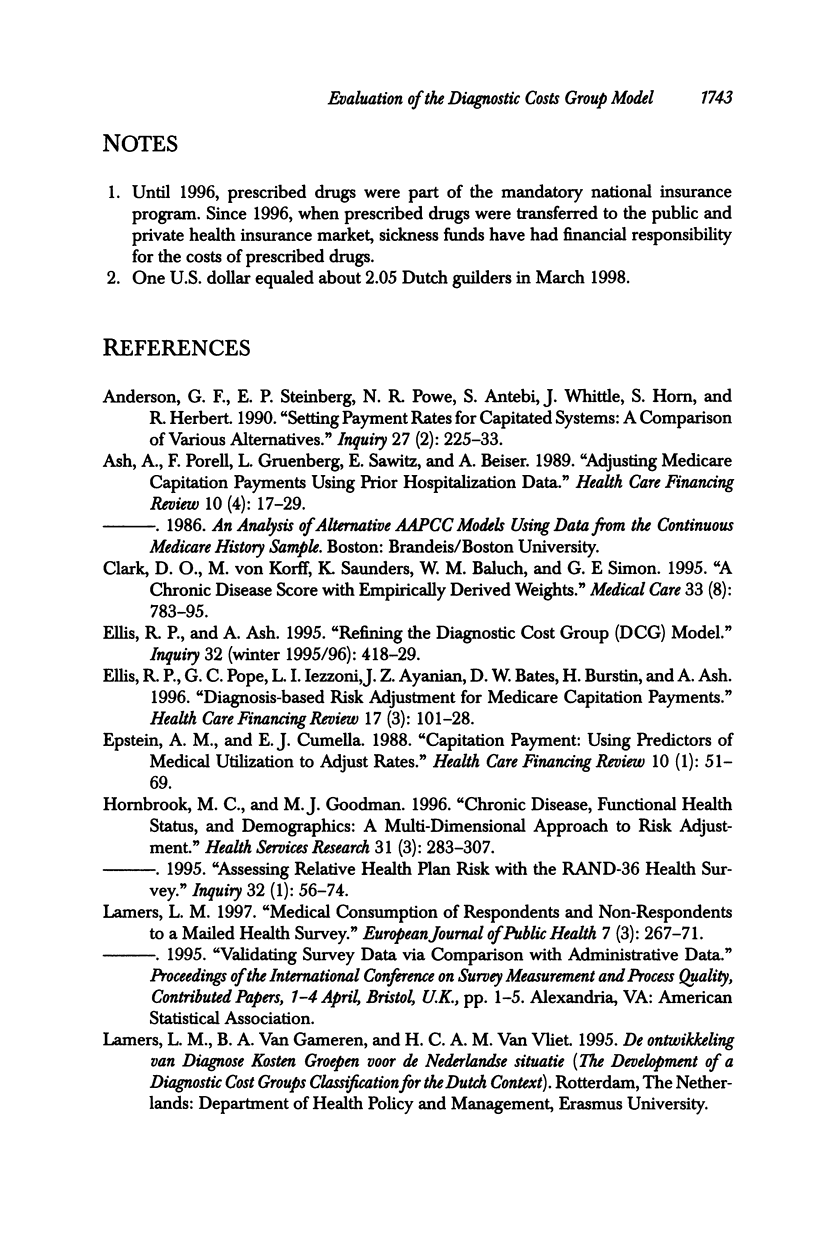
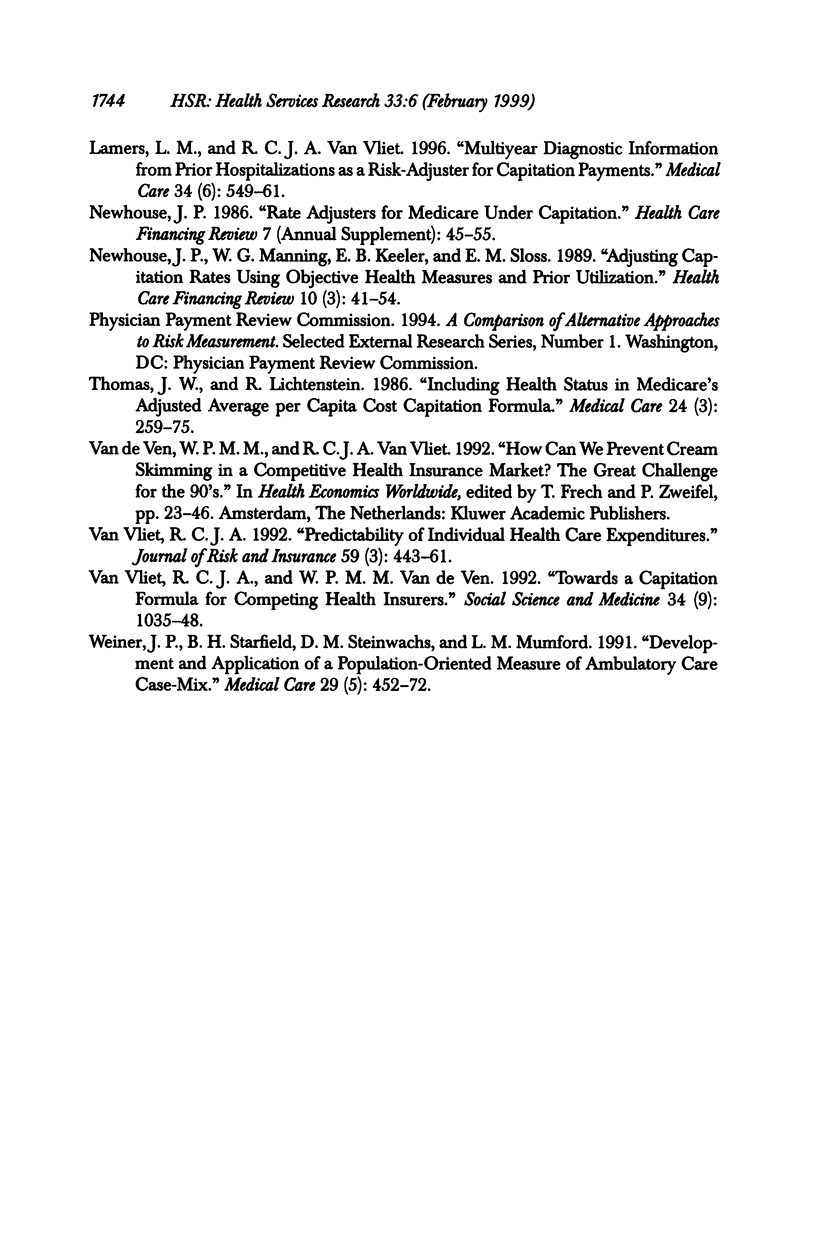
Selected References
These references are in PubMed. This may not be the complete list of references from this article.
- Anderson G. F., Steinberg E. P., Powe N. R., Antebi S., Whittle J., Horn S., Herbert R. Setting payment rates for capitated systems: a comparison of various alternatives. Inquiry. 1990 Fall;27(3):225–233. [PubMed] [Google Scholar]
- Ash A., Porell F., Gruenberg L., Sawitz E., Beiser A. Adjusting Medicare capitation payments using prior hospitalization data. Health Care Financ Rev. 1989 Summer;10(4):17–29. [PMC free article] [PubMed] [Google Scholar]
- Clark D. O., Von Korff M., Saunders K., Baluch W. M., Simon G. E. A chronic disease score with empirically derived weights. Med Care. 1995 Aug;33(8):783–795. doi: 10.1097/00005650-199508000-00004. [DOI] [PubMed] [Google Scholar]
- Ellis R. P., Ash A. Refinements to the Diagnostic Cost Group (DCG) model. Inquiry. 1995;32(4):418–429. [PubMed] [Google Scholar]
- Ellis R. P., Pope G. C., Iezzoni L., Ayanian J. Z., Bates D. W., Burstin H., Ash A. S. Diagnosis-based risk adjustment for Medicare capitation payments. Health Care Financ Rev. 1996 Spring;17(3):101–128. [PMC free article] [PubMed] [Google Scholar]
- Epstein A. M., Cumella E. J. Capitation payment: using predictors for medical utilization to adjust rates. Health Care Financ Rev. 1988 Fall;10(1):51–69. [PMC free article] [PubMed] [Google Scholar]
- Hornbrook M. C., Goodman M. J. Chronic disease, functional health status, and demographics: a multi-dimensional approach to risk adjustment. Health Serv Res. 1996 Aug;31(3):283–307. [PMC free article] [PubMed] [Google Scholar]
- Lamers L. M., van Vliet R. C. Multiyear diagnostic information from prior hospitalization as a risk-adjuster for capitation payments. Med Care. 1996 Jun;34(6):549–561. doi: 10.1097/00005650-199606000-00005. [DOI] [PubMed] [Google Scholar]
- Newhouse J. P., Manning W. G., Keeler E. B., Sloss E. M. Adjusting capitation rates using objective health measures and prior utilization. Health Care Financ Rev. 1989 Spring;10(3):41–54. [PMC free article] [PubMed] [Google Scholar]
- Newhouse J. P. Rate adjusters for Medicare under capitation. Health Care Financ Rev. 1986;(Spec No):45–55. [PMC free article] [PubMed] [Google Scholar]
- Thomas J. W., Lichtenstein R. Including health status in Medicare's Adjusted Average Per Capita Cost capitation formula. Med Care. 1986 Mar;24(3):259–275. doi: 10.1097/00005650-198603000-00008. [DOI] [PubMed] [Google Scholar]
- Van de Ven W. P., Van Vliet R. C. How can we prevent cream skimming in a competitive health insurance market? The great challenge for the 90's. Dev Health Econ Public Policy. 1992;1:23–46. doi: 10.1007/978-94-011-2392-1_2. [DOI] [PubMed] [Google Scholar]
- Weiner J. P., Starfield B. H., Steinwachs D. M., Mumford L. M. Development and application of a population-oriented measure of ambulatory care case-mix. Med Care. 1991 May;29(5):452–472. doi: 10.1097/00005650-199105000-00006. [DOI] [PubMed] [Google Scholar]
- van Vliet R. C., van de Ven W. P. Towards a capitation formula for competing health insurers. An empirical analysis. Soc Sci Med. 1992 May;34(9):1035–1048. doi: 10.1016/0277-9536(92)90134-c. [DOI] [PubMed] [Google Scholar]