Abstract
In intensive care physiological variables of the critical-ly ill are measured and recorded in short time intervals. The existing alarm systems based on fixed thresholds produce a large number of false alarms. Usually the change of a variable over time is more informative than one pathological value at a particular time point. Intelligent alarm systems which detect important changes within a physiological time series are needed for suitable bedside decision support. There are various approaches to modeling time-dependent data and also several methodologies for pattern detection in time series. We compare several methodologies de-signed for online detection of measurement artifacts, level changes, and trends for a proper classification of the patient s state by means of a comparative case-study.
Full text
PDF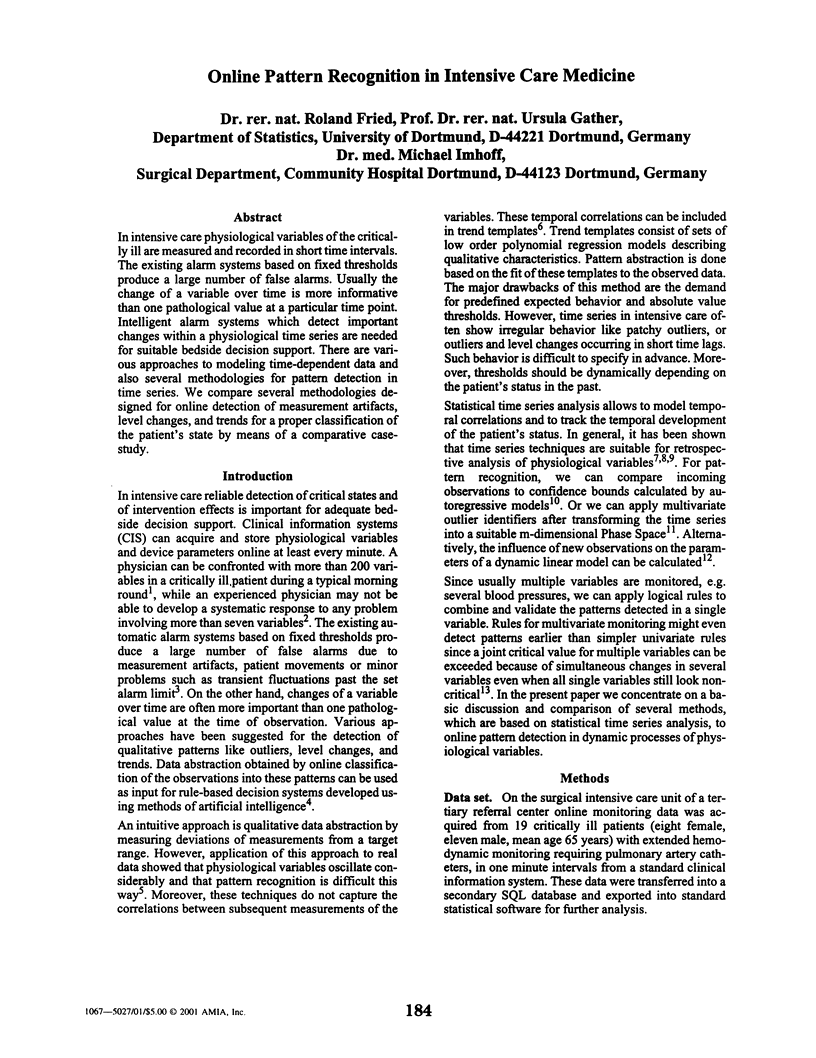
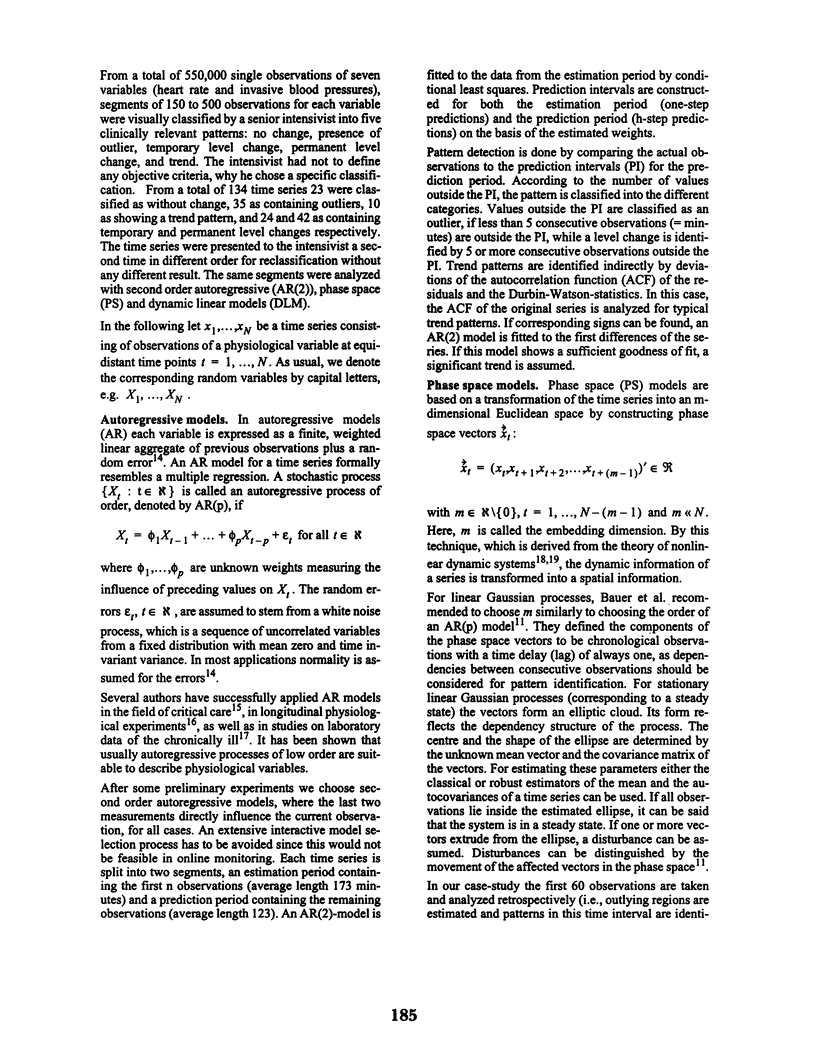
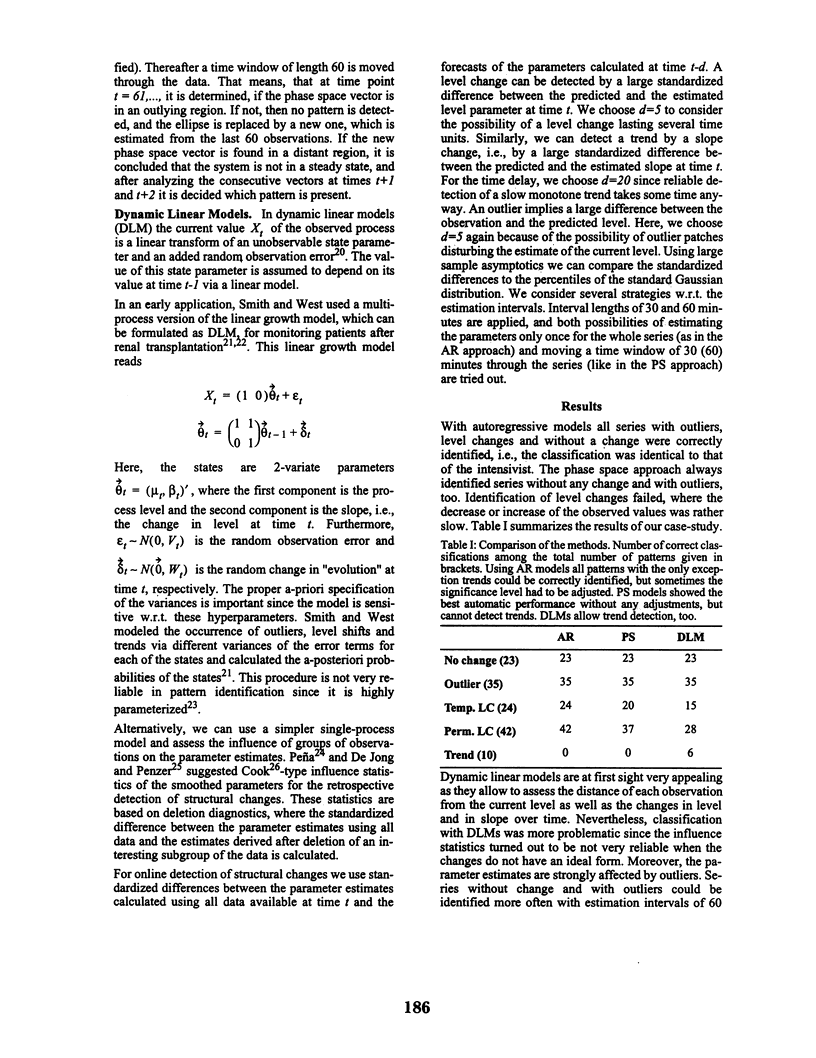
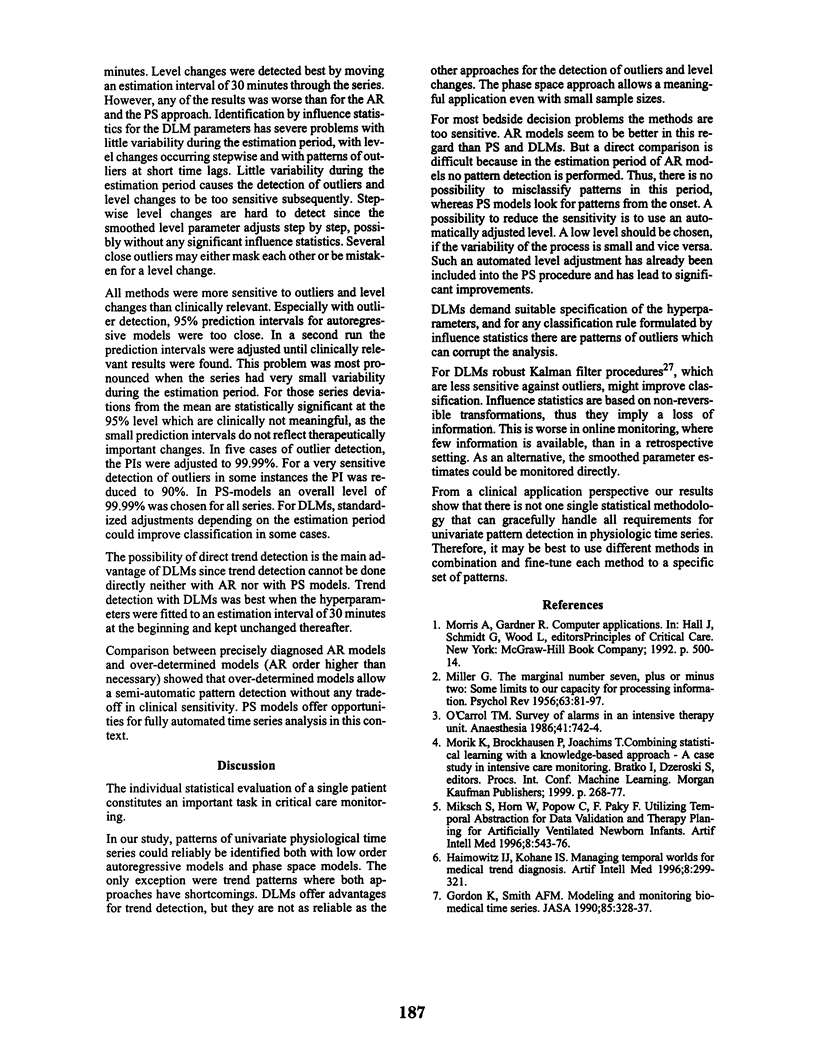
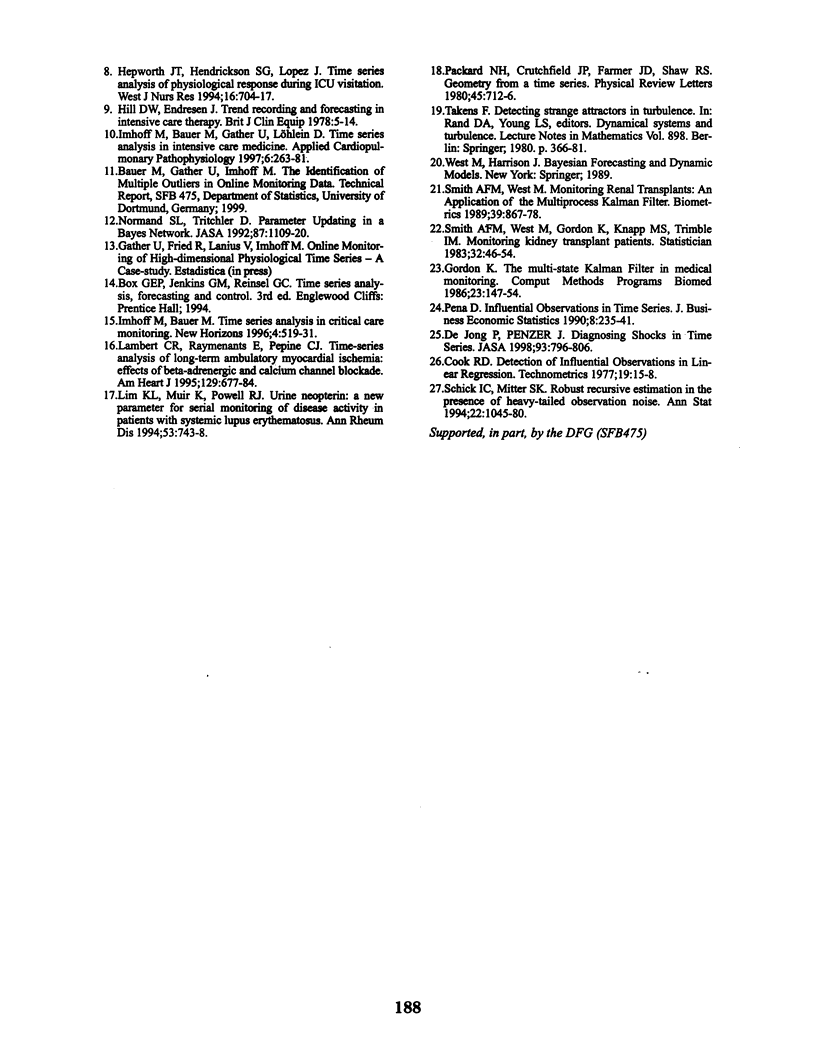
Selected References
These references are in PubMed. This may not be the complete list of references from this article.
- Gordon K. The multi-state Kalman Filter in medical monitoring. Comput Methods Programs Biomed. 1986 Oct;23(2):147–154. doi: 10.1016/0169-2607(86)90109-4. [DOI] [PubMed] [Google Scholar]
- Haimowitz I. J., Kohane I. S. Managing temporal worlds for medical trend diagnosis. Artif Intell Med. 1996 Jul;8(3):299–321. doi: 10.1016/0933-3657(95)00037-2. [DOI] [PubMed] [Google Scholar]
- Hepworth J. T., Hendrickson S. G., Lopez J. Time series analysis of physiological response during ICU visitation. West J Nurs Res. 1994 Dec;16(6):704–717. doi: 10.1177/019394599401600608. [DOI] [PubMed] [Google Scholar]
- Imhoff M., Bauer M. Time series analysis in critical care monitoring. New Horiz. 1996 Nov;4(4):519–531. [PubMed] [Google Scholar]
- Lambert C. R., Raymenants E., Pepine C. J. Time-series analysis of long-term ambulatory myocardial ischemia: effects of beta-adrenergic and calcium channel blockade. Am Heart J. 1995 Apr;129(4):677–684. doi: 10.1016/0002-8703(95)90315-1. [DOI] [PubMed] [Google Scholar]
- Lim K. L., Muir K., Powell R. J. Urine neopterin: a new parameter for serial monitoring of disease activity in patients with systemic lupus erythematosus. Ann Rheum Dis. 1994 Nov;53(11):743–748. doi: 10.1136/ard.53.11.743. [DOI] [PMC free article] [PubMed] [Google Scholar]
- MILLER G. A. The magical number seven plus or minus two: some limits on our capacity for processing information. Psychol Rev. 1956 Mar;63(2):81–97. [PubMed] [Google Scholar]
- Miksch S., Horn W., Popow C., Paky F. Utilizing temporal data abstraction for data validation and therapy planning for artificially ventilated newborn infants. Artif Intell Med. 1996 Nov;8(6):543–576. doi: 10.1016/s0933-3657(96)00355-7. [DOI] [PubMed] [Google Scholar]
- O'Carroll T. M. Survey of alarms in an intensive therapy unit. Anaesthesia. 1986 Jul;41(7):742–744. doi: 10.1111/j.1365-2044.1986.tb12844.x. [DOI] [PubMed] [Google Scholar]
- Smith A. F., West M. Monitoring renal transplants: an application of the multiprocess Kalman filter. Biometrics. 1983 Dec;39(4):867–878. [PubMed] [Google Scholar]