Abstract
Abdominal magnetic resonance imaging (MRI) plays an important role in the evaluation of liver abnormalities. The interpretation of MR images requires expert training in a rapidly changing field. DAFODILL (Decision Aid for Diagnosing Liver Lesions) is a decision-support tool designed to aid radiologists in the diagnosis of hepatic lesions seen on MRI. DAFODILL uses a knowledge base of MRI findings and a belief-network inference engine to generate probabilistic differential diagnoses of the most commonly encountered hepatic lesions. DAFODILL performs limited image processing to identify clinically relevant features, which are presented to the user for confirmation before they are used by the network. Preliminary evaluation of an initial version of the system suggests that DAFODILL may be a useful tool for radiology residents and nonexpert radiologists in interpreting MR images of the liver.
Full text
PDF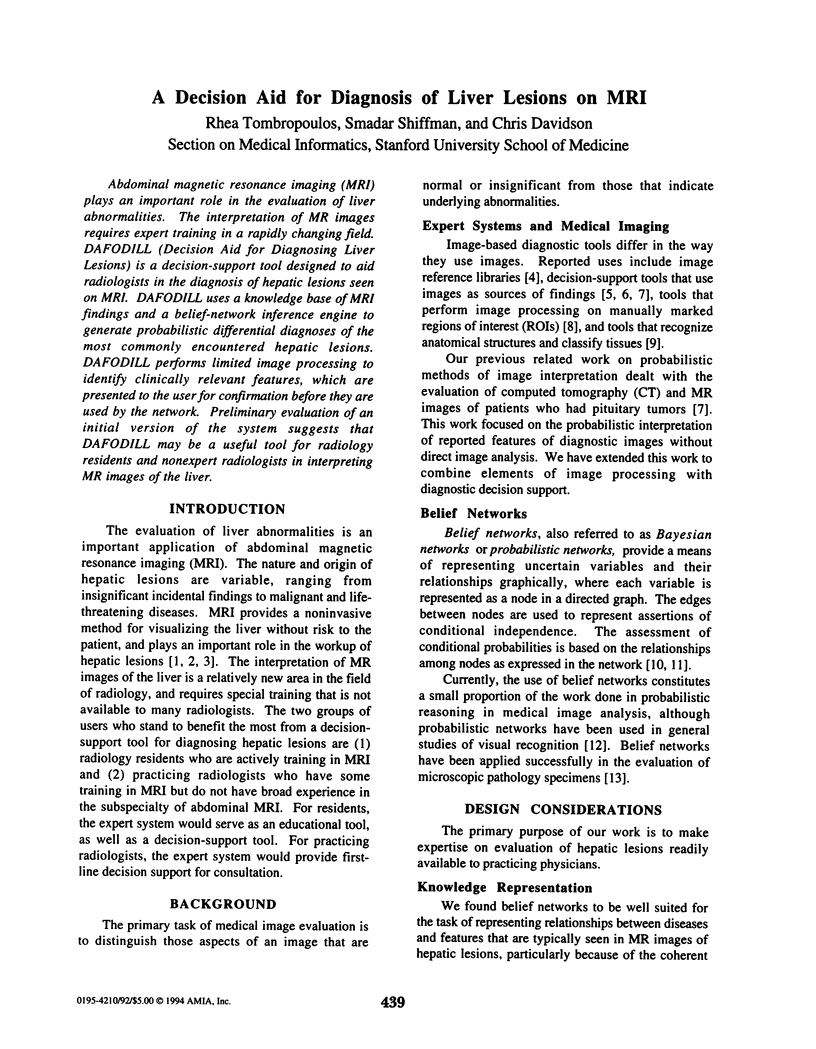
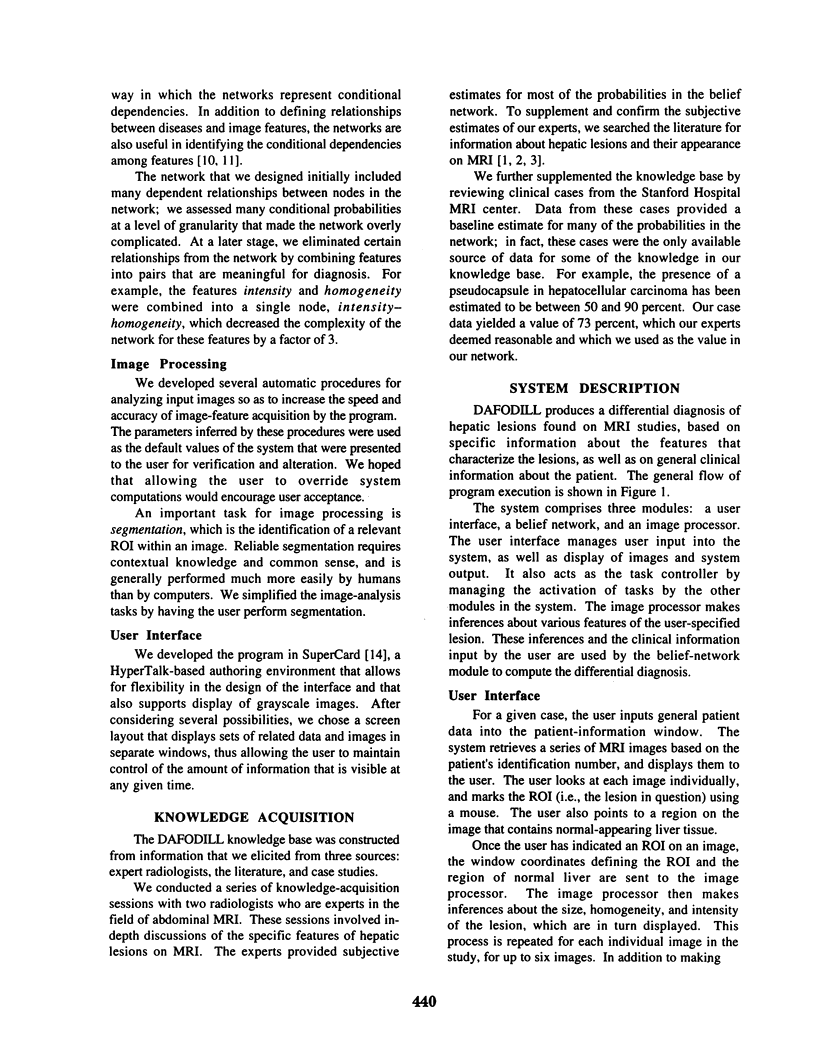
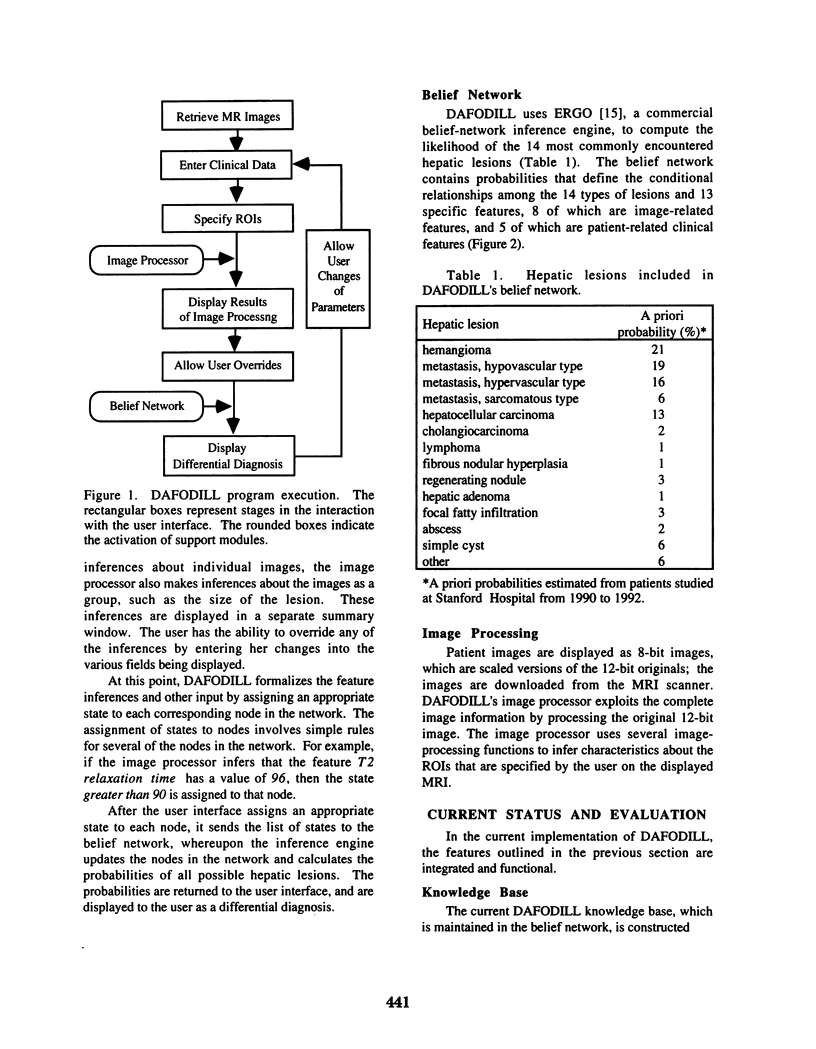
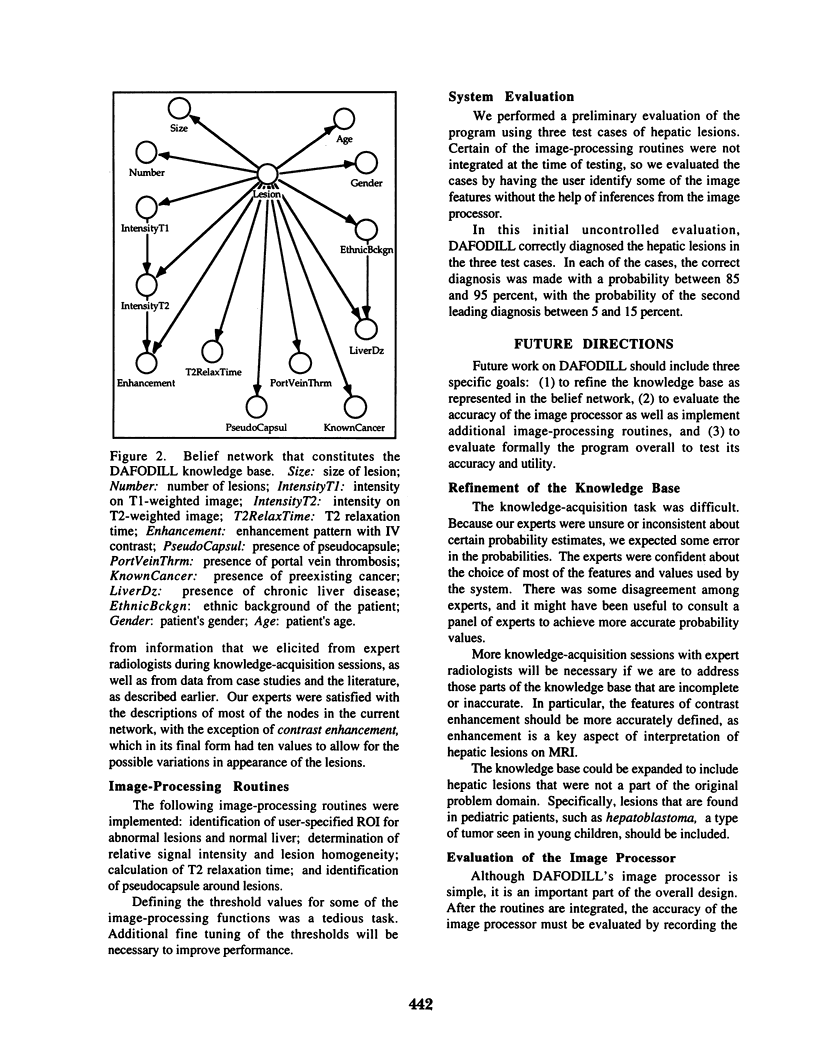
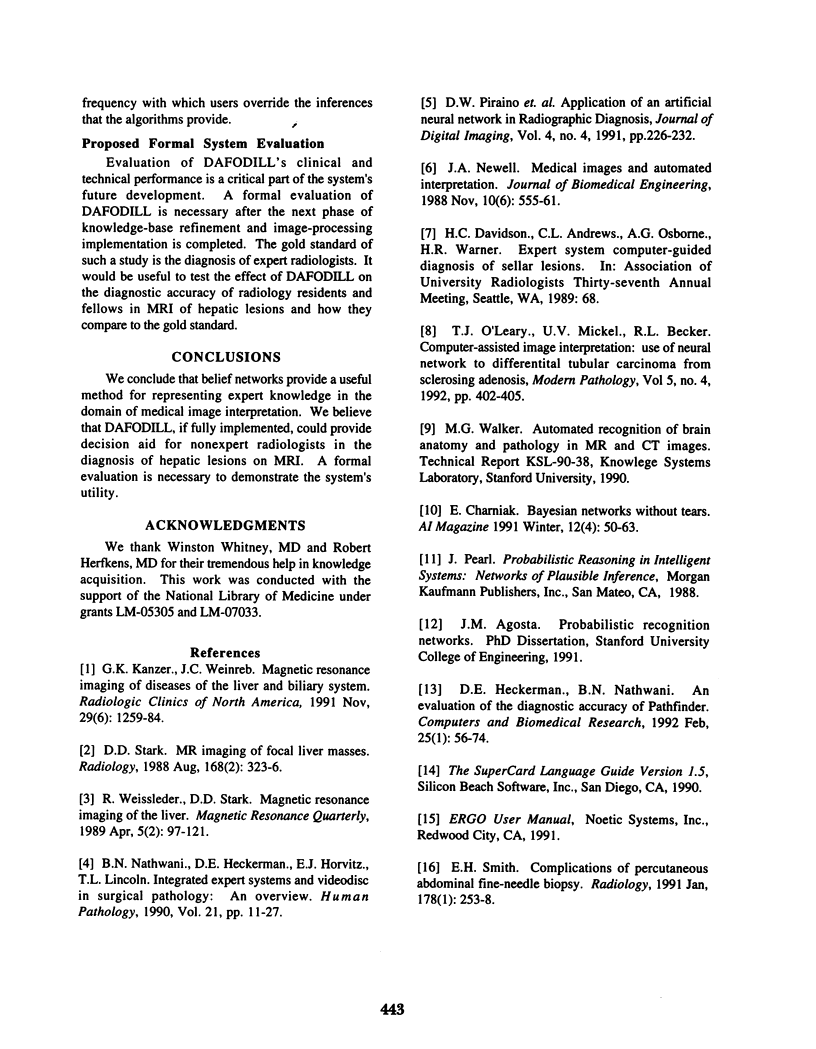
Selected References
These references are in PubMed. This may not be the complete list of references from this article.
- Heckerman D. E., Nathwani B. N. An evaluation of the diagnostic accuracy of Pathfinder. Comput Biomed Res. 1992 Feb;25(1):56–74. doi: 10.1016/0010-4809(92)90035-9. [DOI] [PubMed] [Google Scholar]
- Kanzer G. K., Weinreb J. C. Magnetic resonance imaging of diseases of the liver and biliary system. Radiol Clin North Am. 1991 Nov;29(6):1259–1284. [PubMed] [Google Scholar]
- Nathwani B. N., Heckerman D. E., Horvitz E. J., Lincoln T. L. Integrated expert systems and videodisc in surgical pathology: an overview. Hum Pathol. 1990 Jan;21(1):11–27. doi: 10.1016/0046-8177(90)90071-c. [DOI] [PubMed] [Google Scholar]
- Newell J. A. Medical images and automated interpretation. J Biomed Eng. 1988 Nov;10(6):555–561. doi: 10.1016/0141-5425(88)90115-x. [DOI] [PubMed] [Google Scholar]
- O'Leary T. J., Mikel U. V., Becker R. L. Computer-assisted image interpretation: use of a neural network to differentiate tubular carcinoma from sclerosing adenosis. Mod Pathol. 1992 Jul;5(4):402–405. [PubMed] [Google Scholar]
- Piraino D. W., Amartur S. C., Richmond B. J., Schils J. P., Thome J. M., Belhobek G. H., Schlucter M. D. Application of an artificial neural network in radiographic diagnosis. J Digit Imaging. 1991 Nov;4(4):226–232. doi: 10.1007/BF03173904. [DOI] [PubMed] [Google Scholar]
- Smith E. H. Complications of percutaneous abdominal fine-needle biopsy. Review. Radiology. 1991 Jan;178(1):253–258. doi: 10.1148/radiology.178.1.1984314. [DOI] [PubMed] [Google Scholar]
- Stark D. D. MR imaging of focal liver masses. Radiology. 1988 Aug;168(2):323–326. doi: 10.1148/radiology.168.2.3293102. [DOI] [PubMed] [Google Scholar]
- Weissleder R., Stark D. D. Magnetic resonance imaging of the liver. Magn Reson Q. 1989 Apr;5(2):97–121. [PubMed] [Google Scholar]