Abstract
Accurately risk-stratifying patients is a key component of health care outcomes assessment. And, many health care organizations increasingly are relying upon automated means for assistance in making patient risk-stratification decisions. Unfortunately, the process of outcome model development, as it is currently practiced, is both time consuming and difficult. We investigated the relative abilities of three modeling techniques (logistic regression, artificial neural network (ANN), and Bayesian) to rapidly develop models for risk-stratifying patients. Our results demonstrated that all three modeling techniques perform equally well in certain situations. However, the Bayesian model with conditional independence had the best overall performance. Unfortunately, none of the models were able to achieve the degree of accuracy which would be required in a medical setting.
Full text
PDF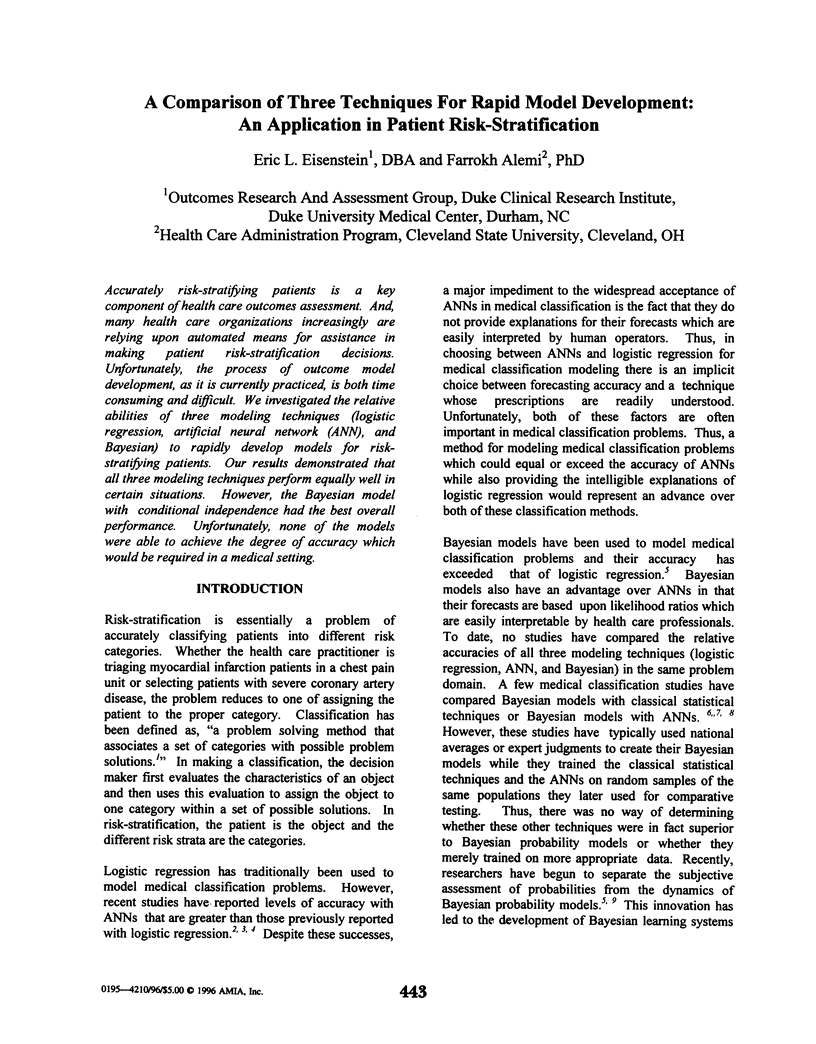
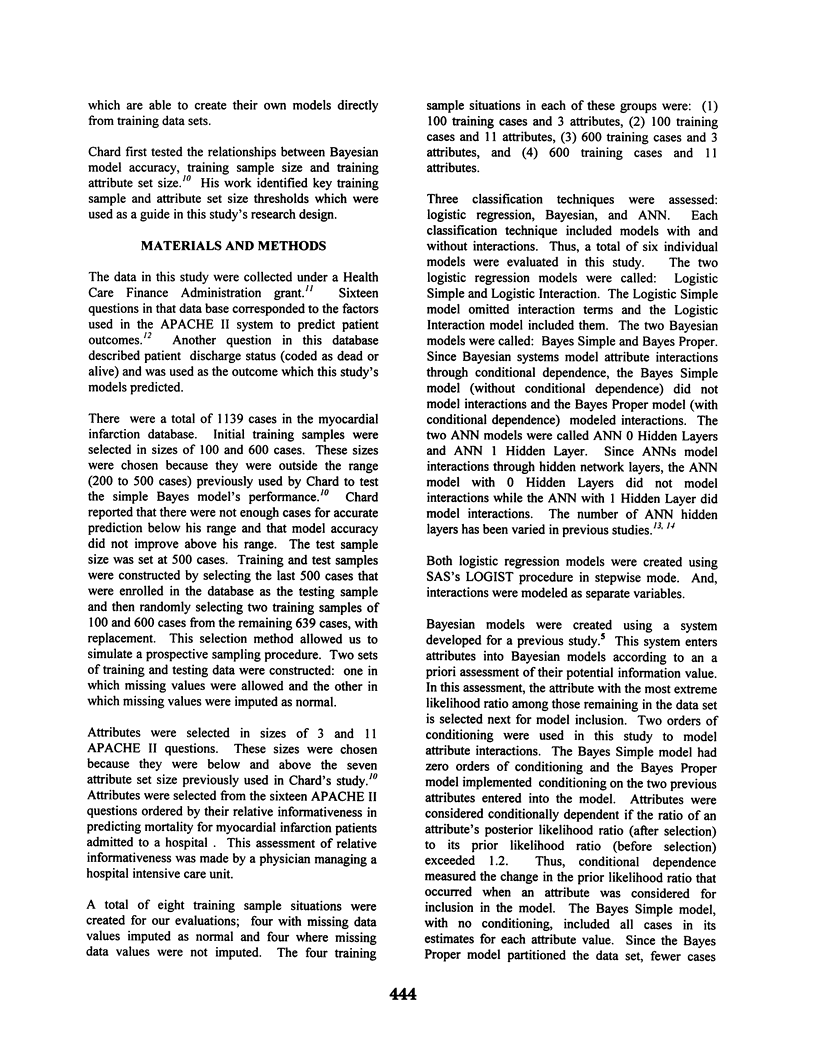
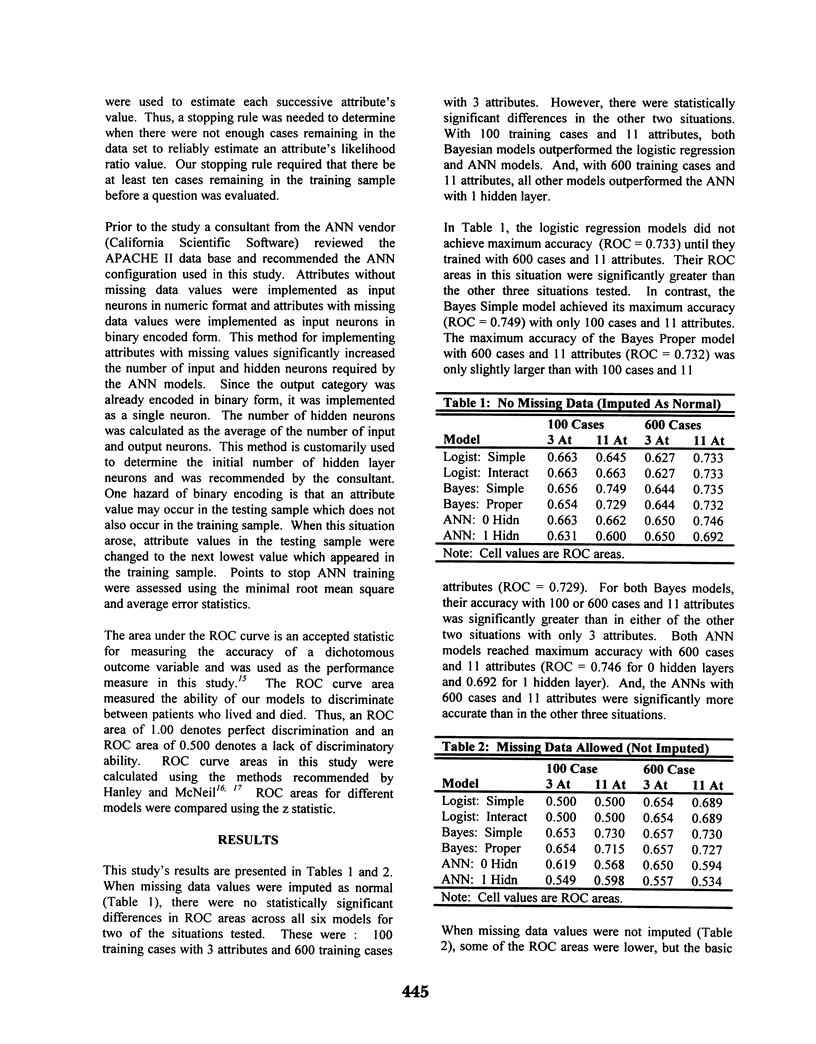
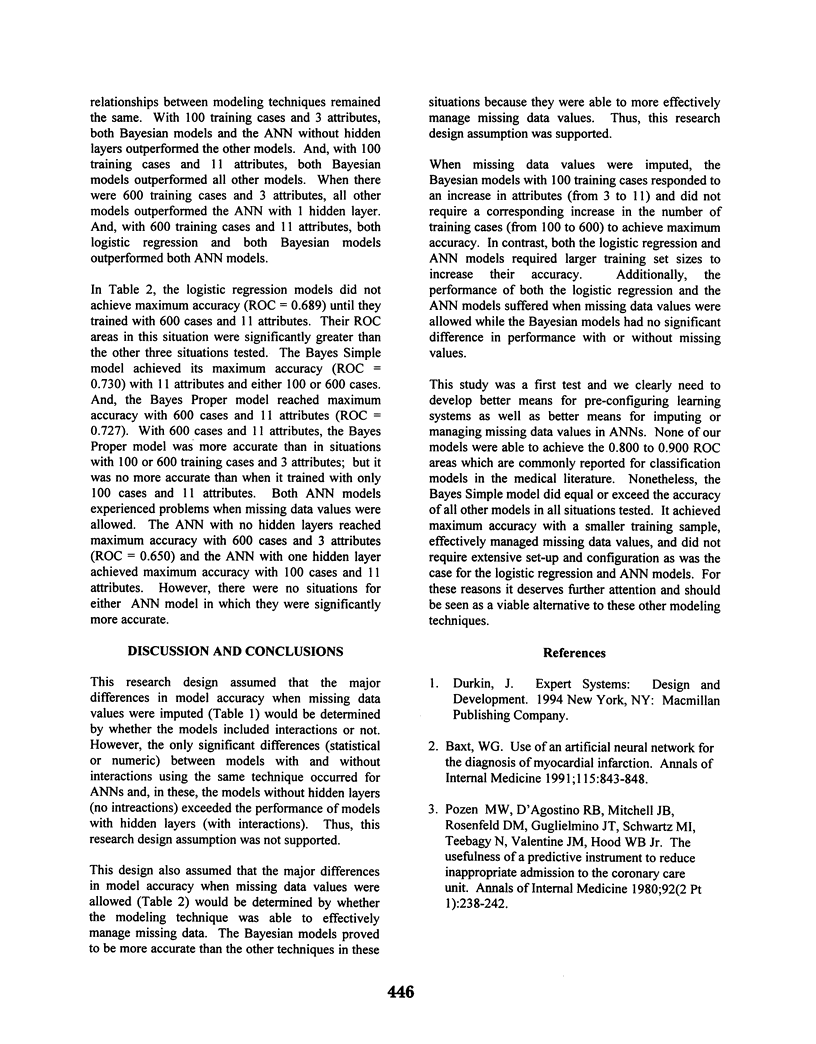
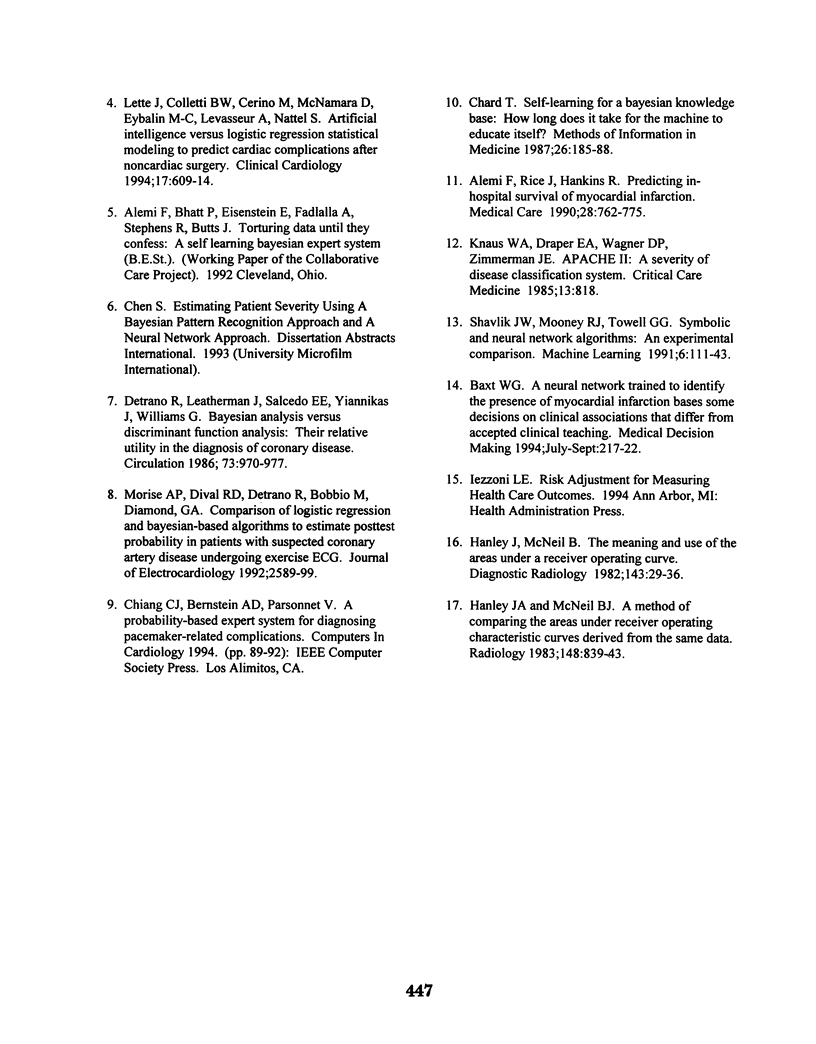
Selected References
These references are in PubMed. This may not be the complete list of references from this article.
- Alemi F., Rice J., Hankins R. Predicting in-hospital survival of myocardial infarction. A comparative study of various severity measures. Med Care. 1990 Sep;28(9):762–775. doi: 10.1097/00005650-199009000-00006. [DOI] [PubMed] [Google Scholar]
- Baxt W. G. A neural network trained to identify the presence of myocardial infarction bases some decisions on clinical associations that differ from accepted clinical teaching. Med Decis Making. 1994 Jul-Sep;14(3):217–222. doi: 10.1177/0272989X9401400303. [DOI] [PubMed] [Google Scholar]
- Chard T. Self-learning for a Bayesian knowledge base: how long does it take for the machine to educate itself? Methods Inf Med. 1987 Oct;26(4):185–188. [PubMed] [Google Scholar]
- Detrano R., Leatherman J., Salcedo E. E., Yiannikas J., Williams G. Bayesian analysis versus discriminant function analysis: their relative utility in the diagnosis of coronary disease. Circulation. 1986 May;73(5):970–977. doi: 10.1161/01.cir.73.5.970. [DOI] [PubMed] [Google Scholar]
- Hanley J. A., McNeil B. J. The meaning and use of the area under a receiver operating characteristic (ROC) curve. Radiology. 1982 Apr;143(1):29–36. doi: 10.1148/radiology.143.1.7063747. [DOI] [PubMed] [Google Scholar]
- Knaus W. A., Draper E. A., Wagner D. P., Zimmerman J. E. APACHE II: a severity of disease classification system. Crit Care Med. 1985 Oct;13(10):818–829. [PubMed] [Google Scholar]
- Lette J., Colletti B. W., Cerino M., McNamara D., Eybalin M. C., Levasseur A., Nattel S. Artificial intelligence versus logistic regression statistical modelling to predict cardiac complications after noncardiac surgery. Clin Cardiol. 1994 Nov;17(11):609–614. doi: 10.1002/clc.4960171109. [DOI] [PubMed] [Google Scholar]
- Pozen M. W., D'Agostino R. B., Mitchell J. B., Rosenfeld D. M., Guglielmino J. T., Schwartz M. L., Teebagy N., Valentine J. M., Hood W. B., Jr The usefulness of a predictive instrument to reduce inappropriate admissions to the coronary care unit. Ann Intern Med. 1980 Feb;92(2 Pt 1):238–242. doi: 10.7326/0003-4819-92-2-238. [DOI] [PubMed] [Google Scholar]