Abstract
We are concerned with the rating of new documents that appear in a large database (MEDLINE) and are candidates for inclusion in a small specialty database (REBASE). The requirement is to rank the new documents as nearly in order of decreasing potential to be added to the smaller database as possible, so as to improve the coverage of the smaller database without increasing the effort of those who manage this specialty database. To perform this ranking task we have considered several machine learning approaches based on the naï ve Bayesian algorithm. We find that adaptive boosting outperforms naï ve Bayes, but that a new form of boosting which we term staged Bayesian retrieval outperforms adaptive boosting. Staged Bayesian retrieval involves two stages of Bayesian retrieval and we further find that if the second stage is replaced by a support vector machine we again obtain a significant improvement over the strictly Bayesian approach.
Full text
PDF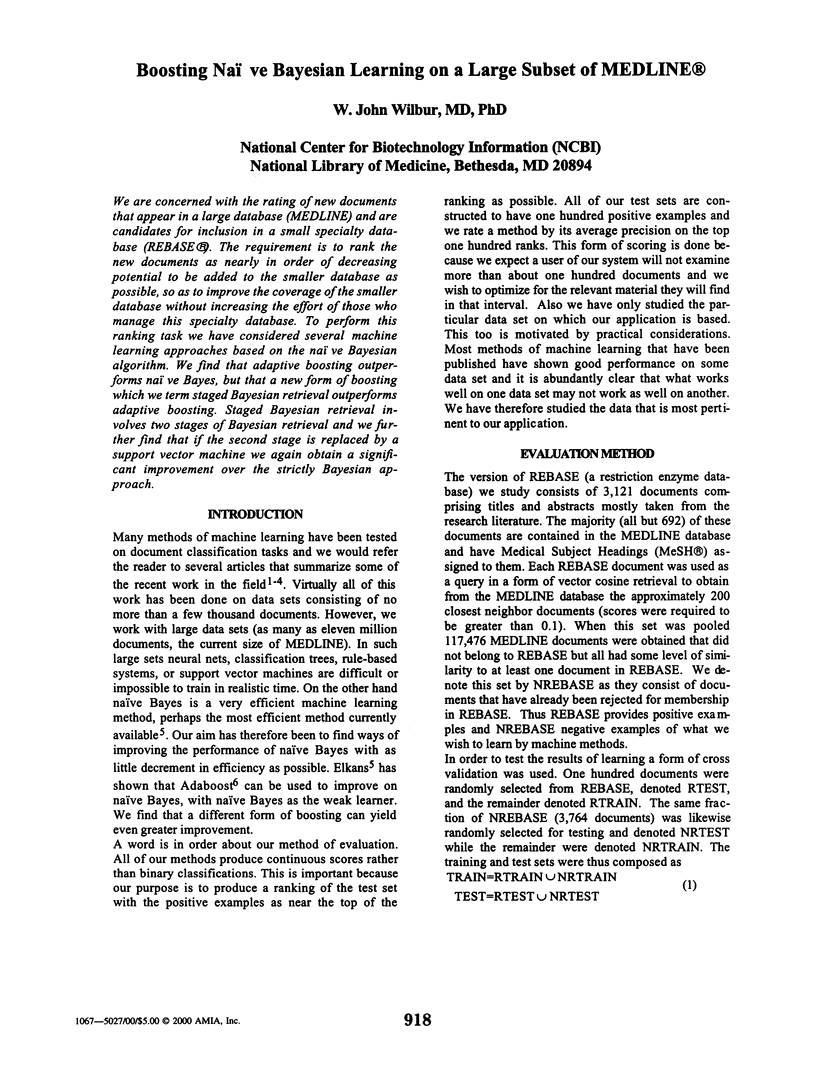
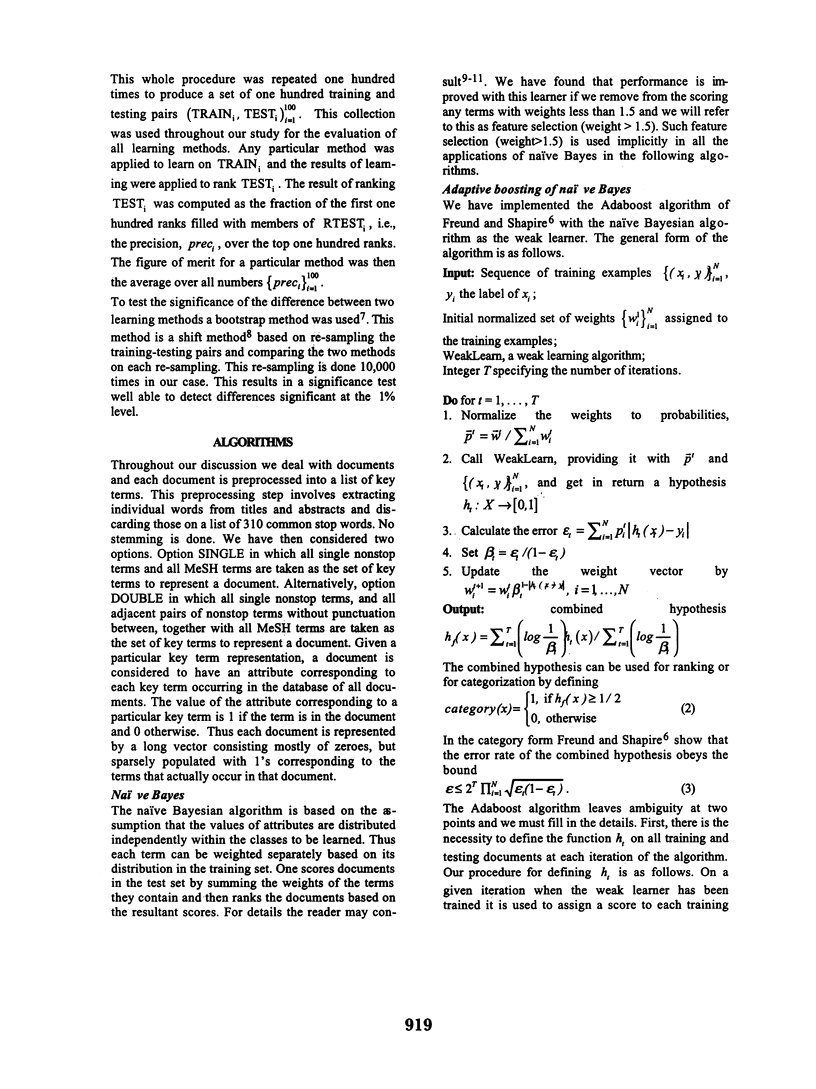
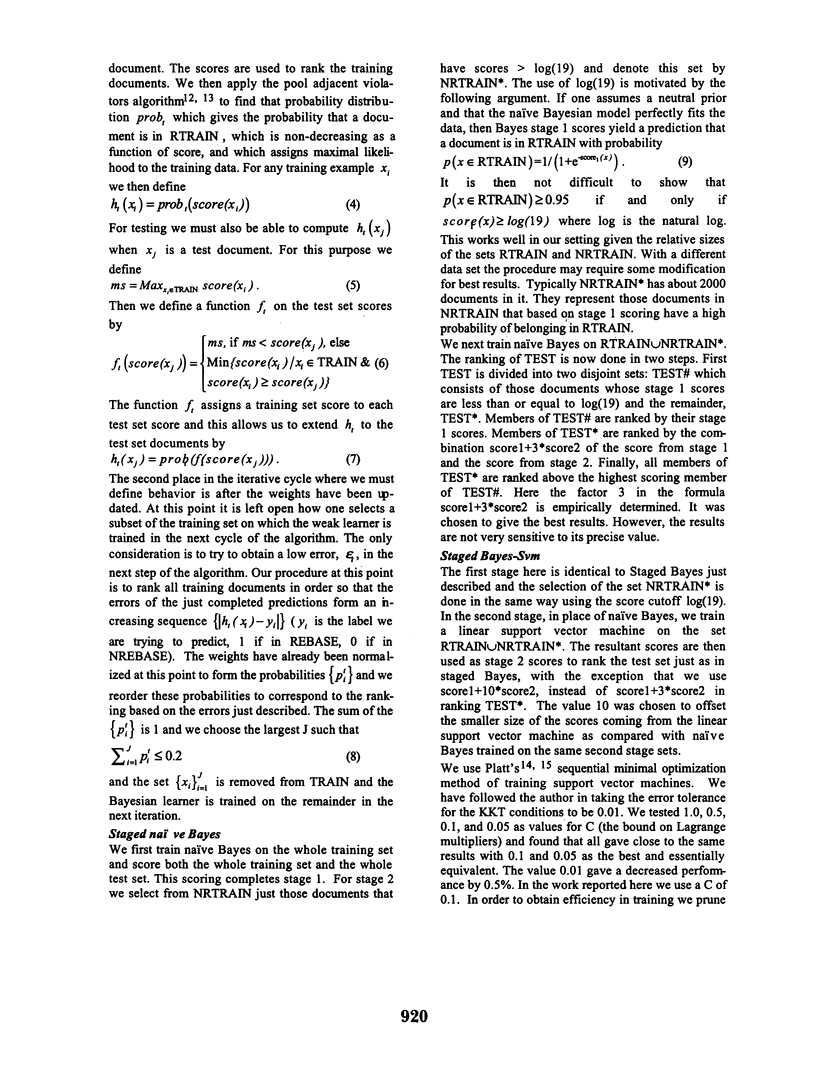
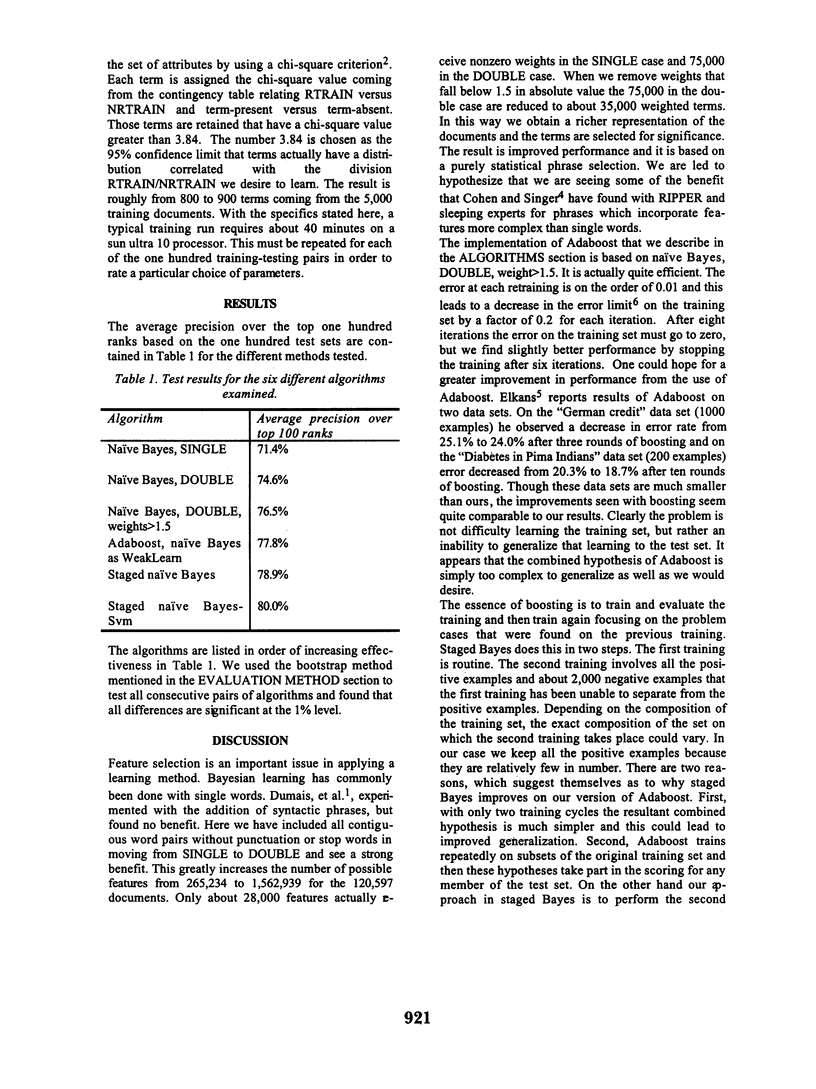
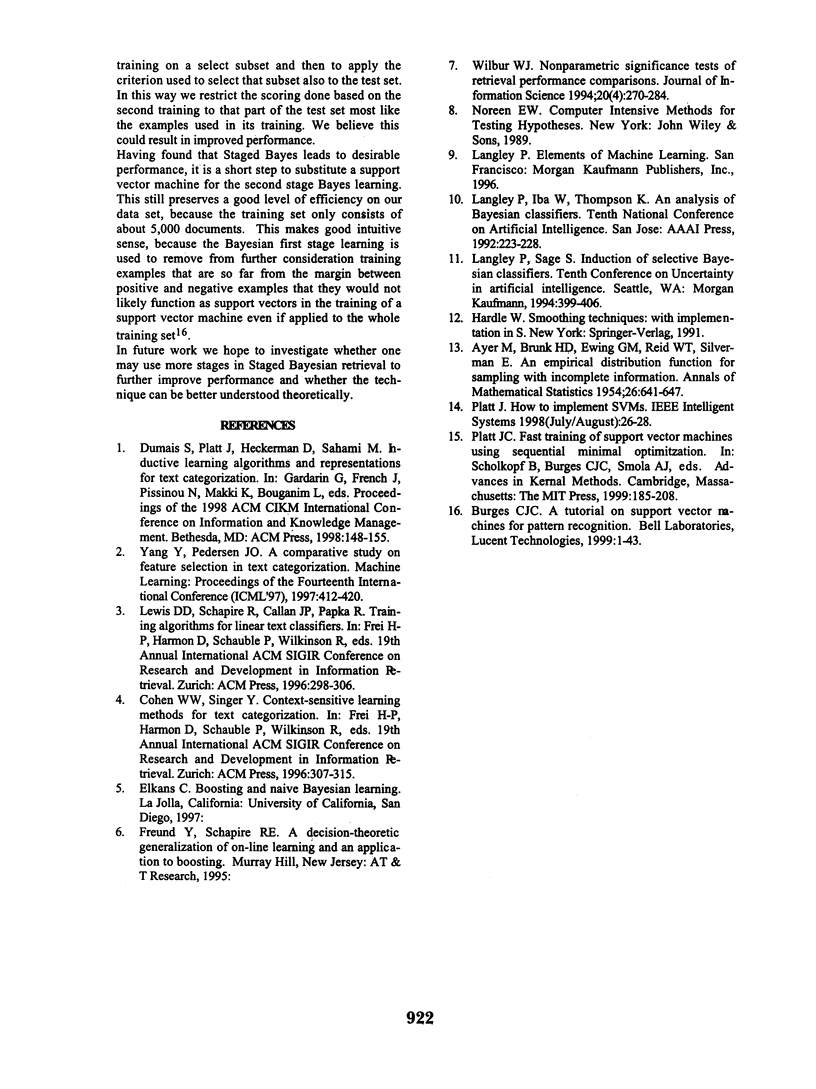