Abstract
Methods for optimizing the prediction of Escherichia coli RNA polymerase promoter sequences by neural networks are presented. A neural network was trained on a set of 80 known promoter sequences combined with different numbers of random sequences. The conserved -10 region and -35 region of the promoter sequences and a combination of these regions were used in three independent training sets. The prediction accuracy of the resulting weight matrix was tested against a separate set of 30 known promoter sequences and 1500 random sequences. The effects of the network's topology, the extent of training, the number of random sequences in the training set and the effects of different data representations were examined and optimized. Accuracies of 100% on the promoter test set and 98.4% on the random test set were achieved with the optimal parameters.
Full text
PDF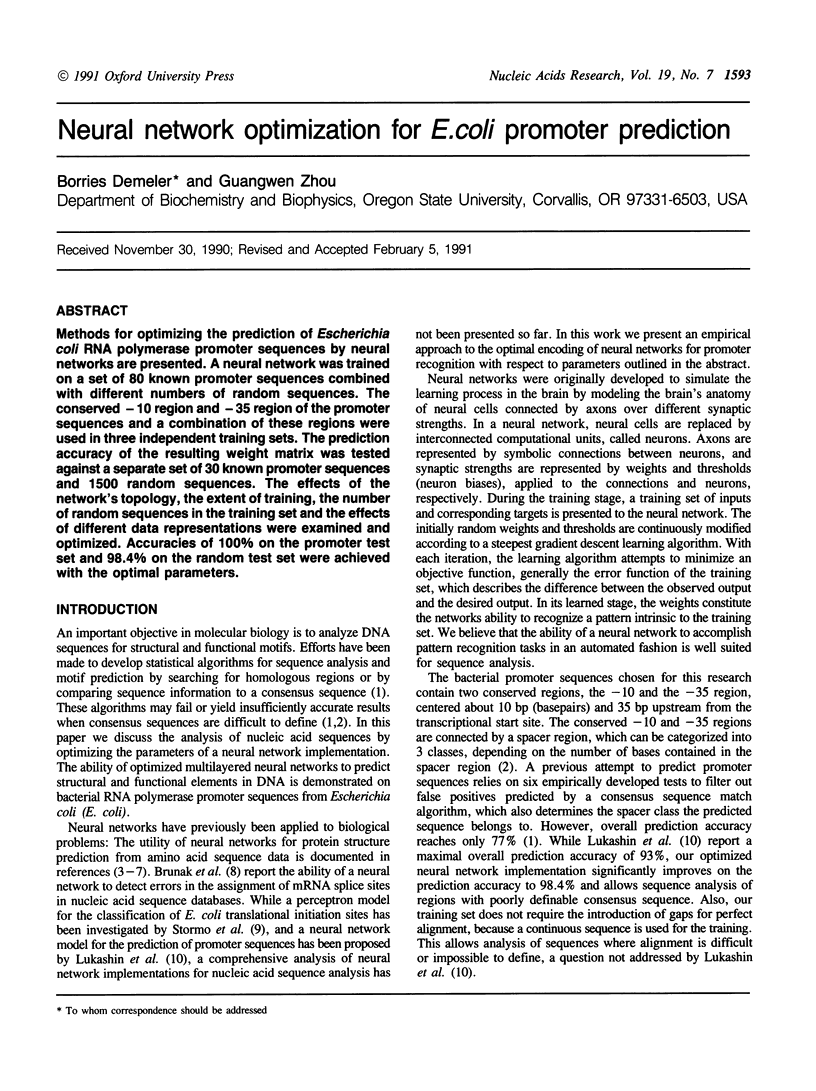
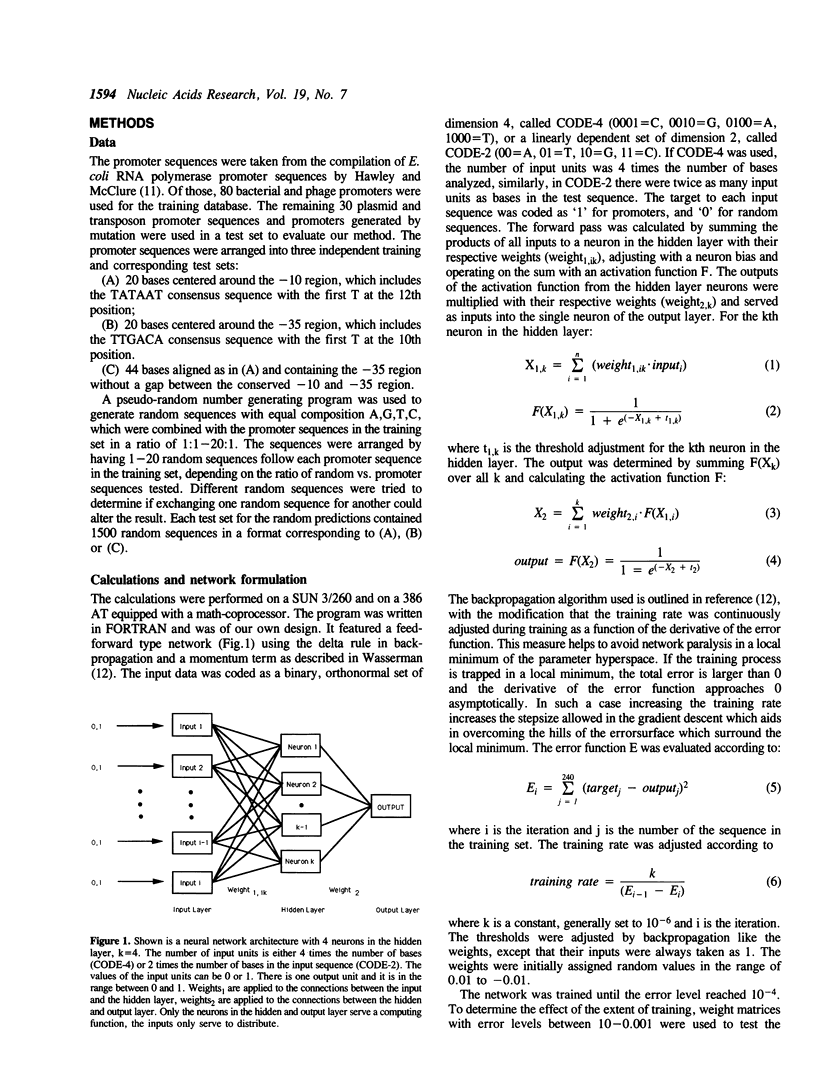
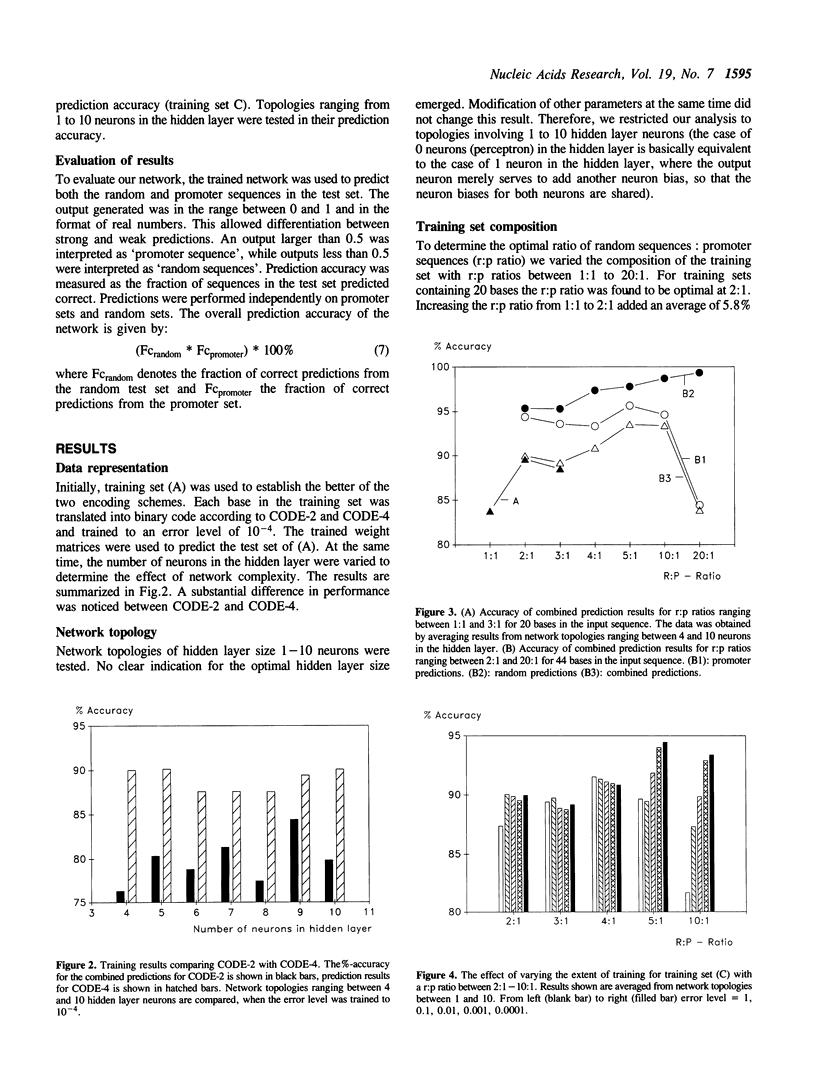
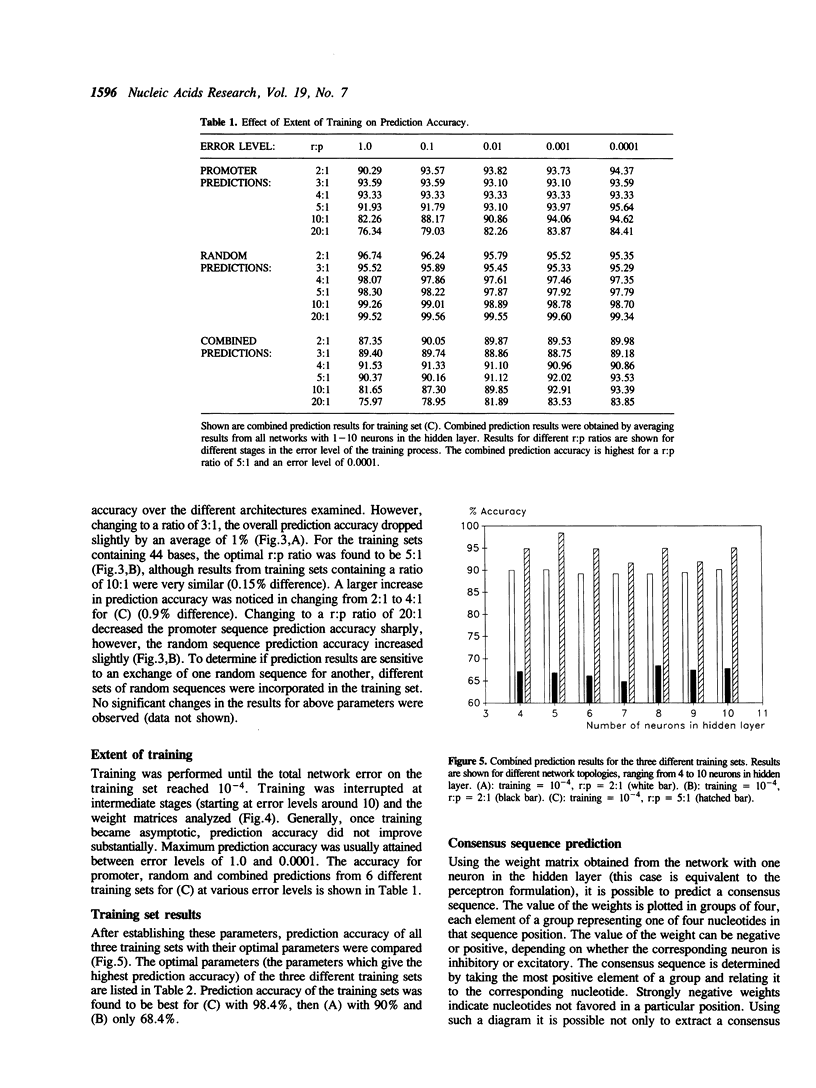
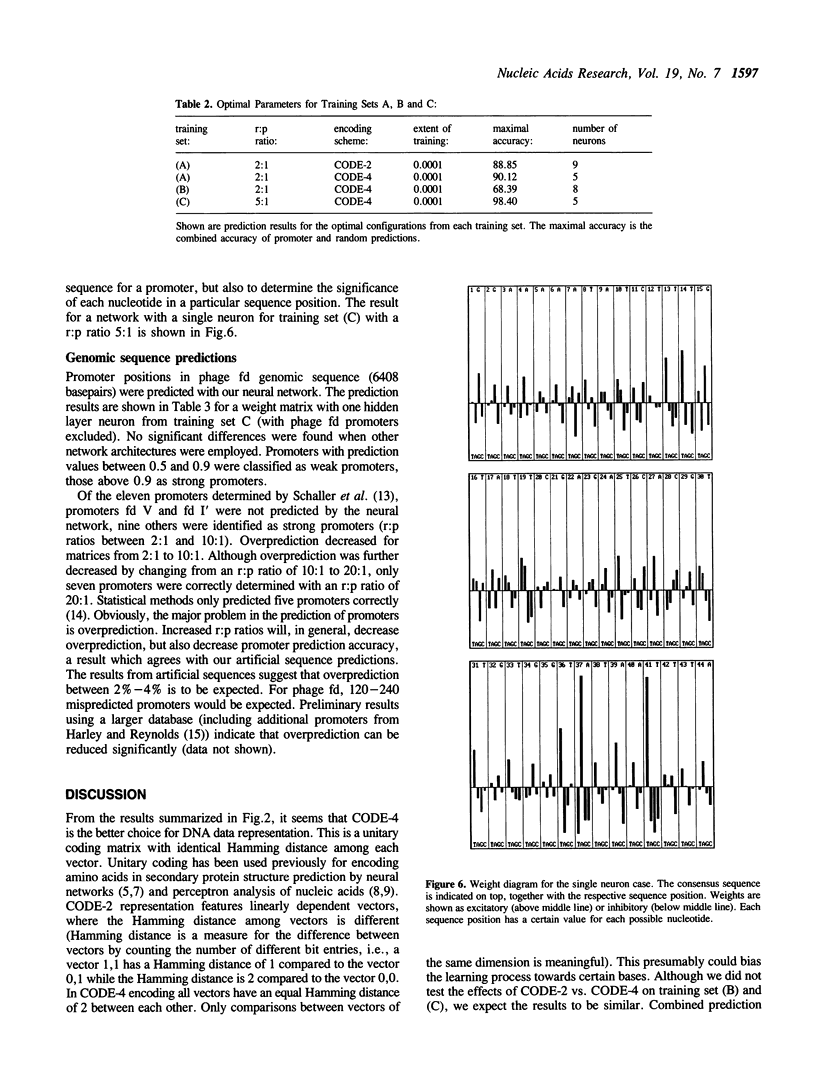
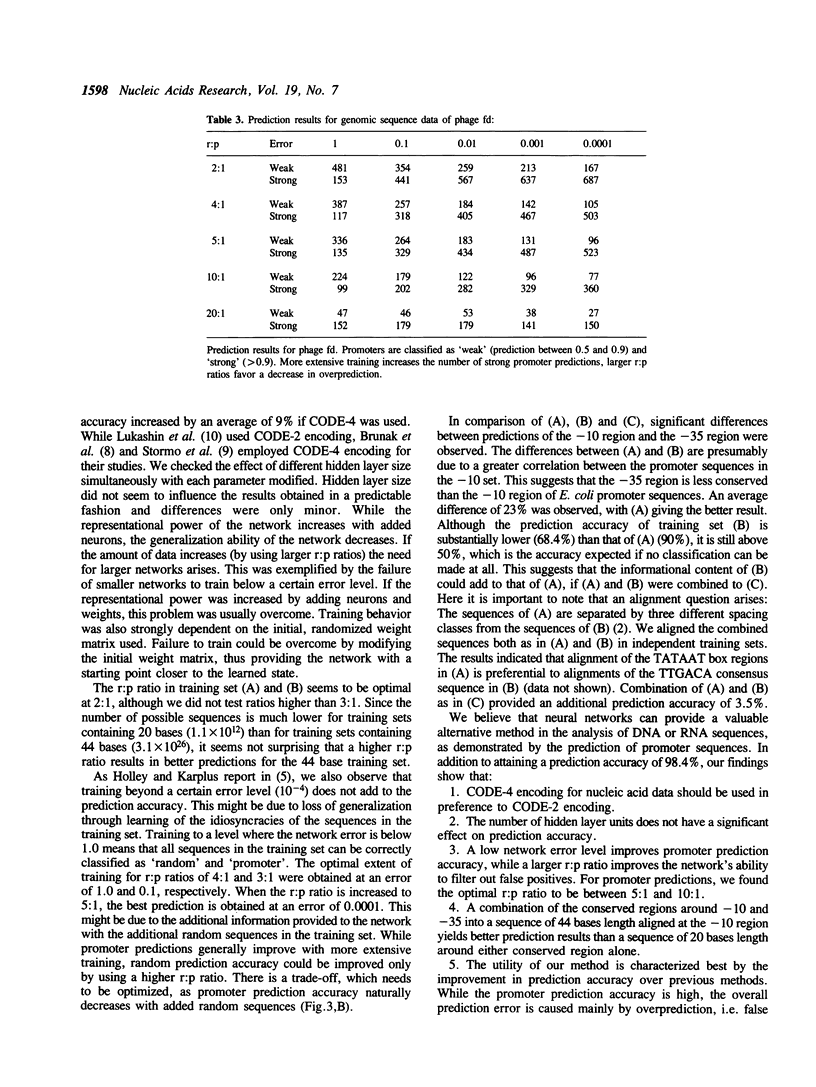
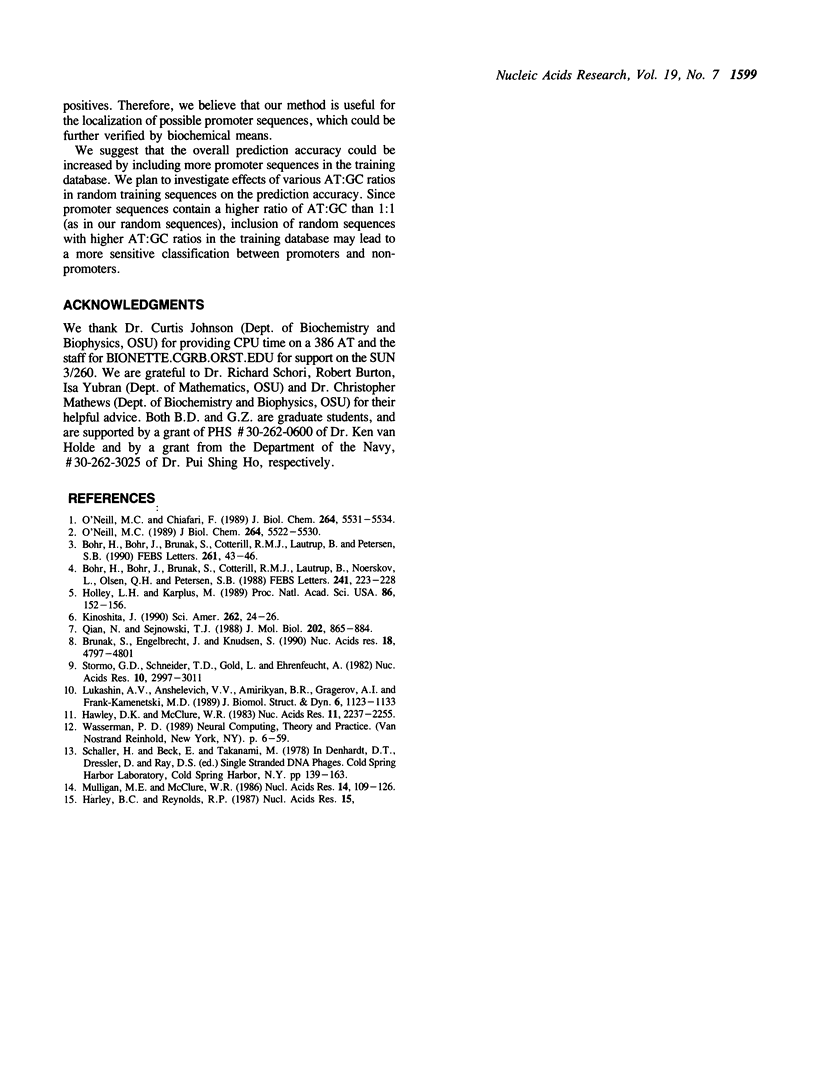
Selected References
These references are in PubMed. This may not be the complete list of references from this article.
- Bohr H., Bohr J., Brunak S., Cotterill R. M., Lautrup B., Nørskov L., Olsen O. H., Petersen S. B. Protein secondary structure and homology by neural networks. The alpha-helices in rhodopsin. FEBS Lett. 1988 Dec 5;241(1-2):223–228. doi: 10.1016/0014-5793(88)81066-4. [DOI] [PubMed] [Google Scholar]
- Brunak S., Engelbrecht J., Knudsen S. Neural network detects errors in the assignment of mRNA splice sites. Nucleic Acids Res. 1990 Aug 25;18(16):4797–4801. doi: 10.1093/nar/18.16.4797. [DOI] [PMC free article] [PubMed] [Google Scholar]
- Hawley D. K., McClure W. R. Compilation and analysis of Escherichia coli promoter DNA sequences. Nucleic Acids Res. 1983 Apr 25;11(8):2237–2255. doi: 10.1093/nar/11.8.2237. [DOI] [PMC free article] [PubMed] [Google Scholar]
- Holley L. H., Karplus M. Protein secondary structure prediction with a neural network. Proc Natl Acad Sci U S A. 1989 Jan;86(1):152–156. doi: 10.1073/pnas.86.1.152. [DOI] [PMC free article] [PubMed] [Google Scholar]
- Kinoshita J. Net result: folded protein. A neural network deciphers the structure of protein. Sci Am. 1990 Apr;262(4):24–26. doi: 10.1038/scientificamerican0490-24b. [DOI] [PubMed] [Google Scholar]
- Lukashin A. V., Anshelevich V. V., Amirikyan B. R., Gragerov A. I., Frank-Kamenetskii M. D. Neural network models for promoter recognition. J Biomol Struct Dyn. 1989 Jun;6(6):1123–1133. doi: 10.1080/07391102.1989.10506540. [DOI] [PubMed] [Google Scholar]
- Mulligan M. E., McClure W. R. Analysis of the occurrence of promoter-sites in DNA. Nucleic Acids Res. 1986 Jan 10;14(1):109–126. doi: 10.1093/nar/14.1.109. [DOI] [PMC free article] [PubMed] [Google Scholar]
- O'Neill M. C., Chiafari F. Escherichia coli promoters. II. A spacing class-dependent promoter search protocol. J Biol Chem. 1989 Apr 5;264(10):5531–5534. [PubMed] [Google Scholar]
- O'Neill M. C. Escherichia coli promoters. I. Consensus as it relates to spacing class, specificity, repeat substructure, and three-dimensional organization. J Biol Chem. 1989 Apr 5;264(10):5522–5530. [PubMed] [Google Scholar]
- Qian N., Sejnowski T. J. Predicting the secondary structure of globular proteins using neural network models. J Mol Biol. 1988 Aug 20;202(4):865–884. doi: 10.1016/0022-2836(88)90564-5. [DOI] [PubMed] [Google Scholar]
- Stormo G. D., Schneider T. D., Gold L., Ehrenfeucht A. Use of the 'Perceptron' algorithm to distinguish translational initiation sites in E. coli. Nucleic Acids Res. 1982 May 11;10(9):2997–3011. doi: 10.1093/nar/10.9.2997. [DOI] [PMC free article] [PubMed] [Google Scholar]