Abstract
We present a general approach to forming structure-activity relationships (SARs). This approach is based on representing chemical structure by atoms and their bond connectivities in combination with the inductive logic programming (ILP) algorithm PROGOL. Existing SAR methods describe chemical structure by using attributes which are general properties of an object. It is not possible to map chemical structure directly to attribute-based descriptions, as such descriptions have no internal organization. A more natural and general way to describe chemical structure is to use a relational description, where the internal construction of the description maps that of the object described. Our atom and bond connectivities representation is a relational description. ILP algorithms can form SARs with relational descriptions. We have tested the relational approach by investigating the SARs of 230 aromatic and heteroaromatic nitro compounds. These compounds had been split previously into two subsets, 188 compounds that were amenable to regression and 42 that were not. For the 188 compounds, a SAR was found that was as accurate as the best statistical or neural network-generated SARs. The PROGOL SAR has the advantages that it did not need the use of any indicator variables handcrafted by an expert, and the generated rules were easily comprehensible. For the 42 compounds, PROGOL formed a SAR that was significantly (P < 0.025) more accurate than linear regression, quadratic regression, and back-propagation. This SAR is based on an automatically generated structural alert for mutagenicity.
Full text
PDF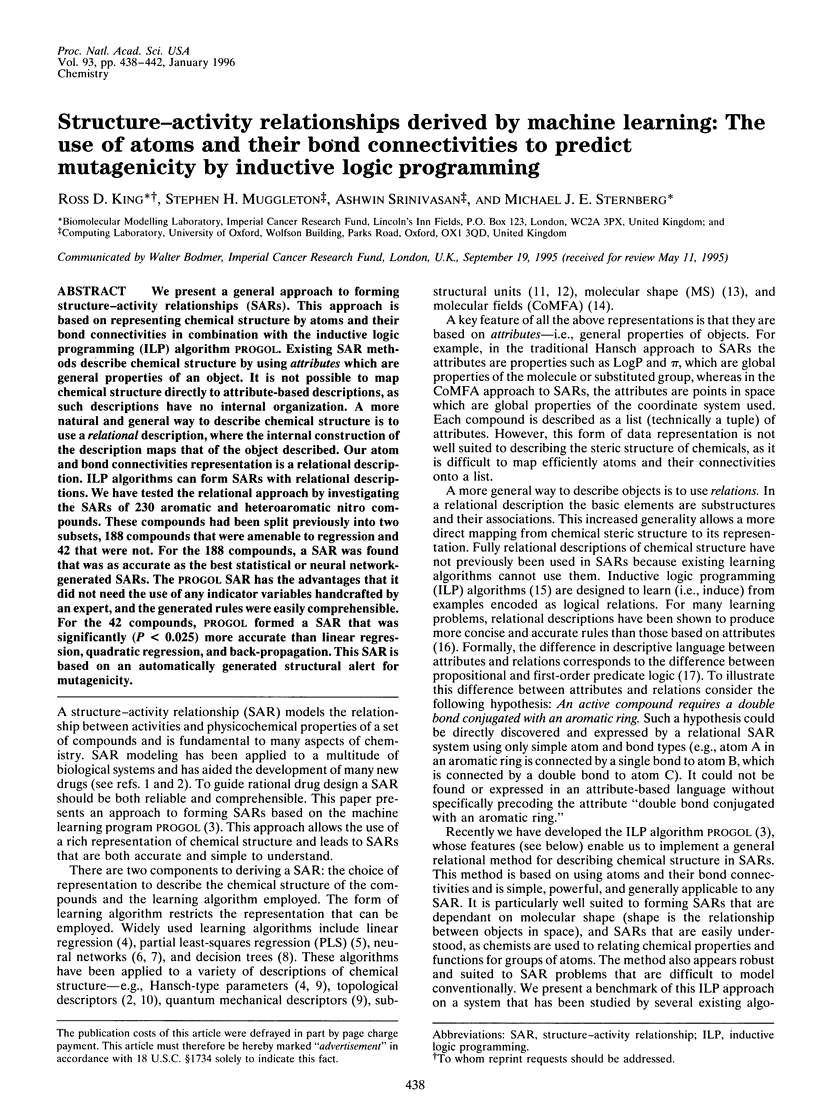
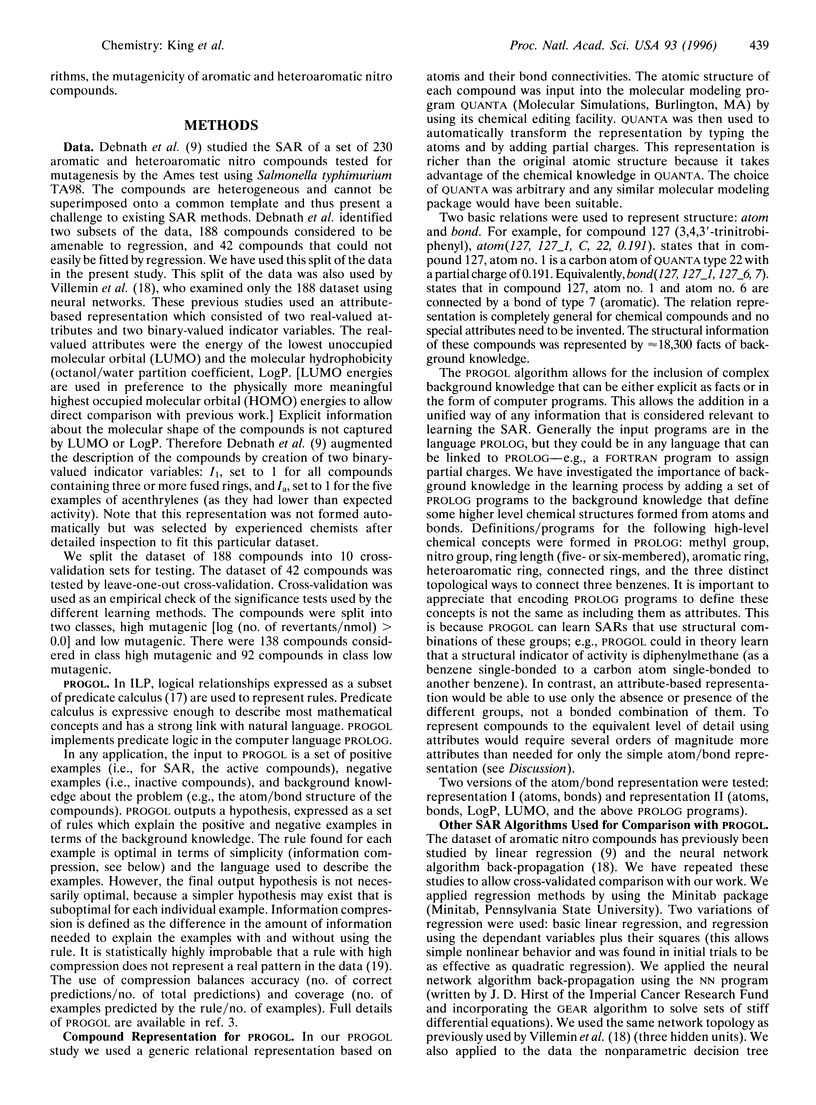
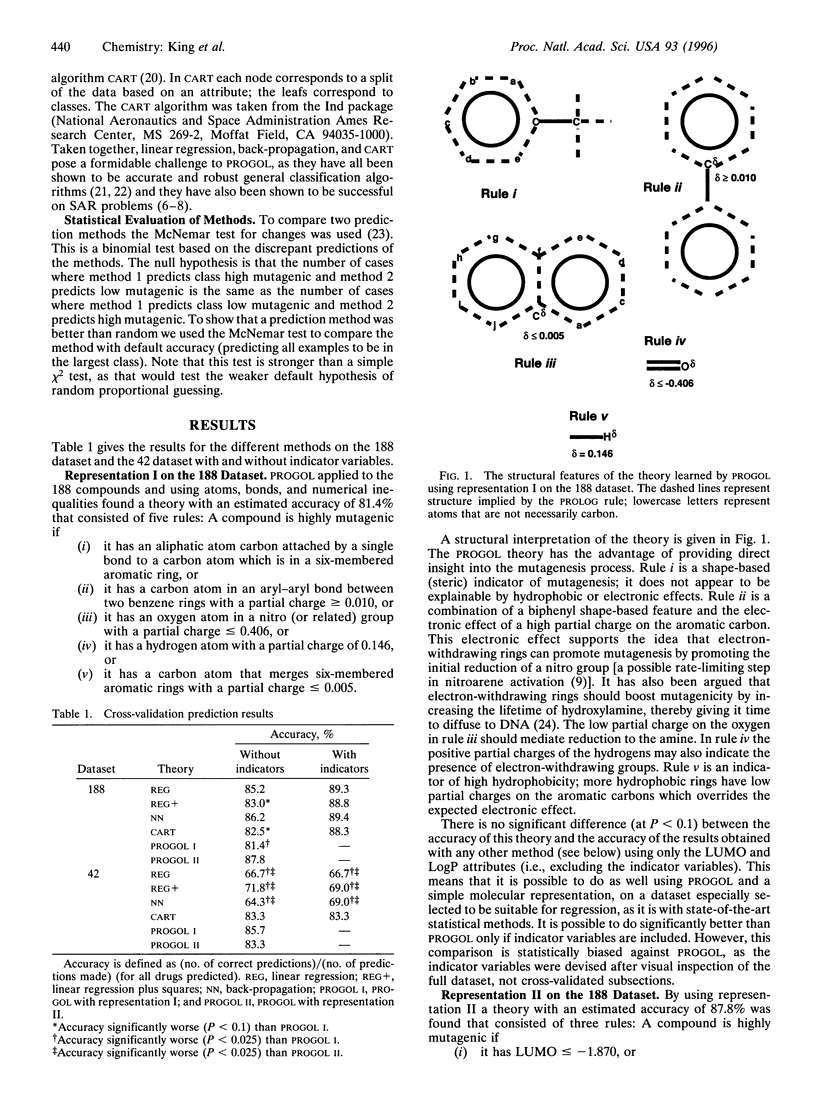
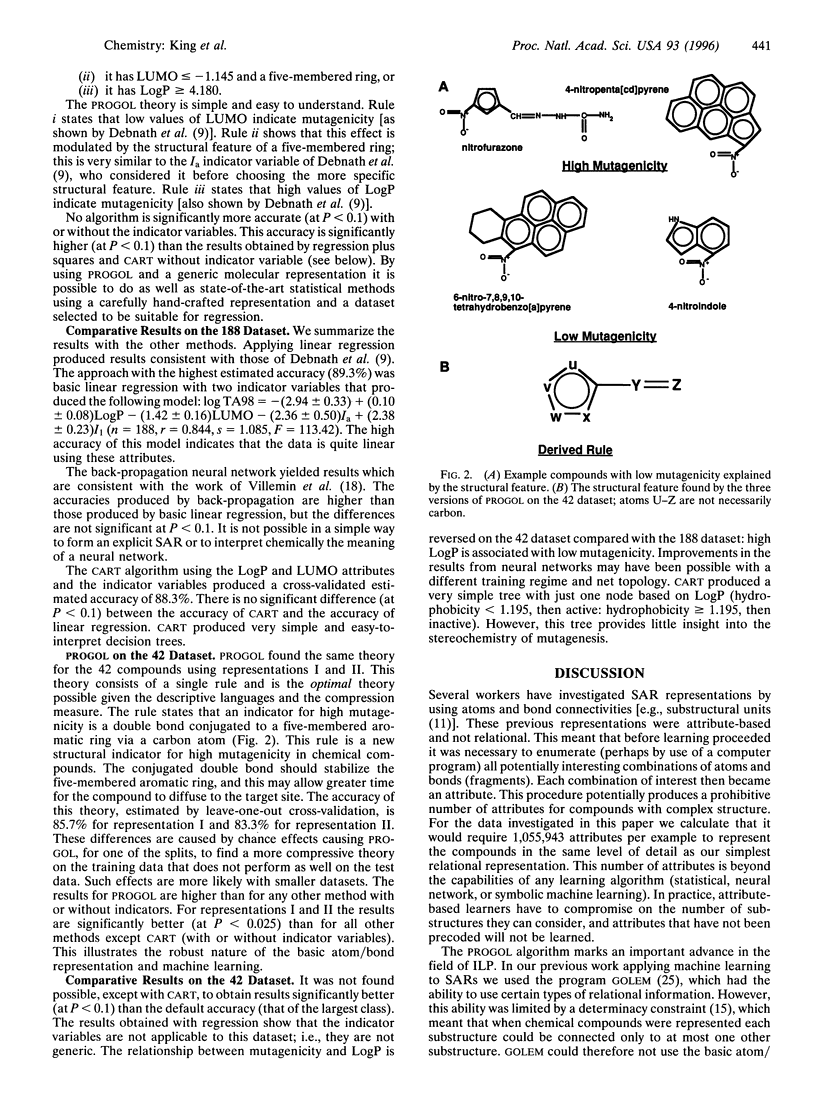
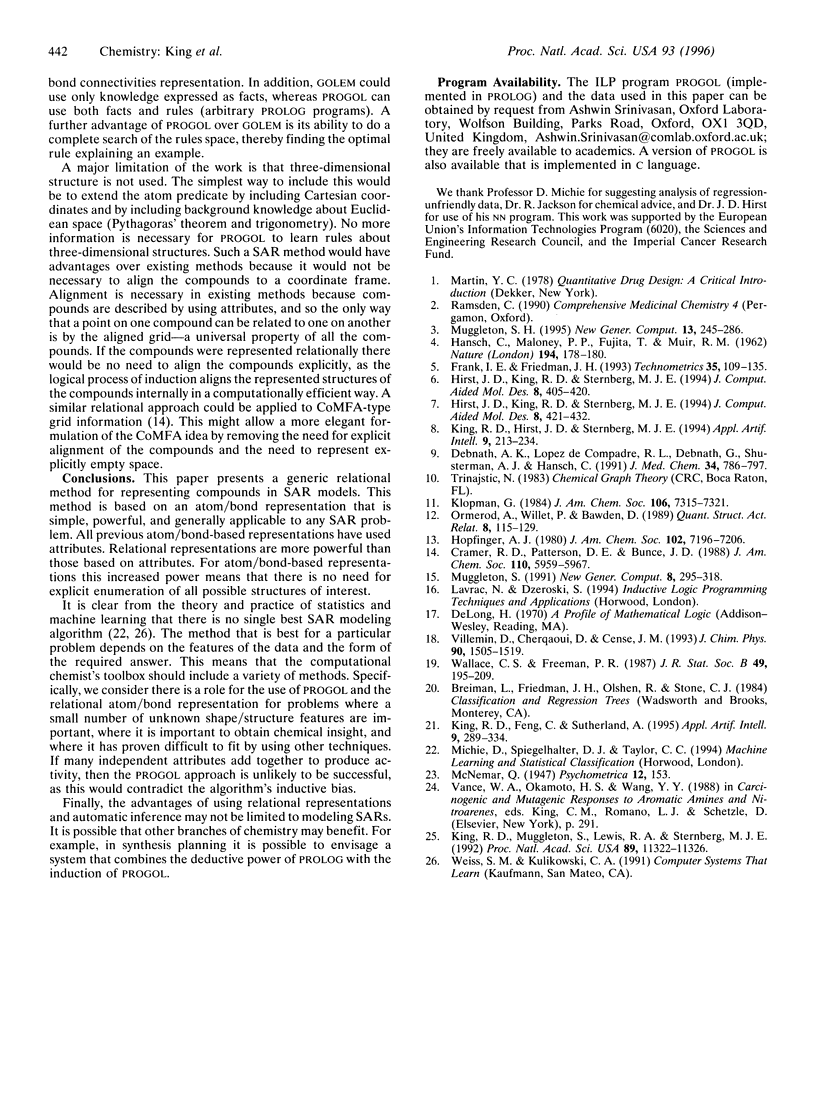
Selected References
These references are in PubMed. This may not be the complete list of references from this article.
- Debnath A. K., Lopez de Compadre R. L., Debnath G., Shusterman A. J., Hansch C. Structure-activity relationship of mutagenic aromatic and heteroaromatic nitro compounds. Correlation with molecular orbital energies and hydrophobicity. J Med Chem. 1991 Feb;34(2):786–797. doi: 10.1021/jm00106a046. [DOI] [PubMed] [Google Scholar]
- Hirst J. D., King R. D., Sternberg M. J. Quantitative structure-activity relationships by neural networks and inductive logic programming. I. The inhibition of dihydrofolate reductase by pyrimidines. J Comput Aided Mol Des. 1994 Aug;8(4):405–420. doi: 10.1007/BF00125375. [DOI] [PubMed] [Google Scholar]
- Hirst J. D., King R. D., Sternberg M. J. Quantitative structure-activity relationships by neural networks and inductive logic programming. II. The inhibition of dihydrofolate reductase by triazines. J Comput Aided Mol Des. 1994 Aug;8(4):421–432. doi: 10.1007/BF00125376. [DOI] [PubMed] [Google Scholar]
- King R. D., Muggleton S., Lewis R. A., Sternberg M. J. Drug design by machine learning: the use of inductive logic programming to model the structure-activity relationships of trimethoprim analogues binding to dihydrofolate reductase. Proc Natl Acad Sci U S A. 1992 Dec 1;89(23):11322–11326. doi: 10.1073/pnas.89.23.11322. [DOI] [PMC free article] [PubMed] [Google Scholar]