Abstract
An analog model neural network that can solve a general problem of recognizing patterns in a time-dependent signal is presented. The networks use a patterned set of delays to collectively focus stimulus sequence information to a neural state at a future time. The computational capabilities of the circuit are demonstrated on tasks somewhat similar to those necessary for the recognition of words in a continuous stream of speech. The network architecture can be understood from consideration of an energy function that is being minimized as the circuit computes. Neurobiological mechanisms are known for the generation of appropriate delays.
Full text
PDF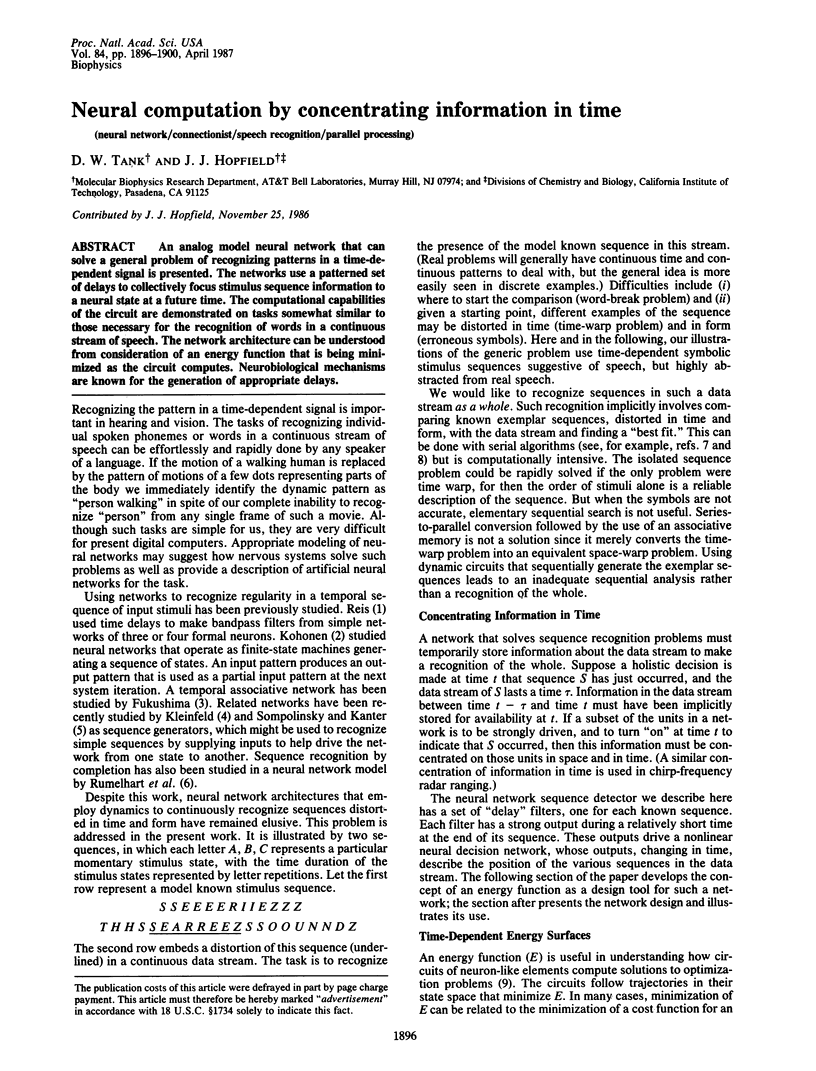
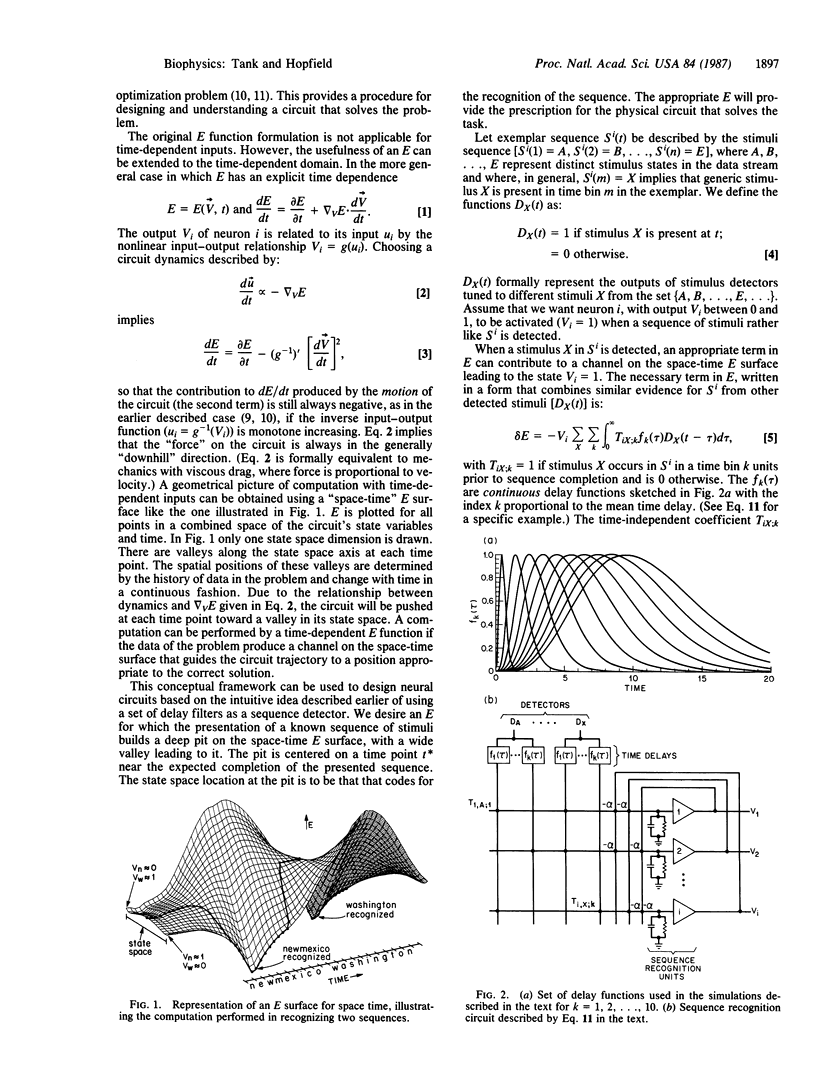
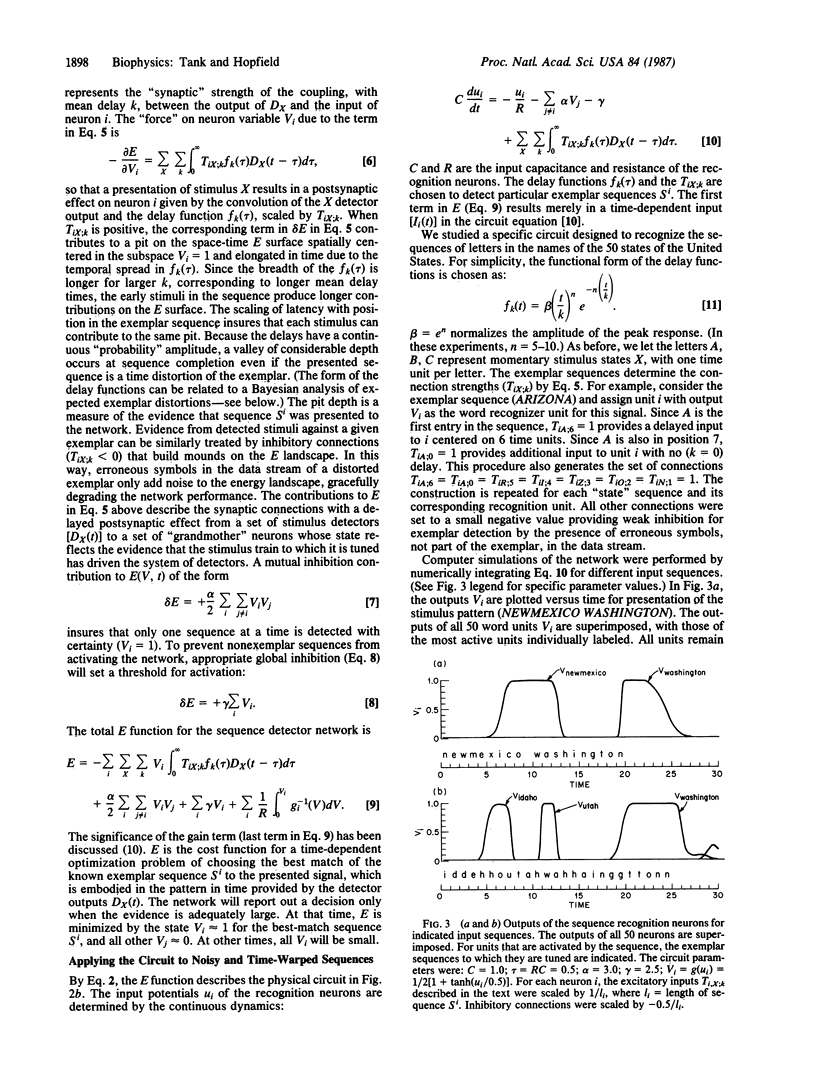
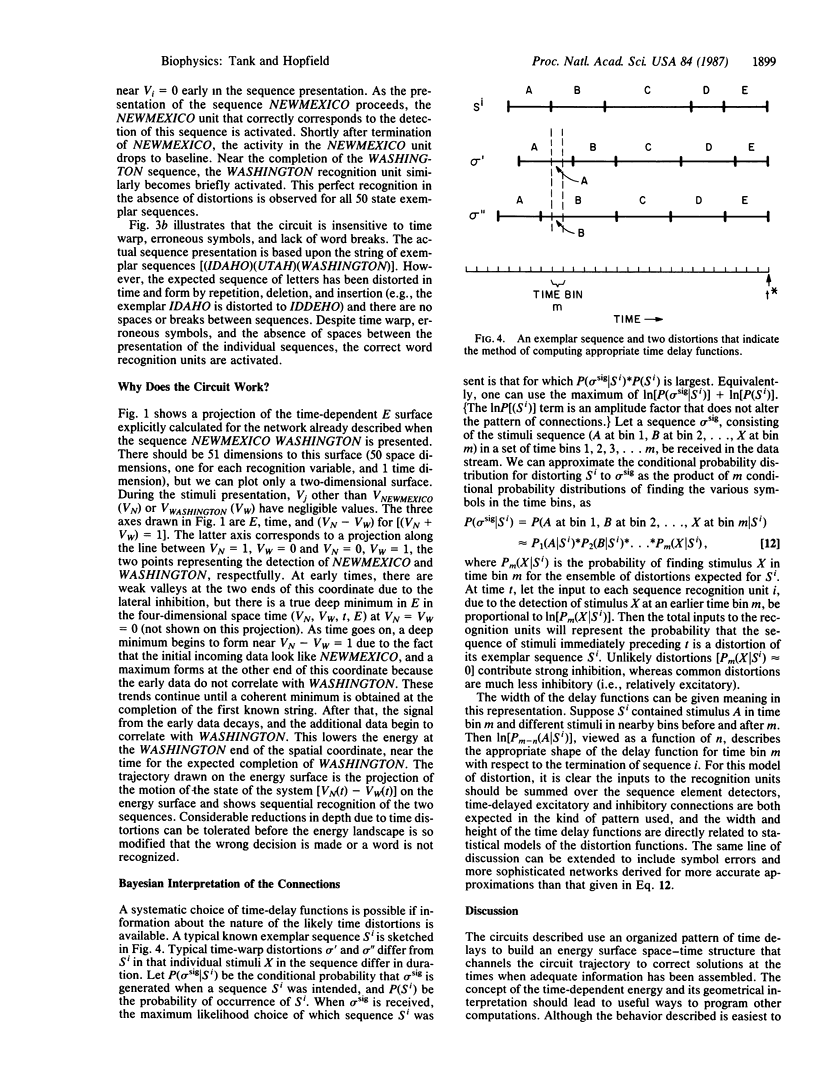
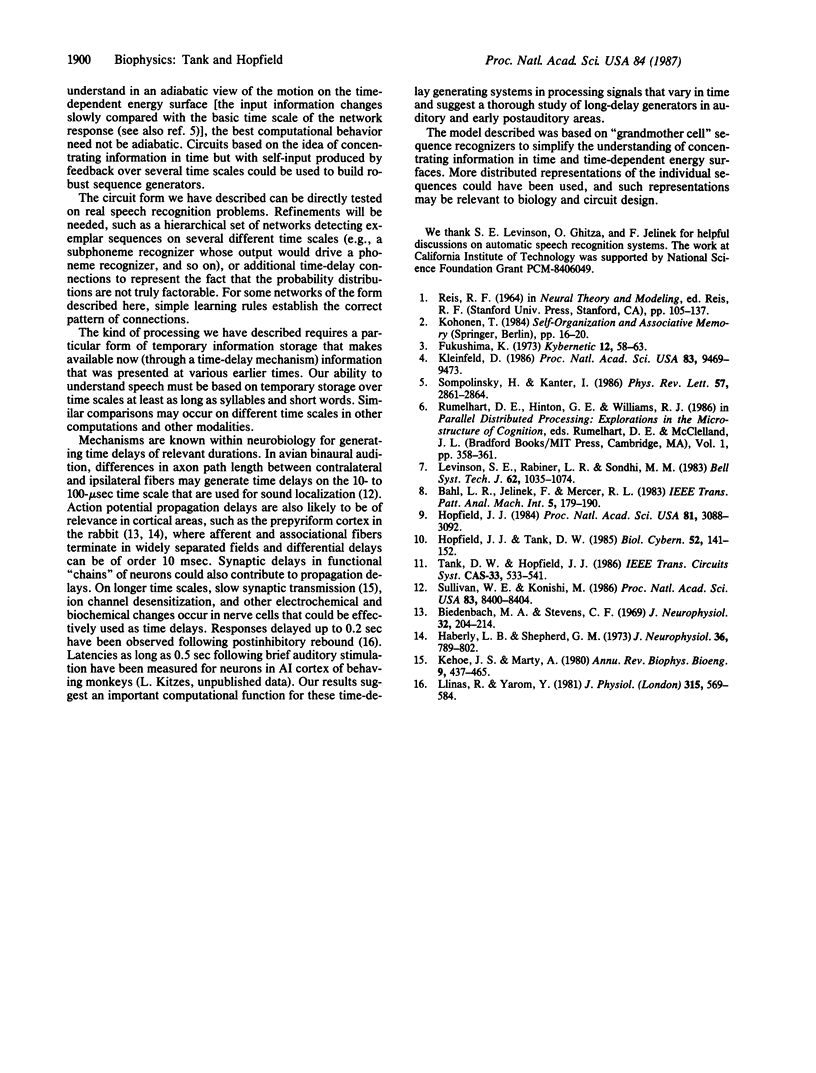
Selected References
These references are in PubMed. This may not be the complete list of references from this article.
- Biedenbach M. A., Stevens C. F. Synaptic organization of cat olfactory cortex as revealed by intracellular recording. J Neurophysiol. 1969 Mar;32(2):204–214. doi: 10.1152/jn.1969.32.2.204. [DOI] [PubMed] [Google Scholar]
- Fukushima K. A model of associative memory in the brain. Kybernetik. 1973 Feb;12(2):58–63. doi: 10.1007/BF00272461. [DOI] [PubMed] [Google Scholar]
- Haberly L. B., Shepherd G. M. Current-density analysis of summed evoked potentials in opossum prepyriform cortex. J Neurophysiol. 1973 Jul;36(4):789–802. doi: 10.1152/jn.1973.36.4.789. [DOI] [PubMed] [Google Scholar]
- Hopfield J. J. Neurons with graded response have collective computational properties like those of two-state neurons. Proc Natl Acad Sci U S A. 1984 May;81(10):3088–3092. doi: 10.1073/pnas.81.10.3088. [DOI] [PMC free article] [PubMed] [Google Scholar]
- Hopfield J. J., Tank D. W. "Neural" computation of decisions in optimization problems. Biol Cybern. 1985;52(3):141–152. doi: 10.1007/BF00339943. [DOI] [PubMed] [Google Scholar]
- Kehoe J. S., Marty A. Certain slow synaptic responses: their properties and possible underlying mechanisms. Annu Rev Biophys Bioeng. 1980;9:437–465. doi: 10.1146/annurev.bb.09.060180.002253. [DOI] [PubMed] [Google Scholar]
- Kleinfeld D. Sequential state generation by model neural networks. Proc Natl Acad Sci U S A. 1986 Dec;83(24):9469–9473. doi: 10.1073/pnas.83.24.9469. [DOI] [PMC free article] [PubMed] [Google Scholar]
- Llinás R., Yarom Y. Properties and distribution of ionic conductances generating electroresponsiveness of mammalian inferior olivary neurones in vitro. J Physiol. 1981 Jun;315:569–584. doi: 10.1113/jphysiol.1981.sp013764. [DOI] [PMC free article] [PubMed] [Google Scholar]
- Sompolinsky H, Kanter I., I Temporal association in asymmetric neural networks. Phys Rev Lett. 1986 Dec 1;57(22):2861–2864. doi: 10.1103/PhysRevLett.57.2861. [DOI] [PubMed] [Google Scholar]
- Sullivan W. E., Konishi M. Neural map of interaural phase difference in the owl's brainstem. Proc Natl Acad Sci U S A. 1986 Nov;83(21):8400–8404. doi: 10.1073/pnas.83.21.8400. [DOI] [PMC free article] [PubMed] [Google Scholar]