Abstract
AIM--To develop an expert system model for the diagnosis of fine needle aspiration cytology (FNAC) of the breast. METHODS--Knowledge and uncertainty were represented in the form of a Bayesian belief network which permitted the combination of diagnostic evidence in a cumulative manner and provided a final probability for the possible diagnostic outcomes. The network comprised 10 cytological features (evidence nodes), each independently linked to the diagnosis (decision node) by a conditional probability matrix. The system was designed to be interactive in that the cytopathologist entered evidence into the network in the form of likelihood ratios for the outcomes at each evidence node. RESULTS--The efficiency of the network was tested on a series of 40 breast FNAC specimens. The highest diagnostic probability provided by the network agreed with the cytopathologists' diagnosis in 100% of cases for the assessment of discrete, benign, and malignant aspirates. Atypical probably benign cases were given probabilities in favour of a benign diagnosis. Suspicious cases tended to have similar probabilities for both diagnostic outcomes and so, correctly, could not be assigned as benign or malignant. A closer examination of cumulative belief graphs for the diagnostic sequence of each case provided insight into the diagnostic process, and quantitative data which improved the identification of suspicious cases. CONCLUSION--The further development of such a system will have three important roles in breast cytodiagnosis: (1) to aid the cytologist in making a more consistent and objective diagnosis; (2) to provide a teaching tool on breast cytological diagnosis for the non-expert; and (3) it is the first stage in the development of a system capable of automated diagnosis through the use of expert system machine vision.
Full text
PDF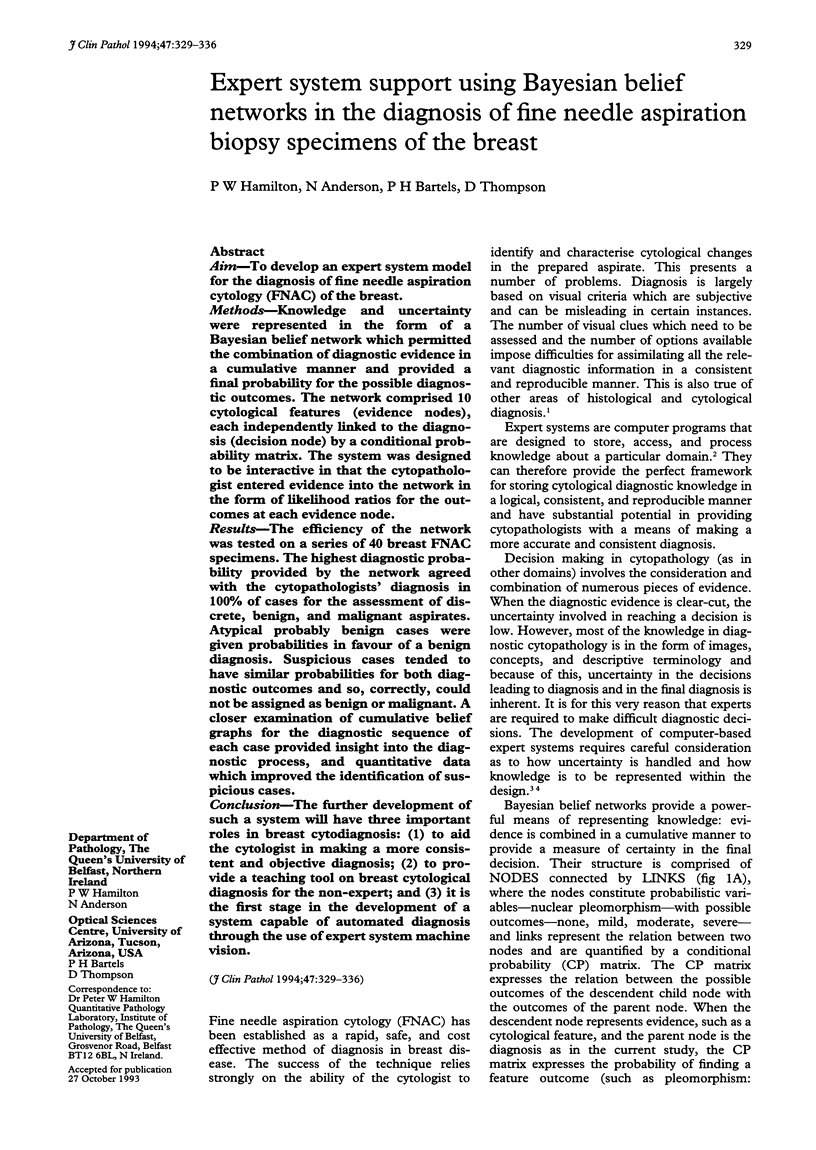
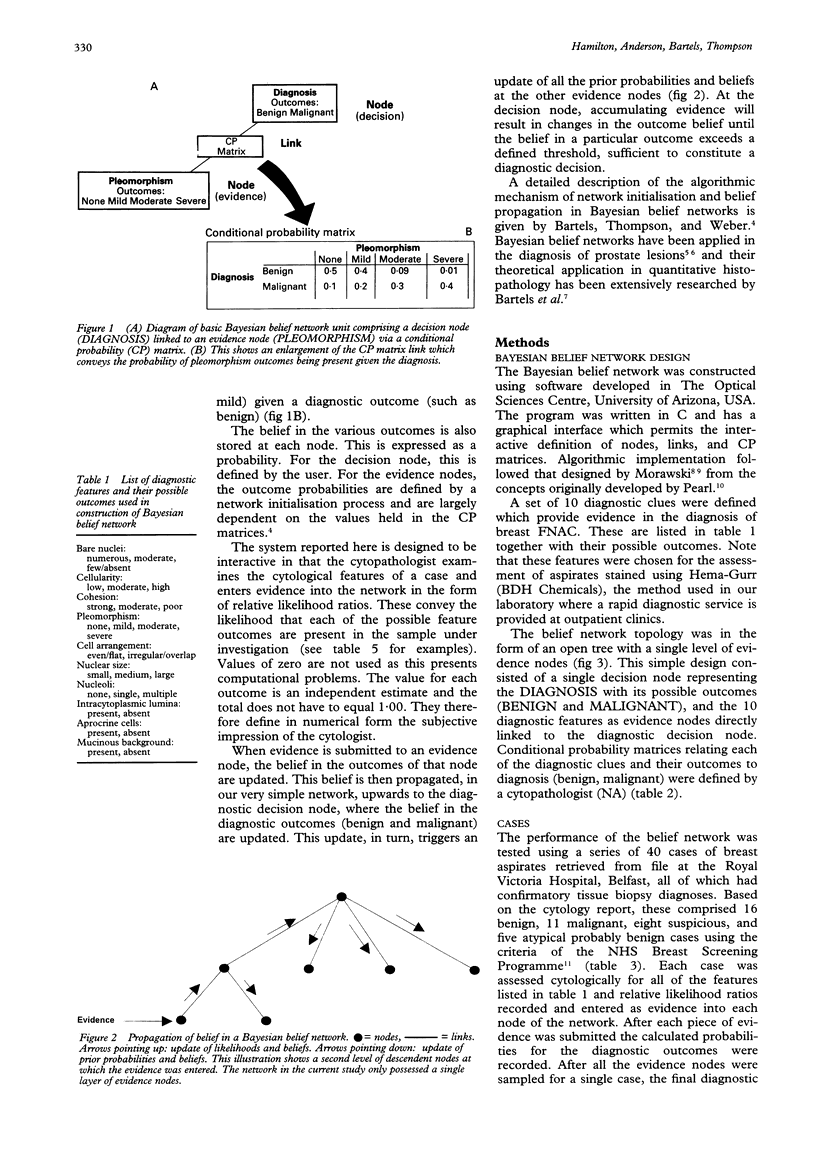
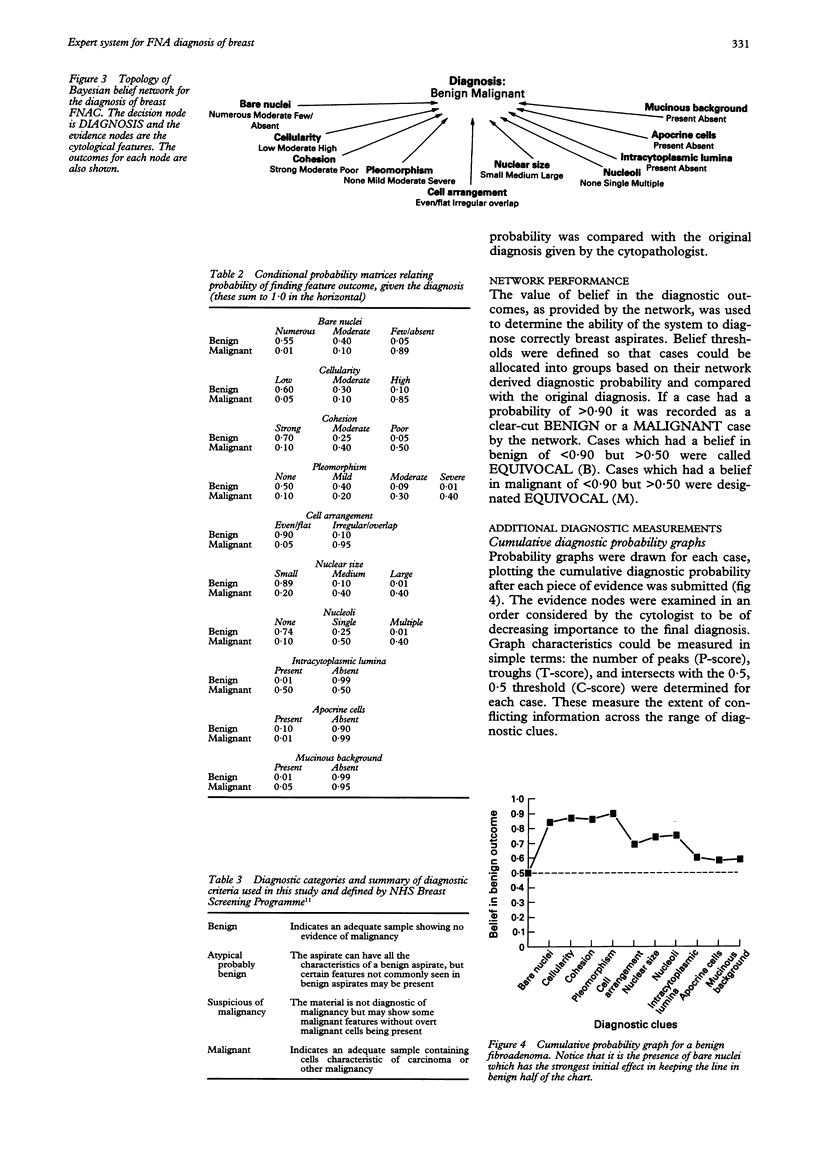
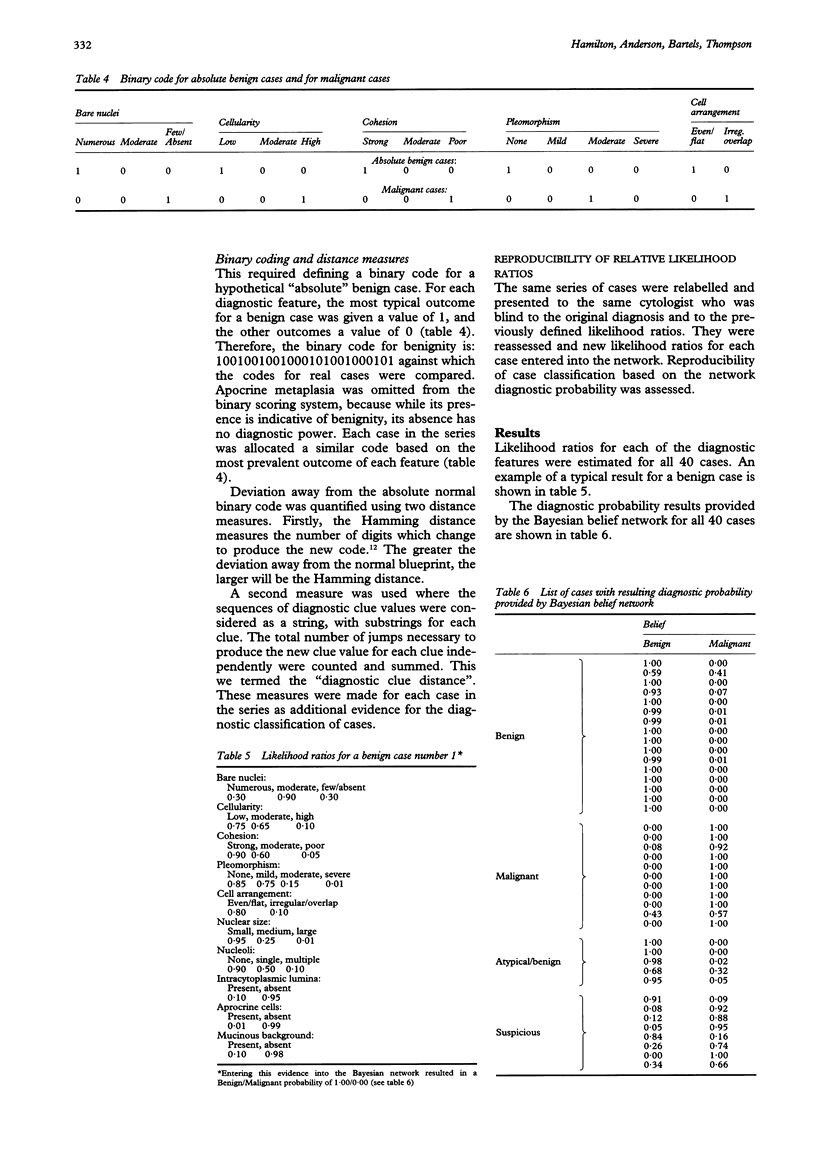
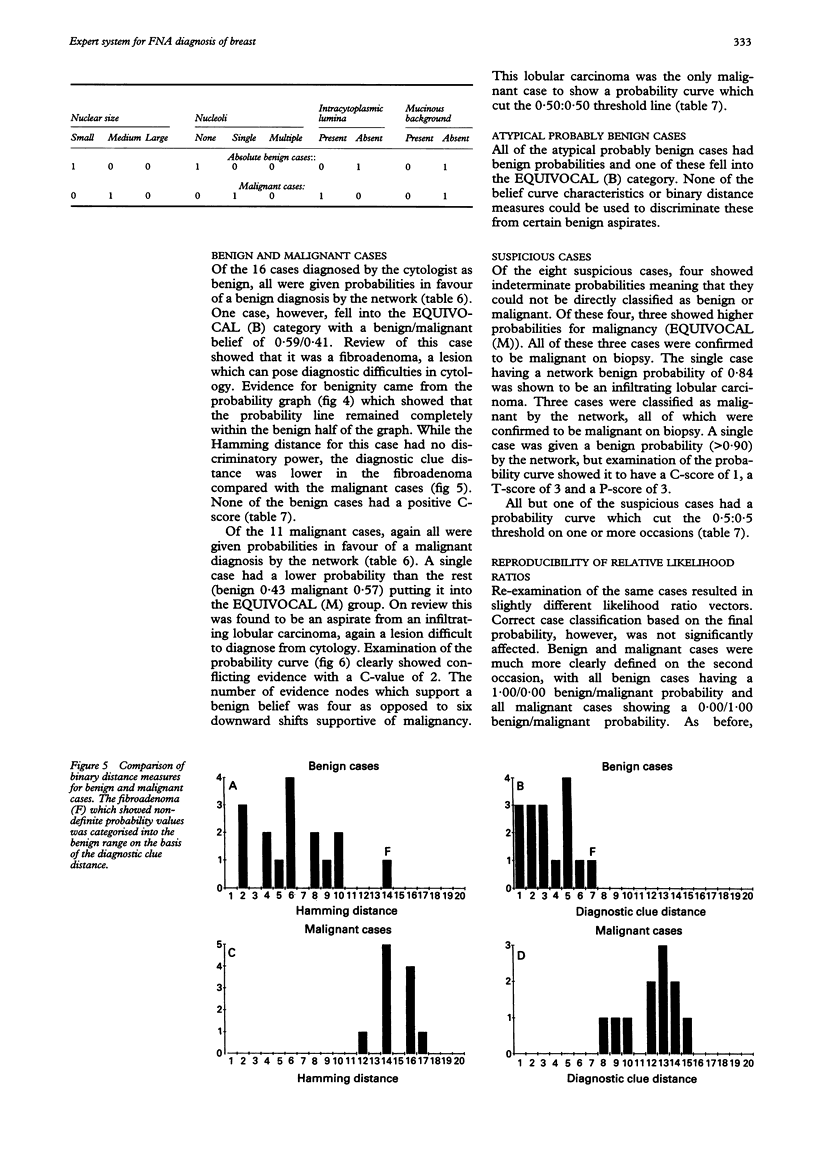
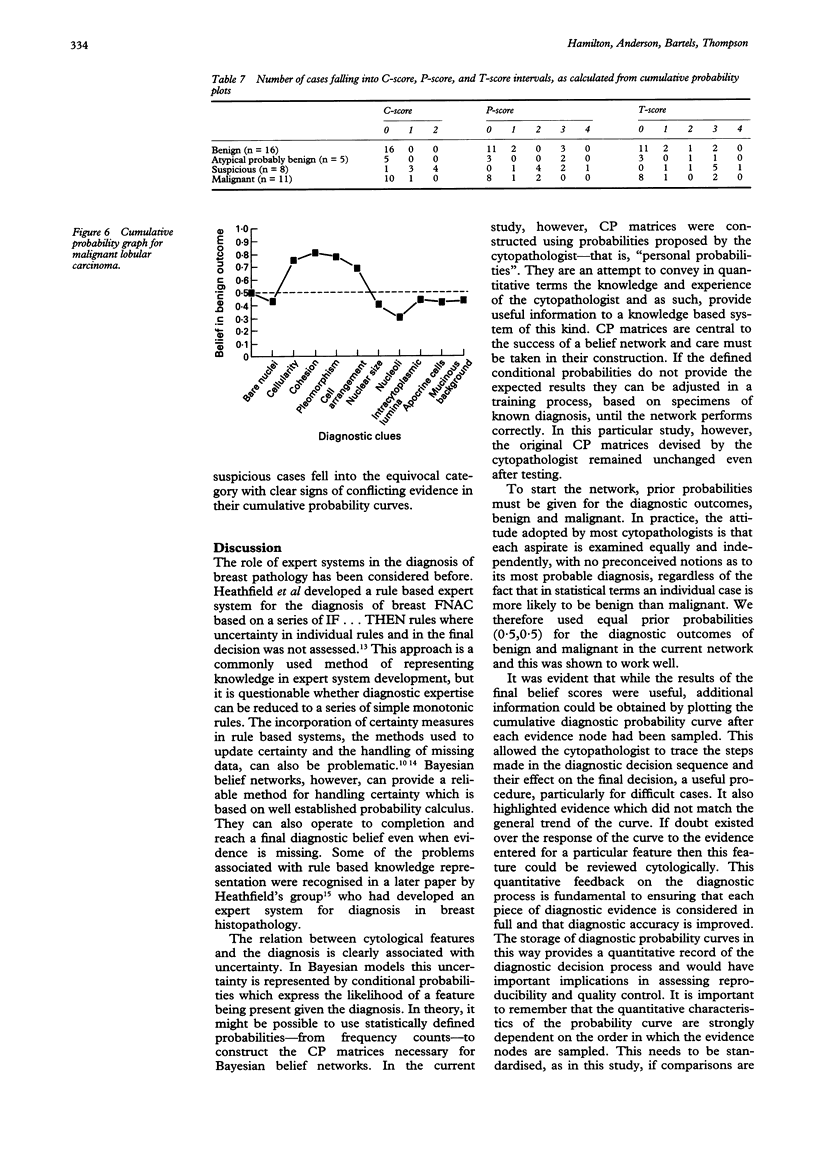
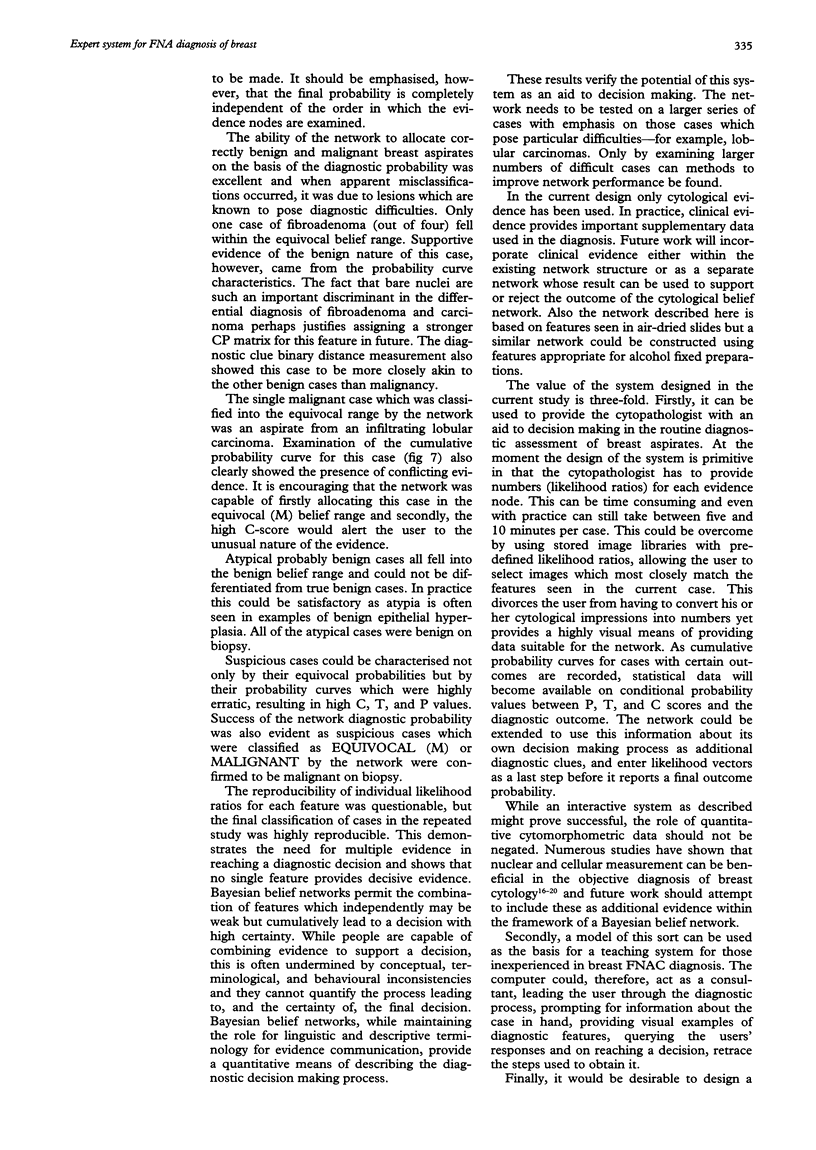
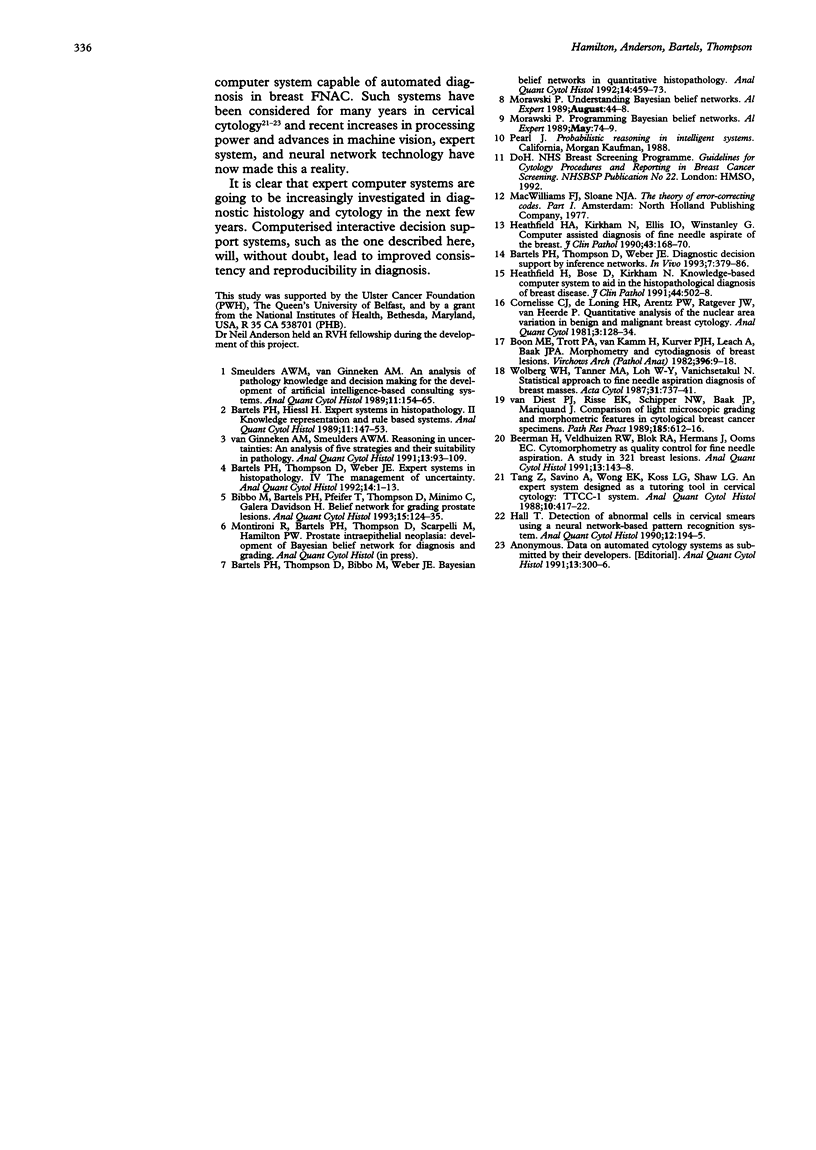
Selected References
These references are in PubMed. This may not be the complete list of references from this article.
- Bartels P. H., Hiessl H. Expert systems in histopathology. II. Knowledge representation and rule-based systems. Anal Quant Cytol Histol. 1989 Jun;11(3):147–153. [PubMed] [Google Scholar]
- Bartels P. H., Thompson D., Bibbo M., Weber J. E. Bayesian belief networks in quantitative histopathology. Anal Quant Cytol Histol. 1992 Dec;14(6):459–473. [PubMed] [Google Scholar]
- Bartels P. H., Thompson D., Weber J. E. Diagnostic decision support by inference networks. In Vivo. 1993 Jul-Aug;7(4):379–385. [PubMed] [Google Scholar]
- Bartels P. H., Thompson D., Weber J. E. Expert systems in histopathology. IV. The management of uncertainty. Anal Quant Cytol Histol. 1992 Feb;14(1):1–13. [PubMed] [Google Scholar]
- Beerman H., Veldhuizen R. W., Blok R. A., Hermans J., Ooms E. C. Cytomorphometry as quality control for fine needle aspiration. A study in 321 breast lesions. Anal Quant Cytol Histol. 1991 Apr;13(2):143–148. [PubMed] [Google Scholar]
- Bibbo M., Bartels P. H., Pfeifer T., Thompson D., Minimo C., Davidson H. G. Belief network for grading prostate lesions. Anal Quant Cytol Histol. 1993 Apr;15(2):124–135. [PubMed] [Google Scholar]
- Boon M. E., Trott P. A., van Kaam H., Kurver P. J., Leach A., Baak J. P. Morphometry and cytodiagnosis of breast lesions. Virchows Arch A Pathol Anat Histol. 1982;396(1):9–18. doi: 10.1007/BF00428497. [DOI] [PubMed] [Google Scholar]
- Cornelisse C. J., de Koning H. R., Arentz P. W., Raatgever J. W., van Heerde P. Quantitative analysis of the nuclear area variation in benign and malignant breast cytology specimens. Anal Quant Cytol. 1981 Jun;3(2):128–134. [PubMed] [Google Scholar]
- Heathfield H. A., Kirkham N., Ellis I. O., Winstanley G. Computer assisted diagnosis of fine needle aspirate of the breast. J Clin Pathol. 1990 Feb;43(2):168–170. doi: 10.1136/jcp.43.2.168. [DOI] [PMC free article] [PubMed] [Google Scholar]
- Heathfield H., Bose D., Kirkham N. Knowledge-based computer system to aid in the histopathological diagnosis of breast disease. J Clin Pathol. 1991 Jun;44(6):502–508. doi: 10.1136/jcp.44.6.502. [DOI] [PMC free article] [PubMed] [Google Scholar]
- Smeulders A. W., van Ginneken A. M. An analysis of pathology knowledge and decision making for the development of artificial intelligence-based consulting systems. Anal Quant Cytol Histol. 1989 Jun;11(3):154–165. [PubMed] [Google Scholar]
- Tang Z. Y., Savino A., Wong E. K., Koss L. G., Shaw L. G. An expert system designed as a tutoring tool in cervical cytology: TTCC-1 system. Anal Quant Cytol Histol. 1988 Dec;10(6):417–422. [PubMed] [Google Scholar]
- Wolberg W. H., Tanner M. A., Loh W. Y., Vanichsetakul N. Statistical approach to fine needle aspiration diagnosis of breast masses. Acta Cytol. 1987 Nov-Dec;31(6):737–741. [PubMed] [Google Scholar]
- van Diest P. J., Risse E. K., Schipper N. W., Baak J. P., Mouriquand J. Comparison of light microscopic grading and morphometric features in cytological breast cancer specimens. Pathol Res Pract. 1989 Nov;185(5):612–616. doi: 10.1016/s0344-0338(89)80204-3. [DOI] [PubMed] [Google Scholar]
- van Ginneken A. M., Smeulders A. W. Reasoning in uncertainties. An analysis of five strategies and their suitability in pathology. Anal Quant Cytol Histol. 1991 Apr;13(2):93–109. [PubMed] [Google Scholar]