Abstract
We show how to generate case-based explanations for non-case-based learning methods such as artificial neural nets or decision trees. The method uses the trained model (e.g., the neural net or the decision tree) as a distance metric to determine which cases in the training set are most similar to the case that needs to be explained. This approach is well suited to medical domains, where it is important to understand predictions made by complex machine learning models, and where training and clinical practice makes users adept at case interpretation.
Full text
PDF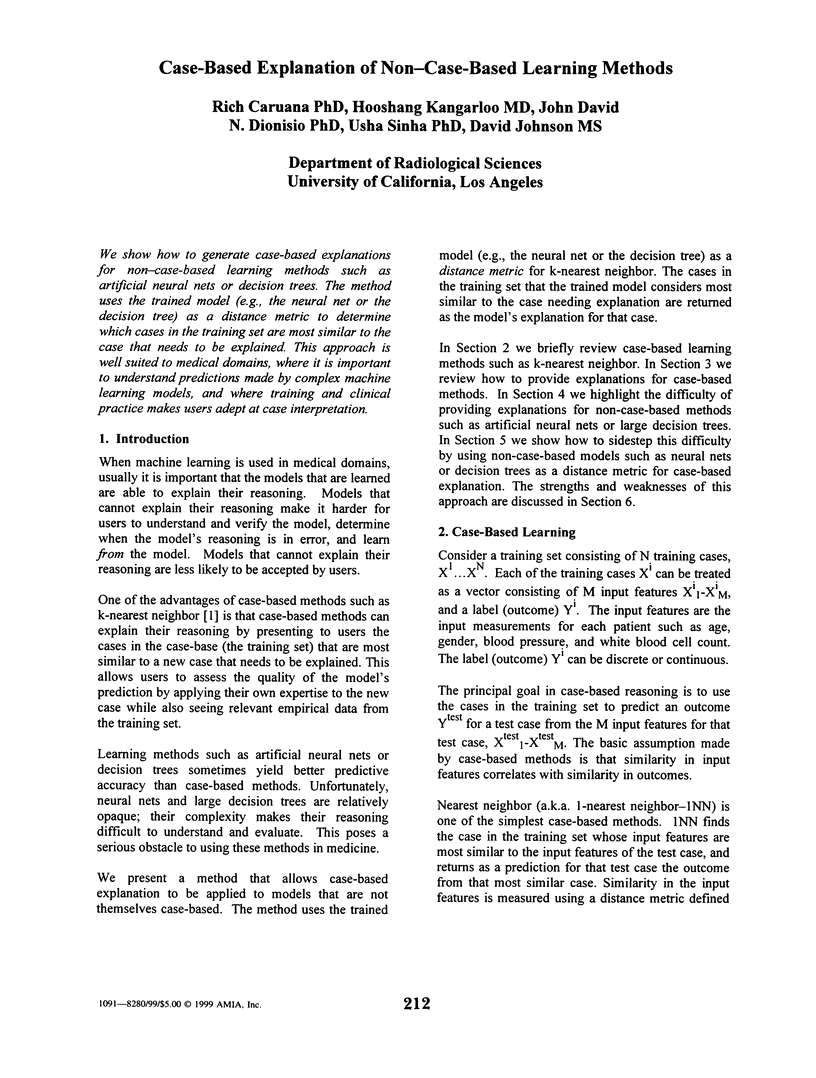
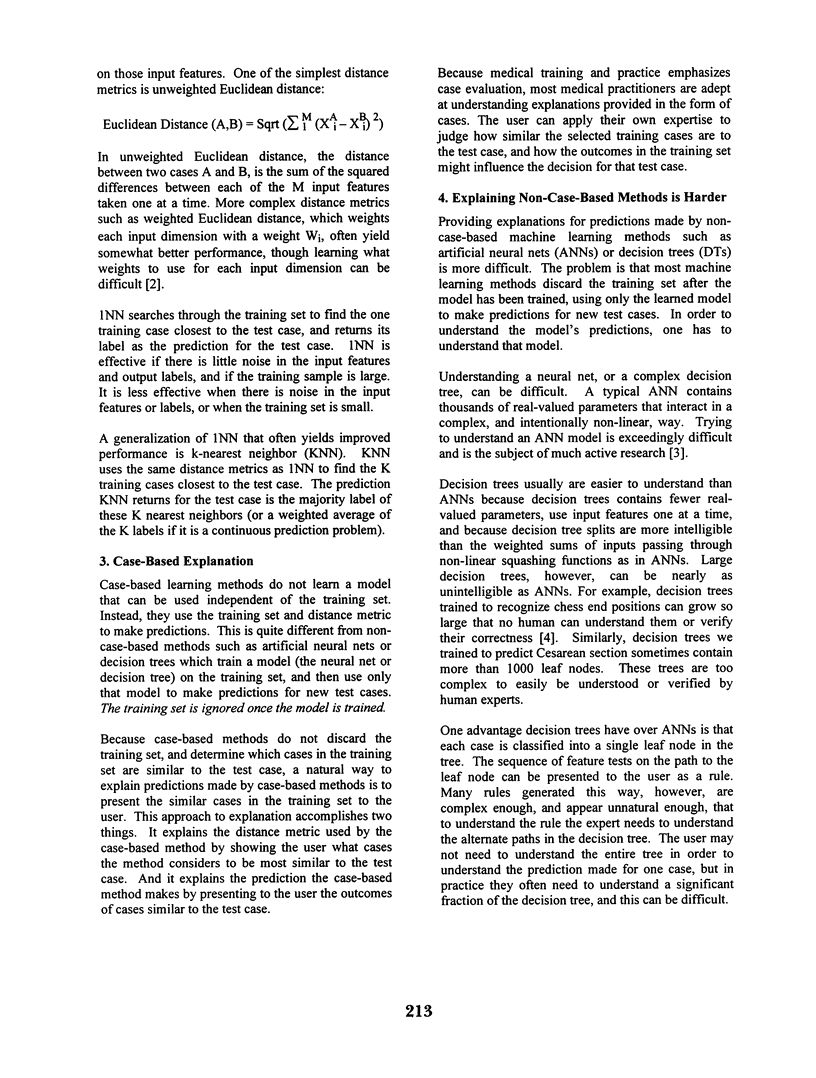
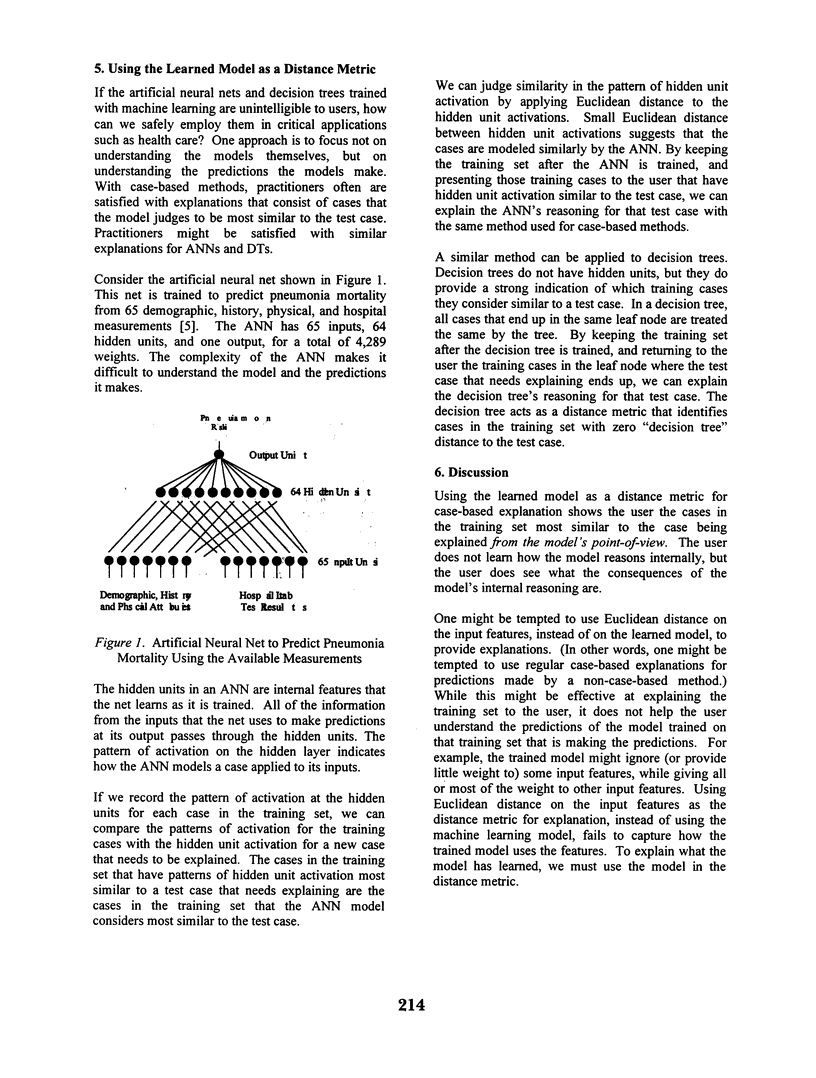
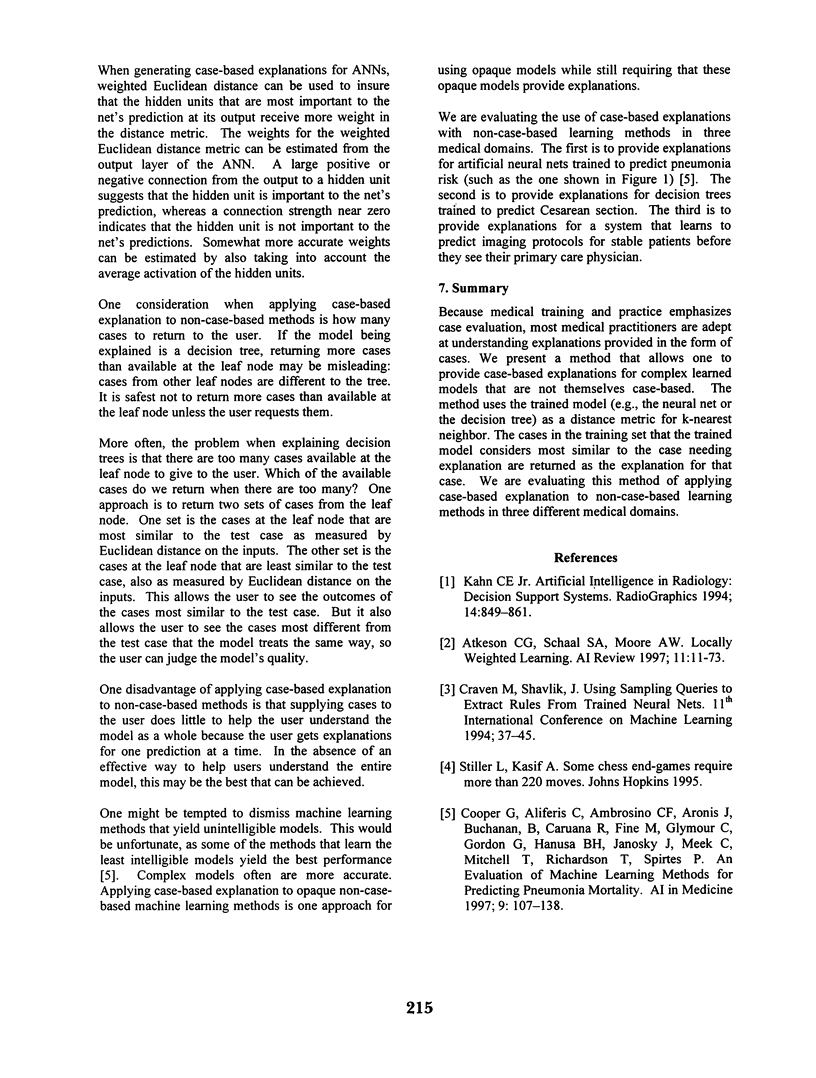
Selected References
These references are in PubMed. This may not be the complete list of references from this article.
- Cooper G. F., Aliferis C. F., Ambrosino R., Aronis J., Buchanan B. G., Caruana R., Fine M. J., Glymour C., Gordon G., Hanusa B. H. An evaluation of machine-learning methods for predicting pneumonia mortality. Artif Intell Med. 1997 Feb;9(2):107–138. doi: 10.1016/s0933-3657(96)00367-3. [DOI] [PubMed] [Google Scholar]
- Kahn C. E., Jr Artificial intelligence in radiology: decision support systems. Radiographics. 1994 Jul;14(4):849–861. doi: 10.1148/radiographics.14.4.7938772. [DOI] [PubMed] [Google Scholar]